AI: If Not Now, When? No, Really — When?
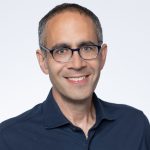
David Shaywitz
“It was all mixed into one, enormous, overflowing stew of very real technological advances, unfounded hype, wild predictions, and concerns for the future. ‘Artificial intelligence’ was the term that described it all.” – Cade Metz, Genius Makers
The buzzy excitement around artificial intelligence (AI), and most recently generative artificial intelligence (genAI), has inspired some biopharma leaders, exasperated many others, and touched almost everyone.
Leading management consulting firms have sold an enormous amount of business by persuading biopharma companies that:
- They are already lagging dangerously behind their competitors on AI adoption;
- There are tremendous productivity gains to be found, and value to be created, in the expeditious adoption of AI.
Within biopharma R&D departments, most researchers remain predictably skeptical of the incessant hype, even as many are authentically curious about promising advances (like AlphaFold, whose inventors received the 2023 Lasker Award for Basic Medical Research). They are also politically astute enough to genuflect to senior management’s imperative to demonstrate the organization’s embrace of AI.
One result has been an AI version of innovation theater, where there’s all sorts of demonstration projects, working groups, PowerPoint decks, partnerships, and celebratory speechifying. A huge amount of heat is generated, but so far, relatively little light.
At one level, none of this is surprising. As I discussed in 2019 in the context of precision medicine, and more expansively in 2023 in the context of AI, it historically takes a very long time for us to figure out how to productively use new technologies. As economic historians like Paul David, Carlota Perez, James Bessen, Robert Gordon, and others have consistently reminded us (as I discussed here), we don’t tend to wring productivity of out new technologies overnight; more typically, it takes decades, and many rounds of successive incremental innovations.
Yet, it’s easy to imagine, in the context of breathless pitches and extravagant promises, that perhaps this time it’s different – perhaps AI has found a way to beat the historical odds, and is leading to the sort of immediate, measurable productivity gains that enthusiasts promise and biopharma executives desperately seek.
For biopharma in particular, the excitement is understandable. As visionaries like Mustafa Suleyman argues in The Coming Wave (my 2023 WSJ review here) and Jamie Metzl argues in Superconvergence (my just-published WSJ review here), the thesis that accelerating revolutions in biotech and AI are compounding each other, and leading us towards a promising, tech+bio future is not just compelling but directionally correct. The question, of course, is when will we realize this AI-infused bio-rapture?
According to a recent, arresting Goldman Sachs (GS) report, entitled “GenAI: Too Much Spend, Too Little Benefit?” perhaps we shouldn’t hold our breath.
The GS report is worth reading in its entirety, but I’ll focus on several salient sections: an interview with the distinguished scholar and MIT economist Daron Acemoglu; an interview with GS’s Global Head of Equity Research Jim Covello, and an interview with two GS Senior Equity Research Analysts, Kash Rangan and Eric Sheridan.
Before we get to these details, it’s worth noting how refreshing it is to read a corporate document that conveys multiple, at times conflicting viewpoints around complex issues like the future state and anticipated economic impact of AI. While top management consulting firms typically offer a singular, consensus view on topics like the path forward for AI, this report from GS acknowledges and systematically explores differences in perspectives and assumptions. The result is an unusually substantive and credible report that conveys nuance and embraces uncertainty.
Daron Acemoglu: Hopeful Skeptic
Daron Acemoglu is a distinguished economist at MIT and the co-author, most recently of Power and Progress. A WSJ review by Deirdre McCloskey described Acemoglu as “a shoo-in for Nobel Prize” in economics, and said the book expressed the authors’ view that “The invisible hand of human creativity and innovation…requires the wise guidance of the state.”
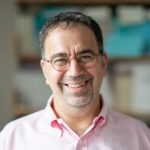
Daron Acemoglu, Institute Professor, MIT
In his conversation with GS, Acemoglu expressed excitement about the promise of genAI, noting it “has the potential to fundamentally change the process of scientific discovery, research and development, innovation, new product and material testing, etc. as well as create new products and platforms.”
However, he cautioned, “these truly transformative changes won’t happen quickly and few—if any—will likely occur within the next 10 years.”
Instead, he suggests, “AI technology will instead primarily increase the efficiency of existing production processes by automating certain tasks or by making workers who perform these tasks more productive.”
He also thinks AI, even with more data and fancier chips, will still struggle with open-ended tasks, like improving “a customer service representative’s ability to help a customer troubleshoot problems with their video service.”
In addition, like many others, Acemoglu worries “where more high-quality data [to power future AI models] will come from and whether it will be easily and cheaply available to AI models.”
He recognizes the future possibilities of genAI, he says, and hopes AI creates new tasks, products, business occupations, competences – but adds this is “not guaranteed.”
While emphasizing that “Every human invention should be celebrated, and generative AI is a true human invention,” he is concerned that “too much optimism and hype may lead to the premature use of technologies that are not yet ready for prime time.”
Jim Covello: Pessimistic Skeptic
As Jim Covello, the Head of Global Equity Research at GS sees it, one critical concern around AI relates to the “substantial cost to develop and run” the technology; the investment only makes sense if AI can “solve extremely complex and important problems for enterprises.” But solving complex problems, he says, is something the technology “isn’t designed to do.”
Covello challenges several familiar assertions used to justify current costs.
“Many people attempt to compare AI today to the early days of the internet,” he says. “But even in its infancy, the internet was a low-cost technology solution that enabled e-commerce to replace costly incumbent solutions.”
In contrast, he argues, AI technology is starting out “exceptionally expensive.”
He adds that the idea that technology “typically starts out expensive before becoming cheaper is revisionist history.” E-commerce, he asserts, “was cheaper from day 1.”
He also says we can’t count on AI prices declining significantly; the dramatic historical decrease in the price of semiconductor chips, he says, was due to fierce competition; but at least today, “Nvidia is the only company cable of producing the [computer chips] that power AI.” Consequently, Nvidia is likely to maintain pricing power in the near-term.
Covello is also skeptical about the transformative potential of AI, arguing “people generally substantially overestimate what the technology is capable of today.” He adds, “I struggle to believe that the technology will ever achieve the cognitive reasoning required to substantially augment or replace human interactions.”
He also contends, “Humans add the most value to complex tasks by identifying and understanding outliers and nuance in a way that it is difficult to imagine a model trained on historical data would ever be able to do.”
Covello points out that he was a semiconductor analyst when smartphones arrived and followed the evolution of smartphone functionality closely. As he remembers it, the ensuing roadmap was clear, “with much of it playing out just as the industry had expected.”
In contrast, he argues, “No comparable roadmap exists today. AI bulls seem to just trust that use cases will proliferate as the technology evolves. But 18 months after the introduction of generative AI to the world, not one truly transformative—let alone cost-effective—application has been found.”
Of particular relevance for biopharma, he notes that “companies outside of the tech sector … face intense investor pressure to pursue AI strategies even though these strategies have yet to yield results. Some investors have accepted that it may take time for these strategies to pay off, but others aren’t buying that argument.”
He warns that “The more time that passes without significant AI applications, the more challenging the AI story will become. And my guess is that if important use cases don’t start to become more apparent in the next 12-18 months, investor enthusiasm may begin to fade.”
Rangan and Sheridan: Long-term Optimists
A somewhat more positive perspective on genAI, at least in the long-term, was expressed by Kash Rangan and Eric Sheridan, both Senior Equity Research Analysts at GS.
Noting that “hardly a week goes by without reports of a new, and better, AI model,” Rangan said he remained enthusiastic about genAI long-term potential, but acknowledged, “we have yet to identify AI’s ’killer application.’ ”
Similarly, while acknowledging that the technology “is still very much a work in progress,” Sheridan said “it’s impossible to sit through demonstrations of generative AI’s capabilities at company events or developer conferences and not come away excited by the long-term potential.”
Rangan acknowledges that “AI technology is undoubtedly expensive today” but argues (in contrast to Covello) that “the cost-equation will change.”
Pointing out that “people tend to overestimate a technology’s short-term effects and underestimate its long-term effect,” Rangan adds “Nobody today can say what killer applications will emerge from AI technology. But we should be open to the very real possibility that AI’s cost equation will change, leading to the development of applications that we can’t yet imagine.”
Sheridan agrees the economics of AI are challenging now: “I readily acknowledge that the return on invested capital (ROIC) visibility is currently low, and the transformative potential of AI will remain hotly debated until that becomes clearer.”
He concludes, “people didn’t think they needed smartphones, Uber, or Airbnb before they existed. But today it seems unthinkable that people ever resisted such technological progress. And that will almost certainly prove true for generative AI technology as well.”
Concluding thoughts
I remain optimistic and energized by the promise to be found at the intersection of AI and biotechnology, and view digital technologies like AI as increasingly essential tools for understanding biology as well as for effectively managing biopharma R&D. But even if at times genAI seems magical, we can’t treat it as magic. We can’t operationalize it as magic. We can’t invoke it as a force that will descend from the rafters, deus ex machina, and somehow fix what ails our organizations. Nor should we set it aside, dismissing it simply the newest new thing. By steering a path between credulous mysticism on the one hand, and reflexive cynicism on the other, we can inquisitively explore and thoughtfully interrogate this powerful emerging technology, and identify meaningful opportunities for productive application in biopharma R&D.