AI in Healthcare: Harvard’s Zak Kohane on What Innovators Are Missing
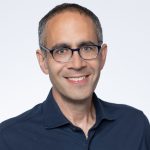
David Shaywitz
Despite, or perhaps because, of the incessant hype, it can be difficult to assess the impact AI is actually having in medicine.
Enter Harvard’s Zak Kohane, who in a remarkably astute recent seminar, available on YouTube, highlighted the opportunities for AI in healthcare while revealing some of the ways AI is falling short – generally by being deployed in a rote fashion that neglects the dynamics of patient care.
Kohane views medicine as “the great frontier,” with “boundless opportunity” – though he emphasizes that “the complexity and breadth of medicine require a multidisciplinary approach.”
Many data scientists, Kohane observes, are enamored of the promise of electronic health data, yet lack an intuitive understanding of the context in which these data were developed. The context is critical, Kohane emphasizes, adding that it’s essential to tease apart at least two very different dynamics captured in medical records data: the physiology of patients and the behavior of doctors.
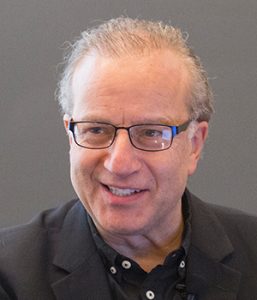
Zak Kohane, Chair of the Department of Biomedical Informatics, Harvard Medical School
Consider the observation that a patient with a normal white blood count at 3 am has a far worse prognosis than a patient with an abnormal white blood count at 3 pm.
A tech guru to whom Kohane presented these data suggested that perhaps this reflected a circadian pattern.
But ask any physician, and the answer is obvious: the only reason anyone would have a blood draw at 3 am would be if something was desperately wrong. In contrast, labs in the afternoon are drawn routinely, and it’s fairly common, incidentally, to see values outside the standard reference range.
Kohane cites a celebrated paper by Google demonstrating the potential of AI to predict hospital readmission rates from EHR data. But hold on for a second, he says. He then shares additional research revealing that nearly all the power from this comes from the AI learning not from the medical values in the EHR records, but rather from the pattern of tests and procedures that were performed.
In other words, the AI is learning from the behavior of physicians as the doctors pursue diagnoses. Kohane shares data demonstrating that when the AI is fed purely procedural information – the “chargemaster” data used for hospital billing – and has no idea what any of these tests and procedures showed, it does nearly as well as the algorithm running off all the actual EHR data, including test results.
Kohane also describes an EHR company’s proprietary algorithm that was developed during the early days of COVID-19, to predict patient deterioration. When it was subsequently tested by an independent investigator on data from a different hospital, the algorithm didn’t perform as well.
The most likely explanation, Kohane says, is also surprisingly common: the algorithm was trained on patients with different characteristics, and involved doctors who might have a different approach to treatment.
It would have been foolish, Kohane says, to rely on such an algorithm, given how rapidly our understanding of the virus changed during the year, including both our approach to treatment and the characteristics of the patients infected. An algorithm developed in the context of one dataset could fail badly if the dataset shifts, especially if the algorithm was used reflexively, and not updated to keep up with the fast-changing circumstances.
Kohane tries more generally to moderate our expectations for AI; for example, he points out that algorithms like the one used to master chess and Go are likely to have only modest applicability to medicine. He quotes a leader in the field, Andrej Karpathy, now at Tesla, who has pointed out that these types of algorithms only work under certain conditions: when the system is deterministic and fully-observed, the action space is discrete, and we have access to a perfect simulator (e.g. the game itself) so that the effects of any action are known.
“Unfortunately,” Kohane observers, “none of this is true of the physiology of disease course, of drug responsiveness, of surgery.”
In fact, Kohane adds ruefully, the only area of medicine where these conditions seem to hold involves reimbursement. He said he expects we will see extensive application of these sorts of algorithms — for the purpose of maximizing billing.
Nevertheless, Kohane is optimistic about the ability of AI to profoundly impact medicine and patient care. One promising application he sees is as an automated note-taker for physicians. That would free doctors up to focus on the patient, while the AI distilled and summarized the doctor’s observations, assessment, and treatment plan.
Identifying relevant disease subtypes is another potential application of AI. One particularly poignant example Kohane noted was the identification of a previously underappreciated subtype of autism with a prominent inflammatory bowel disease (IBD) component.
Savvy analytics helped spot this pattern (which could also help form a hypothesis for further study of what the relation between these two conditions might be). Recognition of the autism/IBD association was especially meaningful to these patients and their families, Kohane explained, because it’s something a physician might otherwise miss – or misdiagnose. Many of these patients are non-verbal, he says, and when they experience gastrointestinal discomfort, their only way to respond is to act out, which is then often treated with tranquilizers – hardly the right therapy for IBD.
Yet now, thanks to data science-enabled research, Kohane says, IBD is more likely to be considered in autistic patients, leading to more appropriate diagnosis and treatment.
Moving forward, Kohane argues, requires us to explicitly recognize and separate health system dynamics and disease physiology; he adds that a third critical component will be patient-gathered data.
Perhaps the most powerful part of the talk occurs after Kohane cites several apparent examples of AI-driven successes. One approach was shown to be helpful in more rapidly weaning premature infants from ventilators. Another algorithm was used to integrate disparate info in a patient’s medical history to suggest a higher probability of domestic abuse.
Yet, Kohane emphasizes, these are not true success stories. Yes, these results were published and shared, but the approaches were never broadly implemented – which he attributes to a failure of leadership, starting with his own.
“We need to recognize our own agency, our own ability to actually change the practice of medicine,” he urges.
Kohane’s other advice for students and young innovators seeking to drive AI through to implementation: “Get an MBA, because business can drive this, and learn about health care policy, because what drives it in the end is money. Having business models and policy models and regulatory models is the way this is going to happen.”
Bottom Line:
The ability of AI-driven approaches to impact the care of patients will depend upon the ability of data scientists to meaningfully incorporate clinical experience and expertise. Determination, leadership — and perhaps a reasonable business plan — are required to ensure that successful AI approaches are not just published in journals but implemented, and find routine expression in the care and treatment of patients.