What Pharma Data Scientists Can Learn from SpaceX, Health System Barriers, & the FDA
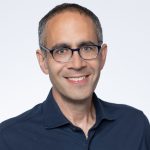
David Shaywitz
Three quick data science items:
1) How pharma companies could engage more constructively with data scientists.
2) How health system barriers to data sharing inhibit robust evaluation of the underlying science.
3) The savvy way the FDA is thinking about data science.
-
Learning From SpaceX
On Wednesday, Elon Musk’s SpaceX landed a prototype spacecraft vertically on the ground — a remarkable engineering accomplishment, and one with important lessons for pharma companies harboring digital aspirations.
SpaceX has been working on this vertical landing for a while. Two previous prototypes crashed to earth with spectacular explosions; even Wednesday’s successful landing was followed in minutes by another large explosion, perhaps because of a “leak in a propellant tank,” the New York Times suggested.
While presumably not pleased by these failures, Musk appeared to see them for what they were, part of the inevitable iterative learning experience that’s required for success with any new technology.
In contrast, many of the data scientists I know in pharma companies feel they have far less room for error. The institutional powers that originally hadn’t welcomed such data scientists at all now have let them in, but often under the equivalent of Dean Wormer’s “Double Secret Probation” (cue up the scene from “Animal House”).
Many data science teams in pharma feel they have a single chance to prove themselves, and if whatever they are trying to do doesn’t work brilliantly, the data scientists worry they’ll be booted, the technology dismissed as not ready for prime time.
This seems precisely the wrong mindset for effective technology implementation. The truth is that it takes time and engagement to figure out any new technology – to learn how to use it effectively in a particular context. The way you do this is by trying something provisionally, seeing what works and what doesn’t, making adjustments, and quickly trying again.
It’s an iterative process that allows for rapid adaptation. This allows far more agility than more classic organizational approaches that insist on the pre-specification of almost everything.
Giving new technology a single shot, and requiring perfection straight out of the gate, sets it up for failure. This sort of rigid thinking ultimately doesn’t allow pharma companies to access the power and benefits that data science and emerging technologies have to offer.
-
Irreproducible
The core premise of science lies in its reproducibility; my description of an experiment should afford you the opportunity to evaluate my math and methods, and to obtain the same results in your own hands.
But this can be a real challenge when the underlying data aren’t shareable – as is often the case with health data studies.
This came up most recently in a departmental journal club I attended, where a guest professor led a captivating discussion of a just-published paper (not hers) that described a particular application of EHR data.
Towards the end of the hour, the focus turned to replication – were the raw data underlying the conclusions in the paper available for review?
Here’s what the text actually stated:
“DATA AVAILABILITY
The data used for this study are available from the [redacted] health system,
but restrictions apply to the availability of these data, which were used under license for the current study, and so are not publicly available. Data are, however, available from the authors upon reasonable request and with the permission of [redacted] Health System.“ (redactions mine).
The way this statement was universally interpreted by 30 or so experienced attendees of this journal club was, as several people actually said, “good luck.”
It was universally understood that no one was ever going to actually access these data.
A couple of points: the first is that health systems typically see no upside in sharing their data. They generally only share the minimum amount possible and only after the maximum amount of duress. Many reasons are often invoked, but a key driver is that there’s no economic incentive for health systems to share, and plenty of reasons not to (including fear of divulging information to competitors). Hence, we are stuck in something of a rut with minimal data sharing.
It’s not just health systems, however. Individual researchers tend not to be especially eager to share their data either.
This isn’t universally true, of course, and important exceptions exist. Some scientists are quite open with their data, and have laudably managed to simultaneously advance both science and their careers – the pioneering work of Daniel MacArthur on the Exome Aggregation Consortium (ExAc) comes to mind.
Nevertheless, many investigators resist sharing “their” data – for reasons that are understandable, if perhaps not always justified. After going through the often arduous process of gathering clinical data, researchers are inclined to guard it so their own team can benefit from this intense upfront effort – a key component of the recent “data parasite” debate. Other times, researchers worry the data will be coarsely reanalyzed, perhaps without adequate understanding of relevant nuance, and legitimate conclusions called into questions by naïve, crusading, attention-seeking critics — a plausible concern.
From the perspective of many researchers, what’s the upside? Many would say: just about none.
This situation evokes the classic academic joke in which a young faculty member is advised to study seven-year locusts – by the time the work can be called into question, the professor will have already achieved tenure!
But to the extent that conclusions drawn from health data can’t be re-evaluated by others because of issues with data access, the science may not be adequately pressure-tested, and flawed methods may remain unchallenged.
This is bad for the discipline of health data science, and ultimately bad for patients.
-
Good News From FDA
Finally, some welcome good news from the FDA, which just released its Data Modernization Action Plan (DMAP) – a companion to the previously released Technology Modernization Action Plan.
I was especially struck (though not surprised, given what DMAP co-author and deputy FDA commissioner Dr. Amy Abernethy has consistently preached) by the emphasis on “high value driver projects.” Dr. Janet Woodcock, acting FDA commissioner, is the other co-author.
As the document states:
“The DMAP is anchored on driver projects that help generate value while building critical capabilities. Driver projects for DMAP are defined as initiatives with measurable value that help multiple stakeholders envision what is possible, allow technical and data experts to identify needed solutions, and develop foundational capabilities. This strategy is distinctly different from focusing on data collection and then looking for questions the data can answer.” (emphasis in original).
In short, this approach gets right what so many get wrong – the importance of collecting and evaluating data with a specific purpose in mind. The intended purpose strongly influences what data are needed and the degree of subsequent validation and refinement required.
This mindset is likely to be far more productive than approaches that robotically dump all data into some kind of lake and then proudly reports how big the lake is.
The DMAP approach is so much savvier, and far more likely to yield meaningful results. This methodology also inherently refines the mechanism of fit-for-purpose data collection; as Dr. Abernethy has stated on a number of occasions, you don’t truly understand a dataset, including its limitations, until you really start to use it.
If FDA starts with these well-defined driver projects, and has success, then there will be more natural momentum to spread the successful practices across the agency.
The pragmatic driver project approach (versus creating a data lake upfront and hoping to figure out how to capture value later) is one that many pharma companies and healthcare organizations would do well to emulate.