Generative AI: No Humbug
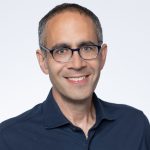
David Shaywitz
In 1845, dentist Horace Wells stood before Harvard medical students and faculty, eager to demonstrate the utility of nitrous oxide – laughing gas – as a general anesthetic.
Wells tried it out on a patient who needed a tooth extraction. The dose, it turned out, wasn’t enough. The patient screamed in agony.
As described by Paul Offit in You Bet Your Life (my 2021 WSJ review here), the demonstration elicited “peals of laughter from the audience, some of whom shouted, ‘Humbug!’ Wells left the building in disgrace.”
About a year and a half later, another dentist, Charles Morton, conducted a similar demonstration, using ether as the anesthetic instead. In front of an audience at an auditorium at the Massachusetts General Hospital (MGH), Morton excised a large tumor from the jaw of a 20-year-old housepainter named Gilbert Abbott. Abbott slept through the entire procedure.
When the operation was complete, John Collins Warren, a professor of surgery at MGH who had hosted both demonstrations, “looked at the audience and declared, ‘Gentleman – this is no humbug.’”
Today, the smartest and most skeptical academic experts I know are floored by a different emerging technology: generative AI. There seems to be a rush among healthtech investors to back startups leveraging AI to solve specific problems in biomedicine and healthcare. Meanwhile, incumbent biopharma and healthcare stakeholders are (or soon will be) urgently contemplating how and where to leverage generative AI – and where their own troves of unique data might be utilized to fine tune AI models and generate distinctive insight.
It seems time to declare, “Generative AI is no humbug.”
Hope Beyond The Hype
I’ve started with this 19th century story to remind us that physicians and scientists have always struggled to assess the promise of emerging technologies.
Today, the hype around generative AI is off the charts. “A New Area of A.I. Booms, Even Amid the Tech Gloom,” reads a recent New York Times headline. It continues, “An investment frenzy over ‘generative artificial intelligence’ has gripped Silicon Valley, as tools that generate text, images and sounds in response to short prompts seize the imagination.”
It’s reasonable to wonder whether this is just the latest shiny tech object that arrives with dazzling promise only to fizzle out, never meaningfully impacting the way care is delivered and the way drugs are discovered and developed.
So far, AI hasn’t really moved the needle in healthcare, as a remarkably blunt recent post from the Stanford Institute for Human-Centered AI (HAI) acknowledges.
But I believe generative AI offers something different, and profound — a perspective shared by the Stanford HAI authors. Generative AI is an area with which we should (and arguably, must) engage deeply, rather than merely follow with detached, bemused interest.
Generative AI and Chat-GPT
What is generative AI? You can ask the AI itself. According to Chat-GPT, openAI’s wildly popular demonstration model of the technology, generative AI, “refers to a type of artificial intelligence that generates new data, such as text, images, or sound, based on a set of training data.”
That explanation is well and good, but if you really want to viscerally appreciate some of the power of the technology, you really need to — and owe it to yourself to — experience it. Go to chat.openai.com, sign up for free, and try chat-GPT for yourself. It’s unbelievable in a way that you need to engage with to really understand. The specific examples always seem trivial, but the range and fluidity of the responses the technology provides is extraordinary.
For example, I asked it to write a commentary about climate change from the perspective of Bernie Sanders, and then another one from the perspective of Donald Trump – the results were uncanny. One of my teenage daughters, not easily impressed, was blown away when I asked the technology to “write a 200-word essay from perspective of teenage daughter asking dad to approve [a particular app],” a highly topical subject in our household. The result was fantastic, even persuasive.
Of course, the technology isn’t perfect, and certainly not infallible – for example when I asked it about the line “Is it safe yet?” in a Dustin Hoffman movie, it correctly identified both the film (“Marathon Man”) and Hoffman’s character, but incorrectly thought it was Hoffman’s line, rather than that of his interrogator, portrayed by Laurence Olivier.
Such errors are not unusual and reflect a well-described challenge known as “hallucinations,” where the model confidently provides inaccurate information, often in the context of other information that’s accurate.
In another example, discussed by Ben Thompson at Stratechery, the model is asked about the views of Thomas Hobbes. It generates a response that Thompson describes as “a confident answer, complete with supporting evidence and a citation to Hobbes work, and it is completely wrong,” confusing the arguments of Hobbes with those of John Locke.
Not surprisingly, healthcare AI experts tend to emphasize the role of “human in the loop” systems for high stakes situations like providing diagnoses. One framing I’ve heard a lot from AI enthusiasts is “you’re not going to be replaced by a computer – you’re going to be replaced by a person with a computer.”
Large Language Models and Emergence
The capabilities behind chat-GPT are driven by a category of model known as “Large Language Models,” or LLMs. The models are trained on as much coherent text as they can find to hoover up, and are designed to recognize words found in proximity to each other.
A remarkable property of LLMs and other generative AI models is emergence: an ability that isn’t present in smaller models, but is present (and often arises, seeming abruptly) in larger models.
As two authors of a recent paper on emergence in the context of LLMs explain,
“This new paradigm represents a shift from task-specific models, trained to do a single task, to task-general models, which can perform many tasks. Task-general models can even perform new tasks that were not explicitly included in their training data. For instance, GPT-3 showed that language models could successfully multiply two-digit numbers, even though they were not explicitly trained to do so. However, this ability to perform new tasks only occurred for models that had a certain number of parameters and were trained on a large-enough dataset.”
(If you’re first thought is that of Skynet becoming sentient, I’m with you.)
Models in this category are often termed “foundation models,” since they may be adapted to many applications (see this exceptional write-up in The Economist, and this associated podcast episode). While the training of the underlying model is generally both time-consuming and expensive, the adaptation of the model to a range of specific applications can be done with relative ease, requiring only modest additional tuning.
Implications for Healthcare and Biopharma
Foundational models represent a particularly attractive opportunity in healthcare, where there’s a “need to retrain every model for the specific patient population and hospital where it will be used,” which “creates cost, complexity, and personnel barriers to using AI,” as the Stanford HAI authors observe.
They continue:
”This is where foundation models can provide a mechanism for rapidly and inexpensively adapting models for local use. Rather than specializing in a single task, foundation models capture a wide breadth of knowledge from unlabeled data. Then, instead of training models from scratch, practitioners can adapt an existing foundation model, a process that requires substantially less labeled training data.”
Foundation models also offer the ability to combine multiple modalities during training. As Eric Topol writes in a recent, essential review (see also the many excellent references within). “Foundation models for medicine provide the potential for a diverse, integration of medical data that includes electronic health records, images, lab values, biologic layers such as the genome and gut microbiome, and social determinants of health.”
At the same time, Topol acknowledges that the path forward is “not exactly clear or rapid.” Even so, he says, the opportunity to apply generative AI to a range of tasks in healthcare “would come in handy (an understatement).” (Readers interested in keeping up with advances in healthcare-related AI should consider subscribing to “Doctor Penguin,” a weekly update produced by Topol and colleagues.)
The question, of course, is how to get from here to there — not to mention envisioning and describing the “there.”
The journey won’t be easy. The allure of applying tech to healthcare and drug discovery has been repeatedly, maddeningly thwarted by a range of challenges, particularly involving data: comparatively limited data volume (vs text on the internet, say), inconsistent data quality, data accessibility, and data privacy. Other obstacles include healthcare’s notorious perverse incentives and the perennial difficulty of reinventing processes in legacy organizations (how’s your latest digital transformation working out?).
As the seasoned tech experts at the “All In” podcast recently discussed, it’s not yet clear how the enormous models underlying generative AI will find impactful expression in startups – though the interest in figuring this out is enormous. One of the hosts suggested that the underlying AI itself was likely to become commoditized, or nearly commoditized; hence,
“the real advantage will come from applications that are able to get a hold of proprietary data sets and then use those proprietary data sets to generate insights, and then layering on … reinforcement learning. If you can be the first out there in a given vertical with a proprietary data set, then you get the advantage, the moat of reinforcement learning. That would be the way to create, I think, a sustainable business.”
When you think about promising proprietary data sets, those that are owned or managed by healthcare organizations and biopharmaceutical companies certainly come to mind.
Healthtech Investors See An Opportunity
Perhaps not surprisingly, many healthtech experts are keen jump on these emerging opportunities through investments in AI-driven startups.
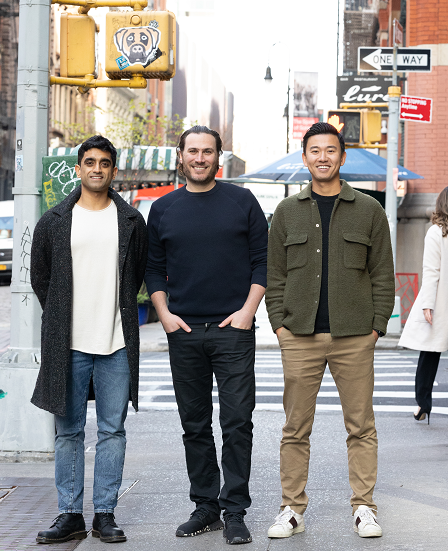
Dimension partners (L to R) Zavain Dar, Adam Goulburn, Nan Li
A new VC, Dimension, was recently launched with $350M in the bank, led by Nan Li (formerly a healthtech investor at Obvious Ventures), Adam Goulburn and Zavain Dar (both experienced healthtech investors joining from Lux Capital). They’re focused on companies at the “interface of technology and the life sciences,” and looking “is looking for platform technologies that marry elements of biotech with computing.” (TR coverage).
Healthtech and the promise of AI has also captured the attention of established biotech investors — it’s a key thesis of Noubar Afeyan’s Flagship Pioneering – and prominent tech VCs, like Andreessen Horowitz. Generative AI informs the thinking of Vijay Pande, who leads Andreessen’s Bio Fund.
Also focused on this interface: five emerging VC investors who collaborate on an thoughtful Substack focused on the evidence-based evaluation of advances (or putative advances) in Tech Bio, with a particular emphasis on AI. The contributors include Amee Kapadia, a biomedical engineer (Cantos Ventures); Morgan Cheatham, a data scientist and physician-in-training (Bessemer Venture Partners); Pablo Lubroth, a biochemical engineer and neuropharmacologist (Hummingbird Ventures); Patrick Malone, a physician-scientist (KdT Ventures); and Ketan Yerneni, a physician (also KdT Ventures).
Meanwhile, physician Ronny Hashmonay, recently announced on LinkedIn that he “is leaving Novartis, after 11.5 years,” and “is founding a new VC fund to continue working and leading the tech revolution in healthcare.”
Concluding Thoughts
It’s enormously exciting, if frequently disorienting, to participate in the installation phase of a new technology, the stage of technology development where the promise is recognized but the path to realization is less clear. Our challenge and opportunity is to help figure out how to translate, responsibly, the power and possibility associated with generative AI into tangible, meaningful benefit for patients and for science.
One final note: despite the ignominious demonstration of Horace Wells, both ether and nitrous oxide ultimately found widespread use as general anesthetics, along with chloroform. Significantly improved reagents and processes were developed, often incrementally, in the first half of the 20th century, and continuing forward. The progress in anesthetics over the last 150 years has been nothing short of remarkable.
And yet, as Offit reminds us more than 175 years later, “the exact mechanism by which they work remains unknown.”
Sounds familiar.