Learning From History How to Think About the Technology of the Moment
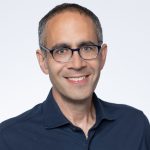
David Shaywitz
Generative AI, the transformative technology of the moment, exploded onto the scene with the arrival in late 2022 of chatGPT, an AI-powered chatbot developed by the company OpenAI.
After only five days, a million users had tried the app; after two months: 100 million, the fastest growth ever seen for a consumer application. TikTok, the previous record holder, took nine months to reach a 100 million users; Instagram had taken 2.5 years.
Optimists thrill to the potential AI offers humanity (“Why AI Will Save The World”), while doomers catastrophize (“The Only Way To Deal With The Threat From AI? Shut It Down”). Consultants and bankers offer frameworks and roadmaps and persuade anxious clients they are already behind. Just this week, McKinsey predicted that generative AI could add $4.4 trillion in value to global economy. Morgan Stanley envisions a $6 trillion opportunity in AI as a whole, while Goldman Sachs says 7% of jobs in the U.S. could be replaced by AI.
The one thing everyone — from Ezra Klein at the New York Times to podcasters at the Harvard Business Review — seems to agree on is that generative AI “changes everything.”
But if we’ve learned anything from previous transformative technologies, it’s that at the outset, nobody has any real idea how these technologies will evolve, much less change the world. When Edison invented the phonograph player, he thought it might be used to record wills. The internet arose from a government effort to enable decentralized communication in case of enemy attack.
As we start to contemplate – and are thrust into – an uncertain future, we might take a moment to see what we can learn about technology from the past.
***
Yogi Berra, of course, observed that “it is difficult to make predictions, especially about the future,” and forecasts about the evolution of technology bear him out.
In 1977, Ken Olsen, President of Digital Equipment Corporation (DEC), told attendees of the World Futures Conference in Boston that “there is no reason for any individual to have a computer in their home.” In 1980, the management consultants at McKinsey projected that by 2000, there might be 900,000 cell phone users in the U.S.; they were off by over 100-fold; the actual number was above 119 million.
On the other hand, much-hyped technologies like 3D TV, Google Glass, and the Segway never really took off. For others, like cryptocurrency and virtual reality, the verdict is still out.
AI itself has been notoriously difficult to predict. For example, in 2016, AI expert Geoffrey Hinton declared:
“Let me start by just saying a few things that seem obvious. I think if you work as a radiologist, you’re like the coyote that’s already over the edge of the cliff but hasn’t yet looked down, so doesn’t realize there’s no ground underneath him. People should stop training radiologists now. It’s just completely obvious that within five years, deep learning is going to do better than radiologists because it’s going to get a lot more experience. It might be 10 years, but we’ve got plenty of radiologists already.”
Writing five years after this prediction, in his book The New Goliaths (2022), Boston University economist Jim Bessen observes that “no radiology jobs have been lost” to AI, and in fact, “there’s a worldwide shortage of radiologists.”
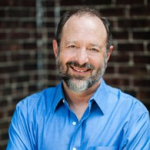
James Bessen, Executive Director of the Technology & Policy Research Initiative, Boston University.
As Bessen notes, we tend to drastically overstate job losses due to new technology, especially in the near term. He calls this the “automation paradox,” and explains that new technologies (including AI) are “not so much replacing humans with machines as they are enhancing human labor, allowing workers to do more, provide better quality, and do new things.”
Following the introduction of the ATM machine, the number of bank tellers employed actually increased, Bessen reports. Same for cashiers after the introduction of the bar code scanner, and for paralegals after the introduction of litigation-focused software products.
The explanation, Bessen explains, is that as workers are more productive, the costs of what their making tends to go down, which often unleashes greater consumer demand – at least up to a point.
For instance, automation in textiles enabled customers to afford not just a single outfit, but an entire wardrobe. Consequently, from the mid-nineteenth century to the mid-twentieth century, “employment in the cotton textile industry grew alongside automation, even as automation was dramatically reshaping the industry,” Bessen writes.
Yet after around 1940, automation continued to improve the efficiency of textile manufacturing, but consumer demand was largely sated; consequently, he says, employment in the U.S. cotton textile industry has decreased dramatically, from around 400,000 production workers in the 1940s to less than 20,000 today.
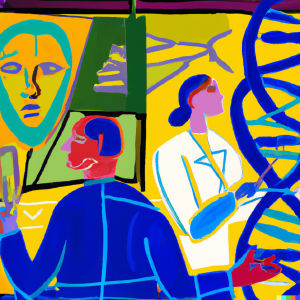
Innovation image created on DALL-E.
The point is that if historical precedent is a guide, the introduction of a new technology like generative AI will be accompanied by grave predictions of mass unemployment, as well as far more limited, but real examples of job loss, as we’ve seen in recent reporting. In practice, generative AI is likely to alter far more jobs than it eliminates and will likely create entirely new categories of work.
For example, Children’s Hospital in Boston recently advertised for the role “AI prompt engineer,” seeking a person who skilled at effectively interacting with chatGPT.
More generally, while it can be difficult to predict exactly how a new technology will evolve, we can learn from the trajectories previous technological revolutions have followed, as economist Carlota Perez classically described in her 2002 book, Technological Revolutions and Financial Capital.
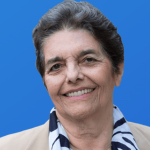
Carlota Perez, Honorary Professor at the Institute for Innovation and Public Purpose (IIPP) at University College London.
Among Perez’s most important observations is how long it takes to realize “the full fruits of technological revolutions.” She notes that “two or three decades of turbulent adaptation and assimilation elapse from the moment when the set of new technologies, products, industries, and infrastructures make their first impact to the beginning of a ‘golden age’ or ‘era of good feeling’ based on them.”
The Perez model describes two broad phases of technology revolutions: installation and deployment.
The installation phase begins when a new technology “irrupts,” and the world tries to figure out what it means and what to do with it. She describes this as a time of “explosive growth and rapid innovation,” as well as what she calls “frenzy,” characterized by “flourishing of the new industries, technology systems, and infrastructures, with intensive investment and market growth.” There’s considerable high-risk investment into startups seeking to leverage the new technology; most of these companies fail, but some achieve outsized, durable success.
It isn’t until the deployment phase that the technology finally achieves wide adoption and use. This period is characterized by the continued growth of the technology, and “full expansion of innovation and market potential.” Ultimately, the technology enters the “maturity” stage, where the last bits of incremental improvement are extracted.
As Perez explained to me, “A single technology, however powerful and versatile is not a technological revolution.” While she describes AI as “an important revolutionary technology … likely to spawn a whole system of uses and innovations around it,” she’s not yet sure whether it will evolve into the sort of full-blown technology revolution she has previously described.
One possibility, she says, is that AI initiates a new “major system” – AI and robotics – within an ongoing information and communication technology revolution.
At this point, it seems plausible to imagine we’re early in the installation stage of AI (particularly generative AI), where there’s all sorts of exuberance, and an extraordinary amount of investing and startup activity. At the same time, we’re frenetically struggling to get our heads around this technology and figure out how to most effectively (and responsibly) use it.
This is normal.
Technology, as I wrote in 2019, “rarely arrives on the scene fully formed—more often it is rough-hewn and finicky, offering attractive but elusive potential.”
As Bessen has pointed out, “invention is not implementation,” and it can take decades to work out how best to use something novel. “Major new technologies typically go through long periods of sequential innovation,” Bessen observes, adding, “Often the person who originally conceived a general invention idea is forgotten.”
The complex process associated with figuring out how to best utilize a new technology may account, at least in part, for what’s been termed the “productivity paradox” – the frequent failure of a new technology to impart significant productivity improvement. We think of this frequently in the context of digital technology; economist Robert Solow wryly observed in a 1987 New York Times book review that “You can see the computer age everywhere but in the productivity statistics.”
However, as Paul A. David, an economic historian at Stanford noted in his classic 1990 paper, “The Dynamo and the Computer,” a remarkably similar gap was present a hundred years earlier, in the history of electrification. David writes that the dawn of the 20th century, two decades after the invention of the incandescent light bulb (1879) and the installation of Edison central generating stations in New York and London (1881), there was very little economic productivity to show for it.
David goes on to demonstrate that the simple substitution of electric power for steam power in existing factories didn’t really improve productivity very much. Rather, it was the long subsequent process of iterative reimagination of factories, enabled by electricity, that allowed the potential of this emerging technology to be fully expressed.
A similar point is made by Northwestern economic historian Robert Gordon in his 2016 treatise The Rise and Fall of American Growth. Describing the evolution of innovation in transportation, Gordon observes that “most of the benefits to individuals came not within a decade of the initial innovation, but over subsequent decades as subsidiary and complementary sub-inventions and incremental improvements became manifest.”
As Bessen documents in Learning by Doing (2015), using examples ranging from the power loom (where efficiency improved by a factor of twenty), to petroleum refinement, to the generation of energy from coal, remarkable improvements occurred during the often-lengthy process of implementation, as motivated users figured out how to do things better — “learning by doing.”
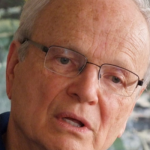
Eric von Hippel, professor, MIT Sloan School of Management
Many of these improvements (as I’ve noted) are driven by what Massachusetts Institute of Technology professor Eric von Hippel calls “field discovery,” involving frontline innovators motivated by a specific, practical problem they’re trying to solve.
Such innovative users—the sort of people who Judah Folkman had labeled “inquisitive physicians”—play a critical role in discovering and refining new products, including in medicine; a 2006 study led by von Hippel of new (off-label) applications for approved new molecular entities revealed that nearly 60% were originally discovered by practicing clinicians.
***
What does this history of innovation mean for the emerging technology of the moment, generative AI?
First, we should take a deep breath, and recognize that we are in the earliest days of technology evolution, and nobody knows how it’s going to play out. Not the experts developing it, not the critics bemoaning it, not the consultants trying to sell work off our collective anxiety around it.
Second, we should acknowledge that the full benefits of the technology will take some time to appear. Expectations of immediate gains in productivity by plugging in AI seem as naïve, the dynamo-for-steam substitution all over again. While there are clearly some immediate uses for generative AI, the more substantial benefits will likely require continued evolution of both technology and workflow processes.
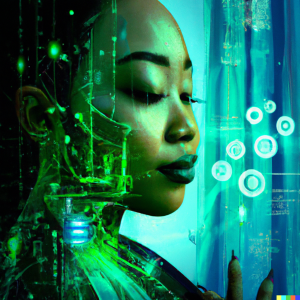
Innovation image created on DALL-E.
Third, it’s unlikely that AI will replace most workers, but it will require many of us to change how we get our jobs done – an exciting opportunity for some, an unwelcome obligation for others. AI will also create new categories of work, and introduce new challenges for governance, ethics, regulation, and privacy.
Fourth, and perhaps most importantly: As mind-blowing as generative AI is, the technology is not magic. It doesn’t descend from the heavens (or Silicon Valley), deus ex machina, with the ability to resolve sticky ethical challenges, untangle complex biological problems, and generally ease the woes of humanity.
But while technology isn’t a magic answer, it’s proved a historically valuable tool, driving profound improvements in the human condition, and enabling tremendous advances in science. The discovery of the microscope, telescope, and calculus all allowed us to better understand nature, and to develop more impactful solutions.
Technology changes the world in utterly unexpected and unpredictable ways.
How exciting to live in this moment, and to have the opportunity — and responsibility — to observe and shape the evolution of a remarkable technology like generative AI.
Yes, there are skeptics. I have a number of friends and colleagues who have decided to sit this one out, reflexively dismissing the technology because they’ve heard it hallucinates (it does), or because of privacy concerns (a real worry), or because they’re turned off by the relentless hype (I agree!)
But I would suggest that we owe it to ourselves to engage with this technology, familiarize ourselves, through practice, with its capabilities and limitations.
We can be the lead users generative AI — like all powerful but immature transformative technologies — requires to evolve from promise to practice.
Recent Astounding HealthTech columns on Generative AI
- Generative AI: No Humbug
- Welcome to the AI Irruption
- New Book Contemplates How Generative AI Will Serve Man
- Tech, Pharma, and the Uneven Distribution of the AI-Enabled Future
- Big, If True: Opportunities and Obstacles Facing AI
- Pharma R&D Execs Offer Extravagant Expectations for AI But Few Proof Points