Pharma R&D Execs Offer Extravagant Expectations for AI But Few Proof Points
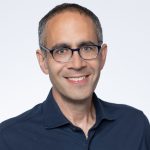
David Shaywitz
As the excitement around generative AI sweeps across the globe, biopharma R&D groups (like most everyone else) are actively trying to figure out how to leverage this powerful but nascent technology effectively, and in a responsible fashion.
In separate conversations, two prominent pharma R&D executives recently sat down with savvy healthtech VCs to discuss how generative AI specifically, and emerging digital technologies more generally, are poised to transform the ways new medicines are discovered, developed, and delivered.
The word “poised” is doing quite a lot of work in the sentence above. Both conversations seamlessly and rather expertly blend what’s actually been accomplished (a little bit) with the vision of what might be achieved (everything and then some).
The first conversation, from the a16z “Bio Eats World” podcast, features Greg Meyers, EVP and Chief Digital and Technology Officer of Bristol Myers Squibb (BMS), and a16z Bio+Health General Partner Dr. Jorge Conde. The second discussion, from the BIOS community, features Dr. Frank Nestle, Global Head of Research and CSO, Sanofi, and Flagship Pioneering General Partner and Founder and CEO of Valo Health, Dr. David Berry. (Readers may recall our discussion of a previous BIOS-hosted interview with Dr. Nestle, here.)
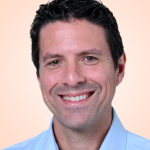
Greg Meyers, chief digital and technology officer, Bristol Myers Squibb
Rather than review each conversation individually, I thought it would be more useful to discuss common themes emerging from the pair of discussions.
Theme 1: How Pharma R&D organizations are meaningfully using AI today
AI has started to contribute meaningfully to the design of small molecules in the early stages of drug development. “A few years ago,” Meyers says, BMS started “to incorporate machine learning to try to predict whether or not a certain chemical profile would have the bioreactivity you’re hoping.” He says this worked so well (producing a “huge spike” in hit rate) that they’ve been trying to scale this up.
Meyers also says BMS researchers “are currently using AI pretty heavily in our protein degrader program,” noting “it’s been very helpful” in enabling the team to sort through different types of designs.
Nestle also highlights the role of AI in developing novel small compounds. “AI-empowered models” are contributing to the design of modules, he says, and are starting to “shift the cycle times” for the industry.
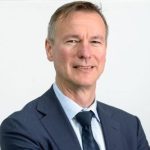
Frank Nestle, chief scientific officer, Sanofi
AI is also now contributing to the development of both digital and molecular biomarkers. For example, Meyers described the use of AI to analyze a routine 12-lead ECG to identify patients who might have undiagnosed hypertrophic cardiomyopathy. (Readers may recall a very similar approach used by Janssen to diagnose pulmonary artery hypertension, see here.)
Nestle offered an example from digital pathology. He described a collaboration with the healthtech company Owkin, whose AI technology, he says, can help analyze the microscope slides with classically stained tissue samples.
Depending on your perspective, these use cases are either pretty slim pickings or an incredibly promising start.
I’ve not included what seemed to me as still exploratory efforts involving two long-standing industry aspirations:
- Integrating multiple data sources to improve target selection for drug development;
- Integrating multiple data sources to improve patient selection for clinical trials.
We’ll return to these important but elusive ambitions later, in our discussion of “the magic vat.”
I’ve also not included examples of generative AI, because I didn’t hear much in the way of specifics here, probably because it’s still such early days. There was clearly excitement around the concept that, as Meyers put it, “proteins are a lot like the human language,” and hence, large language models might be gainfully applied to this domain.
Theme 2: Grand Vision
The aspiration for AI in in biopharma R&D were as expansive as the established proof points were sparse. The lofty idea seems to be that with enough data points and computation, it will eventually be possible to create viable new medicines entirely in silico. VC David Berry described an “aspiration to truly make drug discovery and development programmable from end to end.” Nestle wondered about developing an effective antibody drug “virtually,” suggesting it may be possible in the future. Also possible, he suggests: “the ability to approve a safe and effective drug in a certain indication, without running a single clinical trial.”
Both Nestle and Meyers cited the same estimate – 10^60 – as the size of “chemical space,” the number of different drug-like molecular structures that are theoretically possible. It’s a staggering number, more than the stars in the universe, and likely far beyond our ability to meaningfully comprehend. The point both executives were making is that if we want to explore this space productively, we’re going to get a lot further using sophisticated computation than relying on the traditional approaches of intuition, trial and error.
The underlying aspiration here strikes a familiar chord for those of us who remember some of the more extravagant expectations driving the Human Genome Project. For instance, South African biologist Sydney Brenner reportedly claimed that if he had “a complete sequence of DNA of an organism and a large enough computer” then he “could compute the organism.” While the sequencing of the genome contributed enormously to biomedical science, our understanding of the human organism remains woefully incomplete, and largely uncomputed. It’s easy to imagine that our hubris – and our overconfidence in our ability to domesticate scientific research, as Taleb and I argued in 2008 – may be again deceiving us.
Theme 3: Learning Drug Development Organization
For years, healthcare organizations have strived towards the goal of establishing a “learning health system (LHS),” where knowledge from each patient is routinely captured and systematically leveraged to improve the care of future patients. As I have discussed in detail (see here), the LHS is an entity that appears to exists only as ideal with the pages of academic journals, rather than embodied in the physical world.
Many pharma organizations (as I’ve discussed previously) aspire towards a similar vision, and seek to make better use of all the data they generate. As Meyers puts it, you “want to make sure that you never run the same experiment twice,” and you want to capture and make effective use of the digital “exhaust” from experiments, in part by ensuring it’s able to be interpreted by computers.
Berry emphasized that a goal of the Flagship company Valo (where he now also serves as CEO) is to “use data and computation to unify how… data is used across all of the steps [of drug development], how data is shared across the steps.” Such integration, Berry argues, “will increase probably of success, will help us reduce time, will help reduce cost.”
The problem – as I’ve discussed, and as Berry points out, is that “drug discovery and development has historically been a highly siloed industry. And the challenge is it’s created data silos and operational silos.”
The question, more generally is how to unlock the purported value associated with, as Nestle puts it, the “incredible treasure chest of data” that “large pharmaceutical companies…sit on.”
Historically, pharma data has been collected with a single, high-value use in mind. The data are generally not organized, identified, and architected for re-use. Moreover, as Nestle emphasizes, the incentives within pharma companies (the so-called key performance indicators or “KPIs”) are “not necessarily in the foundational space, and that not where typically the resourcing goes.” In other words, what companies value and track are performance measures like speed of trial recruitment; no one is really evaluating data fluidity, and unless you can directly tie data fluidity to a traditional performance measure, it will struggle to be prioritized.
In contrast, companies like Valo; other Flagship companies like Moderna; and some but not all emerging biopharma companies are constructed (or reconstructed — eg Valo includes components of both Numerate and Forma Therapeutics, as well as TARA biosystems) with the explicit intention of avoiding data silos. This concept, foundational to Amazon in the context of the often-cited 2002 “Bezos Memo,” was discussed here.
In contrast, pharmas have entrenched silos; historically, data were collected to meet the specific needs of a particular functional group, responsible for a specific step in the drug development process. Access to these data (as I recently discussed) tends to be tightly controlled.
Data-focused biotech startups tend to look at big pharma’s traditional approach to data and see profound opportunities for disruption. Meanwhile, pharmas tend to look at these data-oriented startups and say, “Sure, that sounds great. Now what have you got to show for all your investment in this?”
The result is a standoff of sorts, where pharmas try to retrofit their approach to data yet are typically hampered by the organizational and cultural silos that have very little interest in facilitating data access. Meanwhile, data biotech startups are working towards a far more fluid approach to data, yet have produced little tangible and compelling evidence to date that they are more effective, or are likely to be more effective, at delivering high impact medicines to patients.
Theme 4: Partnerships and External Innovation
Both BMS and Sanofi are exploring emerging technologies through investments and partnerships with a number of healthtech startups, even as both emphasize that they are also building internal capabilities.
“We have over 200 partnerships,” Meyers notes, “including several equity positions with other companies that really come from the in silico, pure-play sort of business. And we’ve learned a ton from them.”
Similarly, Nestle (again – see here) emphasized key partnerships, including the Owkin relationship and digital biomarker work with MIT Professor Dina Katabi.
Meanwhile, Pfizer recently announced an open innovation competition to source generative AI solutions to a particular company need: creating clinical study reports.
In addition to these examples, I’ve become increasingly aware of a number of other AI-related projects attributed to pharma companies that upon closer inspection, turn out to represent discrete engagements with external partners or vendors who reportedly are leveraging AI.
Theme 5: Advice for Innovators
One of the most important lessons from both discussions was the challenge for aspiring innovators and startups.
Berry, for example, explained why it’s so difficult for AI approaches to gain traction. “If I want to prove, statistically, that AI or an AI component is doing a better job, how many Phase Two clinical readouts does one actually need to believe it on a statistical basis? If you’re a small company and you want to do it one by one, it’s going to take a few generations. That’s not going to work.”
On the other hand, he suggested “there are portions of the drug discovery and development cascade where we’re starting to see insights that are actionable, that are tangible, and the timelines of them and the cost points of them are so quickly becoming transformative that it opens up the potential for AI to have a real impact.”
Meyers, for his part, offered exceptionally relevant advice for AI startups pitching to pharma (in fact, the final section of the episode should be required listening for all biotech AI founders).
Among the problems Meyers highlights – familiar to readers of this column – are the need “for companies that are focused on solving a real-world problem,” rather than solutions in search of a problem. He also emphasized that “this is an industry that will not adopt something unless it is, really 10x better than the way things are historically done.”
This presents a real barrier to the sort of incremental change that may hard be appreciate in the near term but can deliver appreciable value over time. Even “slight improvements” in translational predictive models, as we recently learned from Jack Scannell, can deliver outsized impact, significantly elevating the probability of success while reducing the many burdens of failure.
Meyers also reminded listeners of the challenges of finding product-market fit because healthcare “is the only industry where the consumer, the customer, and the payor are all different people and they don’t always have incentives that are aligned.” (See here.)
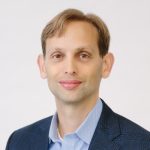
David Berry, CEO, Valo Health
On a more optimistic note, Berry noted that one of the most important competitive advantages a founder has is recognizing that “a problem is solvable, because that turns out to be one of the most powerful pieces of information.” For Berry, the emergence of AI means that “we can start seeing at much larger scales problems that are solvable that we didn’t previously know to be solvable.” Moreover, he argues, once we realize a problem is solvable, we’re more likely to apply ourselves to this challenge.
Paths Forward
In thinking about how to most effectively leverage AI, and digital and data more generally, in R&D, I’m left with two thoughts which are somewhat in tension.
“Pockets of Reducibility”
The first borrows (or bastardizes) a phrase from the brilliant Stephen Wolfram: look for pockets of reducibility. In other words – focus your technology not on fixing all of drug development, but on addressing a specific, important problem that you can meaningfully impact.
For instance, I was speaking earlier this week with one of the world’s experts on data standards. I asked him how generative AI as “universal translator” (to use Peter Lee’s term) might obviate the need for standards. While the expert agreed conceptually, his immediate focus was on figuring out how to pragmatically apply generative AI tools like GPT-4 to standard generation so that it could be done more efficiently, potentially with people validating the output rather than generating it.
On the one hand, you might argue this is disappointingly incremental. On the other hand, it’s implementable immediately, and seems likely to have a tangible impact.
(In my own work, I am spending much of my time focused on identifying and enabling such tangible opportunities within R&D.)
The Magic Vat
There’s another part of me, of course, that both admires and deeply resonates with the integrated approach that companies like Valo are taking: the idea and aspiration that if, from the outset, you deliberately collect and organize your data in a thoughtful way, you can generate novel insights that cross functional silos (just as Berry says). These insights, in principle, have the potential to accelerate discovery, translation (a critical need that this column has frequently discussed, and that Conde appropriately emphasized), and clinical development.
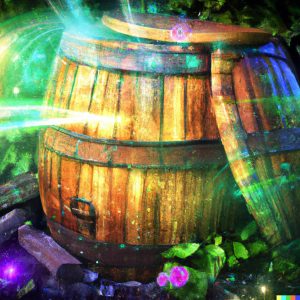
Magic Vat. Image by DALL-E.
Integrating diverse data to drive insights has captivated me for decades; it’s a topic I’ve discussed in a 2009 Nature Reviews Drug Discovery paper I wrote with Eric Schadt and Stephen Friend. The value of integrating phenotypic data with genetic data was also a key tenet I brought to my DNAnexus Chief Medical Officer role, and a lens through which I evaluated companies when I subsequently served as corporate VC.
Consequently, I am passionately rooting for Berry at Valo – and for Daphne Koller’s insitro and Chris Gibson’s Recursion. I’m rooting for Pathos, a company founded by Tempus that’s focused on “integrating data into every step of the process and thereby creating a self-learning and self-correcting therapeutics engine,” and that has recruited Schadt to be the Chief Science Officer. I’m also rooting for Aviv Regev at Genentech, and I am excited by her integrative approach to early R&D.
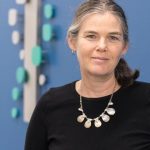
Daphne Koller, founder and CEO, insitro
But throughout my career, I’ve also seen just how challenging it can be to move from attractive integrative ambition to meaningful drugs. I’ve seen so many variations of the “magic vat,” where all available scientific data are poured in, a dusting of charmed analysis powder is added (network theory, the latest AI, etc), the mixture is stirred, and then – presto! – insights appear.
Or, more typically, not. But (we’re invariably told) these insights would arrive (are poised to arrive) if only there was more funding/more samples/just one more category of ‘omics data, etc. — all real examples by the way.
It’s possible that this time will be the charm – we’ve been told, after all, that generative AI “changes everything” — but you can also understand the skepticism.
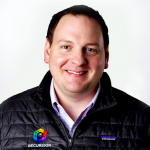
Chris Gibson, co-founder and CEO, Recursion
My sense is that legacy pharmas are likely to remain resistant to changing their siloed approach to data until they see compelling evidence that data integration approaches are, if not 10x better, then at least offer meaningful and measurable improvement. In my own work, I’m intensively seeking to identify and catalyze transformative opportunities for cross-silo integration of scientific data across at least some domains, since effective translation absolutely requires it.
For now, big pharmas are likely to remain largely empires of silos – and will continue to do the step-by-step siloed work comprising drug development at a global scale better than anyone. Technology, including AI, may help to improve the efficiency of specific steps (eg protocol drafting, an example Meyers cites). Technology may also improve the efficiency of sequential data handoffs, critical for drug development, and help track operational performance, providing invaluable information to managers, as discussed here.
But foundationally integrating scientific knowledge across organizational silos? Unless a data management organization already deeply embedded within many pharmas – perhaps a company like Veeva or Medidata – enables it, routine integration of scientific knowledge across long-established silos, in the near to medium term, seems unlikely. It may take a visionary, persistent and determined startup (Valo? Pathos?) to capture persuasively the value that must be here.
Bottom Line:
Biopharma companies are keenly interested in leveraging generative AI, and digital and data technologies more generally, in R&D. To date, meaningful implementations of AI in large pharmas seem relatively limited, and largely focused on small molecule design, and biomarker analysis (such as identifying potential patients through routine ECGs). Nevertheless, the ambitions for AI in R&D seem enormous, perhaps even fanciful, envisioning virtual drug development and perhaps even in silico regulatory approvals. More immediately, pharmas aspire to make more complete use of the data they collect but are likely to continue to struggle with long-established functional silos. External partnerships provide access to emerging technologies, but it can be difficult for healthtech startups to find a permanent foothold with large pharmas. Technology focused on alleviating important, specific problems – “pockets of reducibility” – seems most likely to find traction in the near term. Ambitious founders continue to pursue the vision of more complete data integration.