Tech Integration Into Pharma: A Report From The Front Line
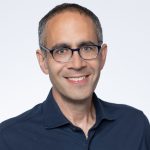
David Shaywitz
Most biopharma companies can talk at some length about embracing data science, and sometimes it’s hard to get beneath the surface of what they’re doing, at least publicly.
But it was my privilege recently to frame and moderate a very insightful recent conversation – recorded for on-demand viewing at this year’s virtual BIO 2020 conference.
This provided an unusual opportunity to get beyond the aspirations and slogans companies tend to use when discussing how to integrate tech and pharma.
For this conversation, we got deep into the sort of issues I tend to encounter in my advisory work, and talked substantively about both the pain points that key players encounter and the specific solutions they are testing as they try to figure out how to make the most of data science in biopharma.
My discussion partners were Ray Deshaies of Amgen, Shahram Ebadollahi of Novartis, Amy Abernethy of the FDA, Chad Robins of Adaptive Biotechnologies, and Peter Lee of Microsoft.
We covered a lot of ground over nearly 90 minutes; rather than summarize, I wanted to share a couple of thematic takeaways that particularly resonated.
Learning From Biological Revolutions In Pharma
It takes a long time for a technology revolution to truly be absorbed by the pharmaceutical industry. Ray Deshaies, SVP of Global Research at Amgen (and before that, a distinguished biologist at Caltech and a HHMI Investigator) described the lengthy process associated with the adoption of biologics (eg antibodies) by an industry that had been built around the discovery, evaluation and development of small molecules.
The revolution clearly took. By 2018, eight of the top ten best-selling drugs in the world were biologics.There’s no doubt these products transformed the industry.
The first monoclonal antibody therapy, Deshaies reminded us, was actually a mouse anti-CD3 antibody, OKT3 (Orthoclone), approved by the FDA in 1986. From there, it took eleven years before the approval of the anti-CD20 antibody rituximab (Rituxan), which would go on to be the first blockbuster drug of this class.
Deshaies reviewed the ongoing evolution of antibody technology, from mouse antibodies to chimeric (part-mouse DNA/part-human protein sequence) antibodies (like rituximab [Rituxan]) to “humanized” (almost all human) antibodies like pembrolizumab (Keytruda) to “fully human” antibodies like secukinumab (Consentyx).
This progression, Deshaies pointed out, reflected a considerable amount of deliberate effort, including extensive investment in successive technology platforms, along with the work required to understand each of them, embellish them, and fully understand the issues with each. In the manufacture of biologics, he noted, there are all sorts of less-appreciated complexities that crop up over time, including issues like the impact of cell line and media, which can each significantly impact the performance of the final protein product, despite a constant DNA sequence encoding it.
The rise of biologics gave rise to profound new needs on the talent side, Deshaies added. It created a need to train a new generation of people; for example, the way you think about pharmacokinetics for biologics is very different than how you think about it for small molecules. Additional learning was required, and it took time for all the newly trained people to get in place.
Deshaies also described a second revolution that also required (ongoing) adaptation: human genetics. This became especially important at Amgen once they resolved to pursue targets validated by human genetics, and acquired Icelandic genetics company deCode in 2012. Most lab researchers, Deshaies explained, were trained in mouse genetics, and need to be retrained in human genetics and human physiology. He reports that while Amgen been able to recruit increasing numbers of scientists trained in statistical genetics and human genetics, there’s still a lot of education required for the organization. “It takes repetition,” he said.
These efforts – at Amgen and across the industry – have persuaded most drug hunters of the value of human genetics; such validation significantly increases a compound’s probability of success. (Of course, a drug may still not work as expected, a tough lesson Amgen itself learned when a genetics-inspired bone drug turned out to cause cardiovascular problems.)
Pursuing human genetics at Amgen, Deshaies added, required more than executive leadership and top-down messaging. While it’s helpful for top brass to say “we want to do human genetics,” he said, it takes so much more for that message and thinking to permeate the organization, and become part of its fabric; considerable time and persistence seem to be required.
Pharma’s experience with these emerging biological technologies seems like useful context as the industry now contemplates the adoption of digital technologies, highlighting the effort, capital, and patience required to effect durable change in the industry. While it’s encouraging that pharma constantly seeks out new technology, it’s sobering to appreciate how long it takes for large legacy organizations to fully internalize new ways of thinking. It’s just not realistic to expect tech adoption to occur overnight in an enterprise this complicated, with this many moving parts.
A Pharma Goes All-In
Novartis is one of those traditional pharma companies that is trying. With deliberate intentionality, and led by CEO Vas Narasimhan since 2018, Novartis is conspicuously trying to take itself through the revolution it sees happening in digital technology.
There has been no shortage of top-down messaging, starting with Narasimhan himself, who essentially introduced himself to the world by offering a vision of Novartis as a data-driven medicines company. Not unexpectedly, as Narasimhan has subsequently reported, progress has been uneven.
Thus it was especially interesting to listen to Novartis’s Global Head of Data Science and AI, Shahram Ebadollahi, discuss his ambitions and approach.
What really struck me was that while Ebadollahi offered an example of “AI exploration”- some sexy longshot project in discovery chemistry – he spent a lot more time discussing “AI empowerment.” He described this effort as an attempt to introduce AI and data science tools (many available through a high-profile collaboration with Microsoft) at every step of the work flow; he wants to distill machine learning into “bite size engines,” so employees don’t even realize they’re using ML – it’s just running in the background. His perspective seemed very aligned with Jim Manzi’s view, using AI to solve unsexy discrete problems.
In addition to the new technology, Ebadollahi described a very deliberate effort to “shift the mindset of people” via constant “multichannel communication.” Basically, if you work at Novartis, it sounds as though you hear about data science relentlessly. There’s an effort to educate current talent through a “data science academy” and other initiatives, and an AI residency program that seeks to embed a recently graduated AI expert within R&D teams.
Successful cultural change in pharma will require an elevation of the practice of data science within the industry, Ebadollahi argued. Today, he said, pharma is driven by biologists and physicians, but if a pharmaceutical organization is really to become a data science company, data scientists have to become truly equal partners.
I imagine most pharma physicians and biologists would reply that this elevation will happen when data scientists demonstrate they can deliver substance and value, not just grandiose promises – which I imagine is why Ebadollahi also highlighted the importance of actually delivering value to programs – of really proving out an approach, not just living in the world of innovation, pilots, and proof-of-concept work. Success, he argued, required putting people with complementary skills in a room and setting them to work.
Learning From Hard-Won Experience
Drawing from her many experiences over her career trying to bring such groups together, Dr. Amy Abernethy, now Principal Deputy Commissioner of the FDA, emphasized not only the importance of integrating health and tech talent, but also the difficulty of pulling it off. The required collaboration is critical but hard, she explained, adding that we need to acknowledge and confront just how hard it is.
Creating a culture that makes all the collaborators equal is a non-trivial challenge, Abernethy said, but also worth it. She argued that the interesting opportunities happen when we pull together streams of information and talent that generally don’t exist in the same place. Like Ebadollahi, she also emphasized the need to move from idea of collaboration to actually getting stuff done.
Abernethy also highlighted the importance of thinking about the application of technology as a “full stack opportunity” – in other words, it’s not only about how tech can help discover a new drug. We also need to think about how it can help accelerate clinical development, and contribute to clinical trials and through the approval and post-marketing phase as well.
Abernethy spoke to the particular importance of not neglecting the “last mile” work – to be sure that the relevant information is delivered to the treating physician in a useful fashion. Doctors operate in an increasingly complex environment, she reminded us, with rapidly evolving knowledge, and limited time with patients who are often (and understandably) distraught. As a physician, you need clarity to offer relevant, useful, data driven advice that technology ought to be able to facilitate.
Abernethy also emphasized that while randomized controlled trials remain the gold standard, the COVID-19 pandemic has highlighted the importance of trying to bring to bear all the data available, and leverage this information to the fullest extent possible, from electronic health record data to claims data to biosensor data. The problem, she explained, is how to bring it all together; everyone thinks there’s a “magic button,” she said, but there’s not, and it’s actually very hard. She hopes that the collaborations driven by the “COVID-19 Evidence Accelerator,” which focuses the community on identifying and then answering a critical set of questions, can help. I hope so too, though my mind kept returning to comments Walmart’s Marcus Osborne made at a recent healthcare conference: the U.S., he said, has no public health infrastructure. That said, if anyone can kluge together something useful from the scattered pieces we have, that person would be Amy Abernethy.
A Startup Integrates Health & Tech From The Outset
The opportunity for emerging tools to potentially add value to established techniques was highlighted by the work of Adaptive Biotechnologies, and their partnership with Microsoft. Adaptive’s focus is sequencing immune receptors – which is probably why their co-founder and CEO, Chad Robins, highlighted the “huge opportunity” in clinical sequencing.
Like me, your first reaction was probably, “Clinical sequencing? How quaint!”
But if we’ve learned anything about revolutions in healthcare, it’s that they take awhile, and often require the confluence of just the right technologies. Adaptive’s thesis is essentially that because the immune systems responds to everything adverse we encounter, it harbors in essence a version of our medical record, documenting the many enemies, both foreign (like a virus) and domestic (like a cancer) we may have encountered and mustered a response against. If this record could be understood, if our history could be “read,” the information could be used to diagnose diseases (like Lyme Disease, celiac disease, cancers, and now infectious diseases like COVID-19), as well as to suggest potentially effective antibodies to be tested as a biologic therapy. For example, they are working with Amgen to prioritize potential therapeutic antibodies against SARS-CoV-2 that Amgen could then develop.
Because of the volume and complexity of the sequencing data they intended to study far exceeded the capabilities of a traditional spreadsheet application, Robins said, they needed to develop their own software tools that could allow researchers to interpret, analyze and visualize the data. This required, from the outset, a culture that necessarily integrated bioinformaticians and biologists.
Role Of Big Tech: Cloud Services Supplier
The Adaptive team also realized that even with their own software, they needed access to ever-more computational power, especially as they set their sights on a particularly ambitious goal – matching specific antigens with specific receptor sequences. This turns out, according to Peter Lee, Microsoft’s Corporate Vice President (and former head of the computer science department at Carnegie Mellon University) a difficult but not impossible machine learning problem, on par with language translation, and a perfect opportunity for a partnership, which is what the two companies announced in 2018, and expanded recently to incorporate work on COVID-19.
In the foundational agreement, Lee explained, Adaptive gained access to the computational power it required to extract remarkable value (if successful) from the immuno-sequencing data the company generates; Adaptive also received embedded Microsoft talent, and an equity investment from Microsoft as well. In exchange, they were compelled to move all their compute to the Microsoft Cloud (and away from competing clouds like Amazon’s AWS and Google Cloud, for example).
Microsoft’s goal, said Lee, is to develop substantial partnerships that drive value through use of cloud-enabled digital technologies. Microsoft sales teams are compensated based not on whether a client buys Microsoft technology (such as access to their Azure cloud), but rather based on the extent to which the client actually uses it. As Microsoft sees it, this places them in strong alignment with customers; the more valuable the cloud is to the business, the more it will be used, and the more revenue Microsoft will receive. (This assumes, of course, that customer use of the cloud services actually correlates with the business value the service generates; there’s a parallel here to fee-for-service medicine.)
Microsoft, in any case, is highly motivated to find ways for the cloud to be useful for customers, as we saw manifest at Novartis as well. (Lee notes the relationship with Novartis is obviously structured very differently than their relationship with Adaptive, which he describes as a model for their relationships with other especially promising startups; however, he notes that even in his relationship with Ebadollahi, Microsoft’s success is “completely indexed” on Novartis’s success.)
Bottom Line
Pharma is highly attuned to technological innovation in a range of domains; the most impactful medicines of today look very different, and were discovered in a very different way, than the medicines of yesterday. Tomorrow’s medicines will look very different than today’s, and are likely to be created, evaluated, and developed in a different fashion as well.
The promise of digital technologies has appropriately captured pharma’s attention, but figuring out how to effectively engage with and implement these emerging approaches remains a work in progress. Powerful computational tools, combined with rich data, offer potentially transformational opportunities in both diagnostics and therapeutics – often in combination with established technologies such as next-generation sequencing and advanced imaging. More immediately, the application of machine learning to the thousands of intricate processes that underlie pharmaceutical operations are likely to provide the earliest indications of impact.
Success of the digital revolution in pharma will require a culture that authentically recognizes and equally values the contributions of data scientists, as well as evidence that the promise of data science is translating into tangible pipeline results. We’ll know we’ve arrived when the impact of data science extends from tools used by patients to stay healthy, prevent disease, and discern early signs of trouble, to the discovery, development, and post-market assessment of new medicines to treat patients when they’re sick, through the provision of timely, point-of-care decision support so providers can most effectively guide patients back to health.
It’s an ambitious vision, at once deeply worthy and highly uncertain. Who’s in?