Entering JPM20 With a Grounded, Yet Hopeful, View of Health Tech
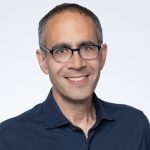
David Shaywitz
Health tech seems balanced precariously between excessive optimism and excessive skepticism, between the promise that emerging technology is poised to disrupt health like it has so many other areas, and the painful recognition that many idealistic technologists misunderstood both the scientific and human dimensions of the inordinately complex problems to be solved in both health care services and the development of novel therapeutics.
It seems like a healthy, motivating tension, provided we can muster both the mental clarity to resist the hype and the intestinal fortitude to outlast the despair.
Technology takes a long time to work through, and figure out how to effectively implement. You can see this in biotech, as I wrote after JPM2018, and discussed recently: today, most leading pharmaceutical companies are aggressively investing in gene therapy and cell therapy, approaches that seemed like fantastical (astounding?) science fiction for years, before a tractable path forward seemed to crystalize before us in just the last decade. Even today, in these areas, we’re still pretty early in the implementation phase; these approaches have demonstrated potential, but generally remain remarkably difficult to execute at scale and successfully commercialize.
What remains clear are the same imperatives that have motivated healthcare innovators for years: the urgent need for profound improvement in the way we practice medicine and discover and develop novel therapeutics.
Clinical Medicine: Crying Out For Improvement
Clinical medicine, as a leading oncologist recently explained to me, remains as much of an art as a science. The aspiration of a learning healthcare system, a perennial talking point, continues to remain an elusive goal; even today, with all our data-gathering and analytic capabilities, so much relevant information is never adequately captured, studied, and fed forward to help the next patient. We need to do a much better job of leveraging the volume of clinical experience to accelerate learning and identify improved approaches to care that could, perhaps incrementally, but in the aggregate, transformatively, improve the care we provide to our patients, which is still largely driven by eminence, intuition, and a litany of cognitive biases.
At the same time, there is also an essential role in medicine for experience and intuition: medicine is the defining example of “fractionated expertise.” For those unfamiliar with the jargon, this is where professionals exhibit demonstrable expertise in some of their activities but not others; I’ve written about this here and here.
The elusive challenge in medicine is figuring how to leverage data without (further) degrading what I continue to believe many patients, especially those with serious and/or chronic conditions, still want (and certainly deserve) from their doctors: an authentic, human relationship, highly attuned to individual emotional subtlety. Such physicians partner with patients in a way that’s responsive to the complexity of their needs – rather than just based on what a coarse algorithm might spit out based on population-level data. The goal is developing the data and the doctors so that we continue to have empathic, inquisitive clinicians with the scientific sophistication to understand the patient’s unique illness, and who are driven to go to the next level, accessing the sort of ready information that can help physicians best tailor treatments to their patients.
Drug Discovery & Development: Crying Out For Improvement
Meanwhile, drug discovery and development seems to be as difficult, and capricious, as ever. Despite the many highly touted advances in biological technology, including the ability to engineer therapeutics with greater intentionality (see here), the failure rate remains staggering. No technology has come along to dramatically improve upon the painful reality that only about one out of 10 drug candidates entering clinical trials (already a steep hurdle) is able to successfully run the gauntlet, and emerge as an approved, commercial product that can be prescribed for patients. Leaders of biomedical R&D teams, appropriately, still regard it as a miracle when a novel drug actually makes it all the way to regulatory approval. Failures at all stages of development continue to abound, challenges I’ve discussed in this space (here).
Every aspect of this process cries out for improvement, from figuring out how to precisely target different conditions at a molecular level to developing suitable candidate molecules and intelligent combinations to precisely matching these candidate therapeutics with the patients most likely to benefit, to identifying these patients and efficiently conducting clinical studies, to, perhaps most importantly, as I discussed in a 2019 Clinical Pharmacology and Therapeutics commentary, understanding how approved drugs are actually functioning in the real world, and learning how to improve and optimize effectiveness.
Given all the concerns about drug prices, there is an urgent need to figure out how to do R&D far more efficiently, or confront the possibility of a devastating slowdown in biomedical innovation if investors decide the rewards from the occasional, rare success no longer justify the high-risk, long-term investment required, and take their dollars to dog food, ad-tech, scooters, or some other less consequential domain.
A definitional question facing pharma companies as they contemplate digital and data science is whether or not to embrace “digital exceptionalism.” This view posits that digital and data approaches are sufficiently distinct that they require a separate locus of expertise. For example, consultancies sending biopharma companies off on a “digital transformation journey” often position the appointment of a chief digital/data R&D officer as an important milestone. Not everyone thinks it should be. As one tech expert with extensive pharma experience recently explained to me, “the world, the science and the market are evolving. If the core technologies are truly quant/technical, the head quant should be the CSO. If not, manage it traditionally via biostats, biomedical informatics and so on,” adding “Digital tools are just tools.” In other words, just as you wouldn’t have a “Chief PCR Officer,” does it make sense to have a chief digital officer, and to consider digital/data as a separate and distinct organizational capability?
On the other hand, you could argue, quite reasonably, that while ultimately digital and data capabilities will be seamlessly integrated, right now, these approaches tend not to be either familiar or intuitive; thus having a core group comfortable with these approaches represents an important and useful temporizing measure.
From “Data Science” to “Science”
Ultimately, as I recently discussed, digital and data science will have the greatest impact when these methods permeate the way biomedical science is done. The good news here is that data science is capturing the interest of undergraduates, and beyond. My middle school daughter – in a California public school – recently devised a small data science-type study for a class science project, receiving support and encouragement from her well-informed teacher. I imagine that as data science becomes inextricably part of more and more scientific domains, learning about it will become as routine as learning about the Krebs cycle and molecular biology, and as familiar to tomorrow’s high school students as squinting at plankton under a microscope or dissecting an unfortunate frog was to students in my generation.
Increasingly, we are likely to think about computation in health the routine way we think about it in other domains. A recent, characteristically fascinating Ben Thompson column discussed how to think about a technology becoming pervasive. He noted that at the turn of the 20th century, there was an explosion of new American car companies: 233 new companies were founded between 1900 and 1909, and an additional 168 in the decade after that. However, the number of new entrants then crashed precipitously, and the big three American automakers in the 1920s – GM, Ford, Chrysler – retained their position of dominance for over 50 years.
Critically, as he points out, “Just because the proliferation of new car companies ground to a halt, though, does not mean that the impact of the car slowed in the slightest: indeed, it was primarily the second half of the century where the true impact of the automobile was felt in everything from the development of suburbs to big box retailers and everything in-between. Cars were the foundation of society’s transformation, but not necessarily car companies.” (emphasis added)
The interesting part is that Thompson (perhaps controversially) argues that “today’s cloud and mobile companies — Amazon, Microsoft, Apple, and Google — may very well be the GM, Ford, and Chrysler of the 21st century. The beginning era of technology, where new challengers were started every year, has come to an end; however, that does not mean the impact of technology is somehow diminished: it in fact means the impact is only getting started.”
He adds that consumer startups take the presence of Microsoft, Apple, Google, and Amazon (MAGA?) as “an assumption, and seek to transform society in ways that were previously impossible when computing was a destination, not a given. That is exactly what happened with the automobile: its existence stopped being interesting in its own right, while the implications of its existence changed everything.”
Perhaps it’s not too much of a stretch to suggest we’re at a similar place in healthcare, where key aspects of the computational infrastructure can now be thought of as a given (even though of course improvements will continue to occur), and rather than wait for some future magic tech to descend from the sky (or Silicon Valley) deus-ex-machina style and magically solve all our healthcare challenges, we need to embrace the imperfect but exceptionally powerful technologies of today and really focus on applying them creatively and pragmatically both to care delivery and to pharmaceutical research and development.
Hopefully, this year’s JPM health tech discussions will focus less on audacious future promises about how technology is poised to disrupt/eat/transform healthcare, and provide concrete examples of how emerging technologies are meaningfully engaging with care providers and drug developers to deliver tangible benefits to real world users.