Randomized Controlled Trials For Healthcare Delivery Work; Now Let’s Do More At Scale
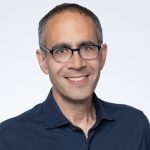
David Shaywitz
The value of randomized controlled trials (RCTs) in healthcare delivery was highlighted earlier this year with the publication in the New England Journal of Medicine (NEJM) of a paper that rigorously evaluated a deeply appealing hypothesis: that you can improve care and reduce costs by focusing on “superutilizers” – the patients who consume the most healthcare resources.
I discussed this paper, and some associated issues, at a recent Harvard Department of Biomedical Informatics (DBMI) faculty journal club, and thought a few highlights might be of particular interest to TR readers.
The story of the trial is captured magnificently by the Tradeoffs podcast – you can listen here, and read a helpful summary here.
The protagonist is a New Jersey physician named Jeffrey Brenner, who became interested in better serving patients in Camden, New Jersey. It’s a city with 74,000 people across from Philadelphia. About 42 percent of the population is African-American, and about 37 percent of the population lives in poverty, according to the US Census Bureau. After reviewing hospital data, Brenner realized that hospital use, and more generally, healthcare costs, weren’t evenly distributed.
In this population, like others, a small percentage of patients (about 5%) accounted for the vast majority of healthcare costs (~ 50%). This group of people has been dubbed the “5/50’s.” Many of these “superutilizers” are patients facing a remarkably complex group of social and economic challenges that can make life, and health, disproportionately difficult for them.
Brenner’s hypothesis was that a key challenge these patients face is engaging effectively with the healthcare system, and by providing them with a team of guides (sort of like sherpas), to help coordinate their care, the patients’ health would improve, and hospital utilization would decline. Initial work seemed to bear this out – healthcare costs and utilization seemed to go down for the first several dozen patients treated in this program.
It all seemed to make a lot of logical sense.
Brenner’s star really began to rise after his efforts were profiled by Atul Gawande in an inspirational New Yorker article, “The Hot Spotters,” in 2011; Brenner received a MacArthur genius award in 2013, and his program was widely hailed as a success, which many sought to emulate.
Yet Brenner, it turns out, encountered skeptics at health conferences. To his exceptional credit, he recognized the need to further test his hypothesis, and really pressure-test his program, through a randomized control study.
This trial was led by an independent, trusted group from MIT, led by noted economist Amy Finkelstein. Brenner himself left to join United Healthcare Group in 2017, attracted, he said, by the opportunity to apply some of his learnings from an even larger platform – that of the world’s largest insurer.
The MIT results, published in the NEJM in January 2020, were disappointing. There was no difference between control and treatment arms in the number of hospital readmissions within 180 days – the primary endpoint. Moreover, both groups showed a decline in utilization, suggesting that the previously-observed decrease seen in the first group of patients may well have represented simply an example of the well-described phenomenon of “regression to the mean.”
There are several relevant lessons we might learn here.
First, the study highlights that even – perhaps especially – when there’s a compelling narrative, it’s critically important to perform the rigorous study to be sure that what you might so desperately want to believe is actually true. There are so many ways we can fool ourselves, and so many potential confounders; RCTs – while not without their own issues, in particular, generalizability – help minimize the effect of bias, which is why they’re appropriately considered the gold standard.
The value of randomized controlled trials (RCTs) is perhaps most acutely felt in situations where the truth feels self-evident, to the point where actually doing a study can strike some as unethical.
Prominent examples from the history of medicine are the use of an anti-arrhythmia drug to reduce sudden cardiac death after heart attacks (the intervention seemed intuitive, yet the CAST study revealed the drug actually made things worse); the routine use of pulmonary artery catheterization in critically ill patients (collecting more data intuitively seemed better, yet RCTs revealed no evidence for improvement; a wag even penned an obituary for the device); and perhaps most famously, the use of hematopoietic stem cell transplant for the treatment of breast cancer (the trial was derided by some as unethical given the assumed benefit, yet the approach was found not to improve survival significantly).
The need for careful study is particularly important, and particularly challenging, in areas characterized by what tech entrepreneur Jim Manzi (Tech Tonics interview here; TR discussion in context of AI here) has called high “causal density.”
This is a term referring to “the number and complexity of potential causes to the outcome of interest.” It’s a factor in biological experiments, of course, and an even greater factor, Manzi argues, in areas of social science. If a vaccine works in one population, he says, he’s reasonably confident it will work in another. But if an educational intervention works in one setting, he’s far less confident it will be generalizable, because of all the factors that could be involved.
Perhaps not surprisingly, when many policy measures are actually evaluated by RCTs, most fail. A study from Arnold Ventures revealed that of “13 instances in which the federal government commissioned large randomized controlled trials to evaluate the effectiveness of entire, Congressionally-authorized federal programs,” 11 essentially failed, one yielded modest/marginal benefit, and only one clearly and repeatedly seemed to work: the Department of Defense’s Guard Youth ChalleNGe, intensive, residential youth development program for high school dropouts.
A frustratingly common observation is that initially promising data often fail to stand up to the test of time. As I discussed in a recent Wall Street Journal review of their book, The Power of Experiments, Harvard Business School professors Michael Luca and Max Bazerman share the story of a thoughtful behavioral intervention developed by University of Pennsylvania faculty Katherine Milkman and Angela Duckworth. While initial results looked promising, the effects soon receded – prompting Duckworth (of Grit fame) to observe, “Behavior changes are really *#$@ing hard!”
Yet all may not be lost.
In 2014, a White House Social and Behavioral Sciences Team (SBST) tried to reduce the over-prescription of addictive medicines (Schedule II controlled substances) by sending out a letter informing these doctors they prescribed far more of these medicines than their peers; this type of approach (as I discussed in the Journal) was demonstrably successful in another context — increasing delinquent tax payments, for example.
Yet here, a RCT evaluating this approach failed to demonstrate an impact on prescriptions.
Instead of giving up, however, the team refined their approach, modifying both the targeting of their letter (now focusing on primary care doctors who were unusually heavy prescribers of quetiapine [Seroquel], specifically) and the language used (in addition to peer comparison, the letter noted the doctor was under review by the Centers for Medicare & Medicaid Services [CMS]), and performed another RCT. This time, it seems, the approach worked well; prescribing was reduced by over 11%, a statistically significant effect that persisted for at least two years.
According to experts like Manzi, success in environments of high causal density require just this sort of iterative approach.
“Run enough tests,” he advises, “and you can find predictive rules that are sufficiently nuanced to be of practical use in the very complex environment of real-world human decision making.”
Testing at this scale, Manzi says, requires “integration with operational data systems and standardization of test design,” approaches that are already adopted by a number of organizations within the business world.
Examples, as I discussed in the Journal, include not just tech giants like Google, Microsoft, and Amazon, but also companies like Nike and State Farm, the insurance company. I’ve also discussed the value of “high velocity incrementalism” to use Harvard Business School Professor Stefan Thomke’s term, in TR, here, and in the context of COVID, here.
Strikingly, “run enough tests” turns out to be the advice of Amy Finkelstein, the MIT economist leading the Camden RCT, as well. In an April 2020 Perspective piece in the NEJM, Finkelstein argued that the key to improving healthcare delivery was conducting more RCTs, noting “the increased availability and use of administrative data have made implementing RCTs easier and less expensive than it once was.”
She noted that improved data systems (versus what were available two decades prior) capturing hospital discharge data enabled the Camden RCT study to be done “at substantially lower cost and effort and with less risk of nonresponsive bias” that would have been possible in an era that relied upon survey data collection.
Finkelstein added that “administrative data also enable use of RCTs for low-cost, rapid testing of repeatedly fine-tuned interventions,” citing a 2019 NEJM study from NYU Langone Health, led by my med school colleague Leora Horwitz, reporting the completion of “ten randomized, rapid-cycle quality-improvement projects in one year.”
We’ve seen the ability of deliberate experimentation at scale to impact website traffic and hone the appeal of political messages to voters.
How exciting to contemplate the integrated — and, I hope, routine — use of this process to improve the delivery of care to patients.