Computational Techniques Are Driving a Tidal Shift in Therapeutic Protein Design
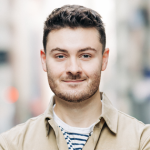
Simon Barnett, research director, Dimension
I recently attended the Molecular Machine Learning (MoML) Conference sponsored by the MIT Jameel Clinic, an institute focused on cutting-edge machine learning (ML) techniques in the life sciences. Computational approaches to drug discovery and development were the centerpiece of the symposium.
Investor sentiment for ML-centric discovery biotechs has been turbulent recently, but researchers at MoML were widely and consistently enthusiastic. Specifically, in silico therapeutic protein design stands apart as an area with enormous, near-term disruptive potential.
Computational techniques may materially compress the costs and timelines associated with therapeutic protein discovery, spawning new business paradigms along the way. How these technologies saturate the pharmaceutical ecosystem to capture value is still nebulous.
Should this thesis play out, adjacent phases of drug development (e.g., clinical translation) will become bottlenecked. We should be grappling with how to solve these challenges today because this future is not as far off as it seems.
Monoclonal Antibody Development is Poised for Disruption
Monoclonal antibodies (mAbs) are a cornerstone of the pharmaceutical industry. Comprising both in vitro and in vivo approaches, the screening technologies scientists use to discover and optimize mAbs have matured over several decades.
Researchers can inject a drug target (e.g., an antigen) into a mouse, rabbit, or other mammal, leveraging these species’ immune systems to produce antibody candidates. Alternatively, scientists can use a method called biopanning. This involves expressing antibody libraries on the surfaces of microorganisms and testing to see which candidates bind to a target antigen.
Both screening paradigms are effective, having produced dozens of approved mAbs. Over the years, both approaches have undergone steady improvements, though I’d argue these have been incremental. At MoML, some even claimed that mAb discovery is effectively solved since very few drug campaigns fail because a high-affinity antibody couldn’t be produced.
I agree—to an extent. Immunization and biopanning are still relatively cost and time-intensive, especially compared to the promise of in silico antibody design. Computational approaches could meaningfully alter the economics of early-stage antibody discovery, enable multi-objective optimization (e.g., affinity and developability) in a manner that chips away at downstream program failures, and contend with the exploding complexity of next-generation biologics (e.g., multi-specific antibodies).
Machine Learning Can Supercharge Protein Design
Drug discovery’s core challenge is traversing between a molecule’s structure (or sequence) and its activity. This relationship is often complex and non-linear. Another hallmark of modern drug discovery is that the design space is orders of magnitude larger than our experimental screening technologies can contend with—creating search bottlenecks.
In silico design is tantalizing because it offers an ostensible bridge between inputs and outputs that isn’t reliant on the throughput of physical laboratories. What if there were a world where researchers could condition ML models to generate a small number of high-quality candidate designs for pennies? This might allow scientists to reconfigure physical assays for the purpose of validation rather than discovery.
This is fiction in 2024, but it may not be in 2030. Progress won’t be uniform, however. My sense is that digital tooling for therapeutic protein design is the most advanced and rapidly improving category.
Proteins have several advantages that other established therapeutic modalities (e.g., small molecules) do not when it comes to the viability of contemporary ML techniques.
Firstly, ML models are only as good as the data they’re trained on. Very large, diverse, well-annotated bodies of data make for the most performant models. These are few and far between in the public domain. Researchers can leverage open repositories like UniProt that are replete with matched protein sequence and function data. Antibody-specific databases like OAS also contain over a billion, highly relevant datapoints.
Small molecules don’t have this advantage. Protein-ligand structural databases, such as the Protein Data Bank (PDB), contain a fraction of the data we have on proteins in the public domain. Though the PDB has given rise to invaluable ML models like the AlphaFold series for structure prediction, I’m convinced that other enabling technologies (e.g., neural network potentials) are required for bridging the structure-activity chasm of small molecules.
Secondly, ML models are attuned to decipher the rich, evolutionary signal embedded in protein sequence data. During training, models extract how natural selection has etched patterns linking sequence motifs with functional attributes. This is how in silico protein models can gain multi-objective capabilities, enabling the simultaneous optimization of affinity as well as other intrinsic properties like aggregation potential, thermostability, and more.
Finally, it’s much easier to physically express and analyze protein sequences than it is for small molecules. Researchers can choose from a host of highly optimized expression chassis and leverage next-generation sequencing (NGS) to map sequence to functional data. This allows labs to establish active learning loops that marry together wet- and dry-lab capabilities.
Where Will Generative Protein Design Be in a Few Years?
Over the next few years, I predict that state-of-the-art computational models will be able to generate 10s of candidate antibody sequences with nanomolar affinity towards specific target epitopes. It’s likely that these models will be multi-objective, enabling co-optimization of multiple, desirable properties. Certain downstream properties with miniscule data (e.g., manufacturing titer) will prove challenging, however.
There’s also a world where specialized or otherwise fine-tuned models excel in adjacent biologics categories, such as multi-specific antibodies, T-cell engagers, minibinders, and more.
What happens in this new, potential reality? It’s true that molecular discovery only represents a fraction of the total cost, time, and failure risk of a drug program. Bookending molecular discovery are target nomination and clinical translation. Both of these are challenging domains that aren’t subject for disruption by even the most sophisticated protein ML models today. Even so, with the rise of potent generative models, several industry aftershocks may occur.
Industry Implications and the Future
Large pharmaceutical companies will seek to maintain their positions. Recently, large pharma has outsourced innovation via M&A of smaller, agile biotechs. This is likely to persist. Genentech’s acquisition of ML-pioneer Prescient Design in Aug. 2021 is an example. I wouldn’t be surprised if most large pharma companies seek to acquire similar, emerging computational platforms.
Established and growing biopharma alike will also outsource biologics R&D to specialized development partners who themselves increasingly lean on computational approaches. San Mateo, Calif.-based BigHat Biosciences and Boston, Mass.-based Generate:Biomedicines are both exceptional protein drug discovery platform companies with burgeoning pipelines.
Other companies will try to transform their discovery engines by purchasing and integrating a wave of ML-native protein design tools from companies like Cradle, Latent Labs, Chai Discovery, and more. The speed of progress is astounding, as evidenced by the launch and open-sourcing of several competitive structure prediction models just 12 months after AlphaFold3’s introduction.
Next-generation antibody development partners may have totally different unit economics. They may have very small physical footprints, and low fixed labor costs, while supporting an equivalent or greater number of campaigns compared to current players. Whether they try to undercut existing vendors or retain the margin to morph into a new type of company is still unclear.
If the cost and time associated with molecular discovery collapses to near-zero, it will place immense pressure on the up and downstream phases of drug development. Do we have enough sound drug targets to prosecute? Do we have the translational infrastructure necessary to deliver these new molecules to patients?
Investing in the entire stack, from target biology to regulatory affairs, is Dimension’s modus operandi (TR coverage, Jan. 2023). While we expect generative protein models to supplant existing discovery techniques, innovative methods will need to saturate the entire ecosystem to achieve tidal shifts in the aggregate burden of bringing new medicines to patients. The potential exists to make drug discovery faster, cheaper, and better. We’re excited about the future and there’s still so much to build.
Simon Barnett is Research Director at Dimension.
Disclosure: Dimension is an investor in Chai Discovery.