Big, If True: Opportunities and Obstacles Facing AI (Plus: Summer Reading)
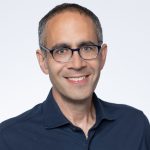
David Shaywitz
Today, we’ll begin with a consideration of the promise for AI some experts see in healthcare and biopharma.
Next, we’ll look at some of the obstacles – some technical, some organizational – and re-visit the eternal “data parasite” debate.
Finally, we’ll conclude with a few suggestions for summer reading.
The AI Opportunity: Elevating Healthcare for All
Earlier this month, I moderated a conversation about AI and healthcare (video here, transcript here) at Harvard’s historic Countway Library of Medicine, in a room just down the hall from a display of Phineas Gage’s skull and the tamping iron that lanced it on September 13, 1848, famously altering his behavior but sparing his life. The episode soon became part of neurology history and lore.
With less overt drama, but addressing a topic of perhaps even greater biomedical importance, the panelists – Harvard’s Dr. Zak Kohane, Microsoft’s Peter Lee, and journalist Carey Goldberg (all co-authors of the recently published The AI Revolution in Medicine: GPT-4 and Beyond, discussed here), addressed their subject.
A key opportunity for AI in health that Kohane emphasized was the chance to elevate care across the board by improving consistency. He told the story of a friend whose spouse was dealing with a series of difficult health issues.
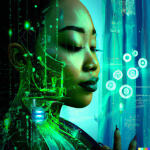
Innovation image created on DALL-E.
Kohane said his friend described “how delightful it was to have a doctor who really understood what was going on, who understood the plan. The light was on.”
However, Kohane continued, the friend would then “go talk to another doctor and another doctor, and the light was not on. And there was huge unevenness.”
The story, Kohane reflected, “reminds me of my own intuition just from experiencing medical training and medical care, which is there are huge variations. There are some brilliant doctors. But there are some also non-brilliant doctors and some doctors who might have been brilliant but then are harried, squished by the forces that propel modern medicine.”
Kohane says he saw Chat-GPT as a potential response to physician inconsistency. For Kohane, generative AI represented a disruptive force that “was going to happen, whether or not medicine and the medical establishment were going to pick up the torch.” Why? Because “patients were going to use it.”
Goldberg, too, recognized the opportunities for patients, and spoke to the urgent need she felt to access the technology:
“Okay, we get it. It has inaccuracies, it hallucinates. Just give it to me. Like, I just want it. I just want to be able to use it for my own queries, my own medically related queries. And I think that what I came away from working on this book with was an understanding of just the incredible usefulness that this can have for patients.”
Goldberg also shared a story of a nurse who suffered from hand pain and was evaluated by a series of specialists who were unable to identify the cause. Desperate, the nurse typed her symptoms into Chat-GPT, and learned that one of her medications could be causing the pain. When this was changed, the pain resolved.
Kohane sees the ready availability of a savvy second-opinion a tremendous resource for physicians. When he was training, he said, the physicians used to convene after clinic and review all the patients. “Invariably,” he notes, “we changed the management” of a handful “because of what someone else said. That went away. There’s no time for it.”
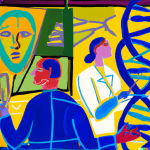
Innovation image created on DALL-E.
The lack of review represents a real loss, Kohane points out, because “even the best doctors will not remember everything all the time.” Kohane says he is convinced that generative AI will restore this capability and enable it serve a co-pilot function, providing real-time assistance to busy providers.
Another opportunity to make physicians’ lives better, the panelists suggested, was in the area of paperwork and documentation, such as the dreaded pre-authorization letters, often required to beseech payors for reimbursement.
Since Lee contributed an entire chapter about the impact on paperwork reduction in healthcare, I asked him whether we’re just going to see AI’s battling with each other: provider AI’s writing pre-authorization letters, and payor AI’s writing justifications for rejection.
Lee responded that this was very similar to a scenario Bill Gates has mentioned, where an email starts as three bullet points you want to share, GPT-4 translates this into a well-composed email, then GPT-4 at other end reduces it back to three bullet points for the reader.
I told Lee this reminded me of Peter Thiel’s famous quote: “We wanted flying cars, instead we got 140 characters.” Surely, I asked, generative AI must offer healthcare something more profound than more efficient paperwork?
In response, Lee highlighted the opportunities associated with the ability to better connect and learn from data – perhaps getting us closer to at long last fulfilling the elusive promise of a “learning healthcare system” (see here). In particular, Lee highlighted the potential of AI serving as a “universal translator of healthcare information,” allowing for the near-effortless extraction and exchange of information.
For more perspectives on how AI could benefit healthcare and the life sciences, I’d recommend:
- A recent Atlantic piece by Matteo Wong emphasized the opportunities for leveraging multi-modal data – a topic Eric Topol and colleagues have consistently highlighted.
- This short Nature Biotechnology essay, by Jean-Philippe Vert, a data science expert who now leads R&D at the health data company Owkin. Vert describes four ways AI may impact drug discovery. Of particular interest: his suggestion that generative AI might provide a “framework for the seamless integration of heterogeneous data and concepts.” However, he acknowledges that “How exactly to implement this idea and how effective it will be largely remain open research questions.”
- This recent Nature Communications paper from M.I.T. and Takeda (disclosure: I work at Takeda, but wasn’t involved in this collaboration), demonstrating an application of AI in manufacturing. This operational area seems especially amenable to AI-driven improvements, in part because the richness and completeness of data capture (see also here).
Pesky Obstacles to AI implementation
The inconvenient truth is that while generative AI and other emerging technologies have captivated us with their promise, we’re still figuring out how to use them.
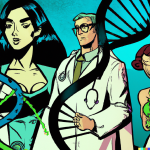
Innovation image created with DALL-E.
Even user-friendly applications like ChatGPT and GPT-4-enabled-Bing are not always plug-and-play. For example, in preparation for an upcoming workshop I’m leading for a particular corporate function highlighting the capabilities of GPT-4, I tried out some of the team’s most elementary use cases with Bing-GPT. The results were disappointing and included a number of basic mistakes. Often, Bing-GPT seemed to perform worse than Bing or Google search alone. The results generated seemed unlikely to inspire corporate colleagues to urgently adopt the technology.
These challenges are hardly limited to GPT-4 or Bing. From the perspective of a drug development organization, technology issues seem to surface in every area of digital and data. Far more often than not, the hype and promise touted by eager startups seem at odds with the capabilities these nascent companies demonstrably can deliver. In fairness, the difficulty many legacy biopharma companies have figuring out how to work in new ways with these healthtech startups probably also contributes to the challenge.
To understand the issues better, let’s consider one example, outside of biopharma, recently discussed by University of North Carolina Vice Chair and Professor of Medicine Spencer Dorn. His focus: the adoption of AI in radiology.
Dorn notes that while AI expert Geoffrey Hinton predicted in 2016 that AI would obviate the need for radiologists within five years, this hasn’t happened. In fact, Dorn says, only a third of radiologists use AI at all, “usually for just a tiny fraction of their work.”
Dorn cites several reasons for AI’s limited adoption in clinical radiology:
- Inconsistent performance of AI in real-world settings, compared to test data;
- AI “may be very good a specific tasks (e.g. identifying certain lesions)…but not most others”;
- “Embedding AI into diagnostic imaging workflows require time, effort, and money,” and basically, the juice doesn’t seem to be worth the squeeze;
Dorn warns that generative AI “in healthcare will need to overcome these same hurdles. Plus, several more.”
Similar issues apply to the adoption, for high-stakes use-cases, of a range of emerging technologies, including digital pathology, decentralized trials, and “the nightmare” of digital biomarkers – challenges this column has frequently discussed.
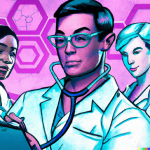
Innovation image created on DALL-E.
But remarkably, technology problems are probably not the most difficult issue for healthtech innovators to solve. Technology tends to improve dramatically over time (think about the camera on your smartphone). No, the most difficult sticking point may well be organizational behavior. Essentially, the return of the eternal, dreaded “Data Parasite” debate (as I discussed in 2016 in a three-part series in Forbes, starting here.)
In most large organizations, both academic and corporate (I am unaware of many exceptions), there is a constant battle between those who effectively own the data and those who want to analyze the data. In theory, of course, and depending upon the situation, data belong to: patients / the organization / taxpayers, or some combination of the three. Researchers, meanwhile, are just “stewards” or “trustees” of the data. Yet in practice, someone always seems to control and zealously guard the access to any given data set within an organization.
Typically, those who “own” the data (whether an academic clinical investigator or a pharma clinical development team) are using the data to pursue a defined, high-value objective. Others who want access to these data tend to have more exploratory tasks in mind. Theoretically, there’s a huge amount of value that can be obtained by enabling data exploration. Once again, in practice, the theoretical value is often difficult to demonstrate, and is often viewed as offering little upside – and a fair amount of perceived downside risk, as well as gratuitous aggravation – to the data “owners.” Much of this perceived risk relates to the concern about sloppy or ill-informed analyses that generates, essentially, “false positive” concerns, as I allude to here.
I’ve seen very few examples where the data “analyzers” have sufficient leverage to win here. In general, the data “owners” tend to hire data scientists of their own and say “let us know what you want to know, and we’ll have our people run the analysis for you.” This has the effect of slowing down integrative exploratory analyses to a trickle, particularly given the degree of pre-specification the data “owners” tend to require.
If you are a data owner, you probably view this as an encouraging result, since analyses are only done by people who ostensibly have a feel for how the data were generated and understand the context and the limitations. As discussed in a previous column, “data empathy” is vitally important.
But if you are a data analyzer not working directly with a data “owner,” you are constantly frustrated by the near-impossibility of obtaining access to data you’d like to explore. Perhaps most strikingly, many researchers who fiercely defend their own data from external analyses are often fiercely critical of others for not sharing data the same researchers hope to explore. As Miles famously observed, “where you stand depends on where you sit.”
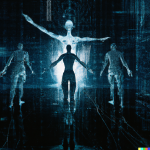
Innovation image created on DALL-E.
Of course, it’s possible that technology could help ease sharing. Even so, it’s really difficult to envision the tight hold on data changing, so long as so much power in organizations clearly rests with those in control of the data. Perhaps, as Lakhani and others suggest, this can be addressed in new companies who have a fundamentally different view of data (Amazon – driven by the “Bezos Mandate” — is the canonical example), and can readily monetize data fluidity. Alternatively, the demonstrated utility of exploratory integrated analyses across multiple data silos and “owners” in legacy organizations could potentially facilitate more consistent access.
For now, in both academia and biopharma, virtuous stated preferences to the contrary, this revealed tension remains very much alive.
Briefly Noted Summer Reading
A must-read for all biotechies, For Blood and Money, by Marketwatch’s Nathan Vardi, tells the captivating story of two cancer medicines targeting the BTK kinase: ibrutinib and acalabrutinib. A decade ago, for Forbes, I wrote about the beginning of the ibrutinib story.
It was thrilling to read Vardi’s account of the medicine’s complete journey – and the journey of its competitor, acalabrutinib (which, fun fact, was originally discovered by the same company in the Netherlands that discovered the product that became the blockbuster Keytruda, see here). As Jerome Groopman’s thoughtful review in the New York Review of Books suggests, Vardi’s book also raises difficult questions about the role of luck vs skill in drug development, as well as the role of capital vs labor, since the investors appeared to make out far better than the scientists who did the lion’s share of the work. This pithy review by Adrian Woolfson, in Science, also provides a good summary.
Less essential but fascinating for readers who recall the rise of companies like Gawker and Buzzfeed, is Traffic, by Ben Smith. He describes how emerging media companies – and the young men and women who contributed the content – desperately chased reader traffic, with important consequences both for them and society. See here for an excellent review of the book by the Bulwark’s Sonny Bunch.
Also intriguing, if a bit uneven: Beyond Measure, a book about the history of measurement, written by James Vincent, Senior Reporter at The Verge. See here for a thoughtful review of Vincent’s book by Jennifer Szalai in The New York Times.
Finally, a few recommended posts. On the concerning side, this piece about the devolution of clinical medicine captures what I seem to be hearing from nearly every single physician I know. Even doctors who were once so excited about taking care of patients now seem abjectly miserable, trapped in a system that has reduced them to widgets. (See also here, here, here.)
On the innovation front, several comments about the wildly popular GLP-1 medicines tirzepatide and semaglutide caught my eye (see also my last piece, here). On the one hand, it’s clear the development of these powerful and promising medicines was, as Dr. Michael Albert of Accomplish Health suggests, clearly the result of deliberate, meticulous effort, both by companies like Lilly and Novo Nordisk, and pioneering academics like physician-scientist Daniel Drucker (who also maintains this authoritative website on the evolving science). On the other hand, it’s interesting that (as Sarah Zhang writes in The Atlantic), these medicines may have entirely unanticipated application in the management of addictions and compulsions.
Bottom Line
Generative AI offers the possibility of elevating the quality of healthcare patients receive. However, the implementation of AI and other digital technologies may be impaired both by the growing pains of nascent technology and, more significantly, by the territoriality of those who control access to data silos within large organizations — although this may also ensure that the data are more likely to be analyzed by those who have a greater feel for the context in which they were generated). Finally, For Blood and Money, by Nathan Vardi, Traffic, by Ben Smith, and Beyond Measure, by James Vincent are all good additions to your summer reading list.