AI Can Help with Repeatable Processes, But Don’t Expect Thunderbolts for Drug Discovery
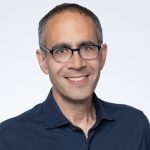
David Shaywitz
Biopharmaceutical and healthcare executives increasingly find themselves attending conferences and presentations featuring the evangelistic selling of AI by self-assured VCs, energetic entrepreneurs, and earnest consultants. The promise is that AI will change everything.
Then the executives return to work, face the quotidian reality of their operation, and wonder whether AI will change anything.
Enter Jim Manzi.
Manzi, depending on your view, is a business operations guy with an affinity for math, or a math whiz who’s taken his talents to business operations. Manzi, as Lisa Suennen and I found out on a recently-recorded Tech Tonics podcast, scheduled to air later this year, enrolled at MIT at the age of 16 because he was bored with high school. Soon, he discovered he loved everything about the place, ultimately pursuing advanced math and physics.
After MIT, he started a prestigious graduate program at Wharton, but then decided against academic life and dropped out after a year. He wanted to get to work, and soon found that he loved thinking his way through practical business problems.
At first, he pursued this through a quantitative management consulting firm. Later he started his own company, called Applied Predictive Technologies (APT), which enabled consumer companies to set up randomized controlled studies to empirically address real-world choices, like how best to position candy on a convenience store shelf. The company was acquired by MasterCard in 2015, for $600 million.
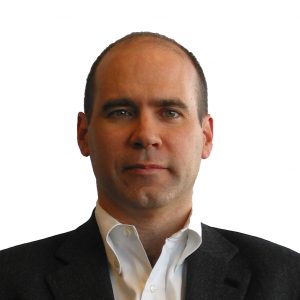
Jim Manzi
Manzi’s experiences at APT led him in 2012 to write a fantastic book, Uncontrolled, about both the utility of such experiments but also about the dangers of overgeneralizing from these sorts of studies to draw unfounded policy conclusions. As he told Lisa and me, “Knowing the confidence interval is as important as knowing the estimate.“
More recently, Manzi has started a new company, Foundry.ai, which essentially seems to offer “AI business solutions as a service.” The company seeks out pressing business problems that could potentially be solved by AI, and designs a fit-for-purpose solution. Projects that gain traction can potentially evolve into stand-alone businesses that might be offered by Foundry.ai to new customers with similar problems. Foundry.ai benefits by working closely with an initial customer to develop and refine a solution to a known high-value business problem, and the original customer benefits from having a pressing business problem effectively solved in a customized fashion.
As Manzi increasingly finds himself meeting with life science and healthcare delivery companies, I thought his perspective on AI might be especially relevant.
For starters, Manzi views AI as “a new name for an old thing” – as well as “the most overhyped term in the history of the world” (the irony of this description isn’t lost on him).
As Manzi sees it, “AI on television may be robots playing Jeopardy” but when “applied in real business settings to improve performance,” AI is basically “data plus math used to create statistical improvement in some repeated business decision process.” He tends to frame problems as “Is there a real opportunity to drive performance improvement?”
Consequently, Manzi tends to be on the lookout for “repeated processes.” This is pretty much the opposite of asking AI to conjure up the creativity required to make a new drug from scratch. But Manzi notes there are “business processes that happen repeatedly within drug discovery, within financial operations of a company, within the execution of randomized trials – solving those sub-problems is actually a much more appropriate use of AI.”
As Manzi puts it, “The idea that AI is going to solve a problem that a group of extremely smart expert people cannot solve is generally a mirage; where that’s happened is in very defined problem spaces like Chess or Go.”
He adds, “When you get into an extremely unstructured problem space, doing something that no human can do, to me is a really heavy lift for an AI system.” What seems more suitable, he suggests, are tasks that “an expert human can do with reasonably good reliability” but with AI you can do “at way lower cost, way faster, and with greater reliability and lower error…. You have a more narrowly defined problem space.”
Manzi worries about the way AI is currently portrayed by some enthusiasts. “When I look at current AI technologies that I’ve seen up close and write code around, I think that there are extravagant promises being made and assertions being made about what it can do. It can do really valuable things – I build companies around it – that are enormously economically important and actually can improve clinical health outcomes.” But these solutions, Manzi says, do not involve wicked problems.
Still, by improving repeatable processes and focusing on important sub-problems that end up consuming considerable amounts of people’s time and energy, you can free up some human ingenuity to work on the more wicked aspects of drug discovery. In this way, AI “may make it feasible to get you to a drug you might not have gotten to otherwise – a lot faster and a lot cheaper.”