You Have Chosen … Poorly: Why Drug Developers Make Bad Decisions
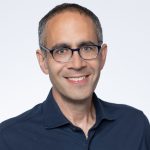
David Shaywitz
Drug development remains an incredibly expensive endeavor. Much of the cost can be attributed to late-stage clinical trial failures.
The burden is borne first and foremost by clinical trial participants who aren’t helped by the experimental medicine. It also significantly impacts the companies sponsoring these studies. Everyone would like to improve the chances that a novel medicine that advances into clinical studies ultimately will prove successful and earn approval from regulators around the world.
Yet the odds of success remain stubbornly low.
What’s the problem? (And: is AI likely to help? We’ll return to this briefly at the end.)
Let’s consider two recent perspectives on the topic.
David Grainger: remove organizational bias
We’ll start with David Grainger, a scientist, entrepreneur and VC (now Venture Advisor) at Medicxi. I’ve admired and discussed Grainger’s work for years (here, here, here). Writing in his always worthwhile “Drug Baron” blog, Grainger argues that drug developers have the data to make much smarter decisions than they actually do; the problem, he suggests, are the biases that consistently lead developers astray.
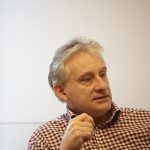
David Grainger
Among the examples Grainger cites:
Infrastructure bias – “if you have a team of employees, a lease on a building and so forth, it can be painful to call a halt even if the enthusiasm is draining away.” Since “there nearly always is” some potential path forward, “further investment becomes almost inevitable.” This is sometimes termed “inertia bias” or “status quo bias,” reflecting our shared tendency to stick with, and justify, decisions we’ve already made.
Narrative bias – the story “that companies, particularly biotechs, tend to weave around their assets to make them palatable to investors.” Grainer notes the narrative can involve technology (e.g. DNA editing), deep expertise in a particular indication (e.g. endometriosis), or a focus on a particular biological mechanism (e.g. caspases).
The problem with this thematic approach, Grainger says, is that there can be a reluctance to discontinue development of a lead asset (despite bad data), for fear of besmirching the broader narrative. It can also lead to a failure to consider promising programs outside the narrative, even if they might have a higher probability of success.
Commercial bias – large pharma companies, Grainger observes, “are usually phenomenally good” at sales, adding that “selling drugs, rather than discovering or developing them, is arguably the core competence of the biggest and best in our industry.” Because of commercial commitment to a finite number of areas and indications, Grainger writes, “the ‘gravitational pull’ from the commercial hub starts to dominate R&D decisions.” Thus, to meet the need for new products in a given area, an R&D team may “risk choosing to buy or progress inferior assets, in order to meet that imperative.”
The answer, according to Grainger, is to develop drugs in a strictly asset-focused way, deliberately seeking to avoid the biases he has described. He’s pursuing this through a Medicixi-backed company called Centessa (he’s the Chief Innovation Officer).
While Grainger is right about the existence of these biases, such as the drive to fill therapeutic area pipelines, he may underestimate the value that comes from working intensively, over time, in a given therapeutic area, and the expertise and judgement that comes from this hard-won experience.
It’s reminiscent of the logic that leads some to suggest pharma should ditch research and early development entirely, and just focus on in-licensing promising assets for late-stage development. The problem, of course, is that if you’re not actively trying to develop your own drugs in a particular area, you tend not to know what you don’t know, and absent this organic expertise – likely including the learning associated with previous failures — your ability to make informed, nuanced decisions can be limited. Perhaps this might be termed “ignorance bias.”
Another challenge with Grainger’s approach is that bias-free drug development, much like a learning health system, is an entity that’s often discussed, invariably praised, yet rarely if ever seen in the wild. Blame human nature.
Grainger, certainly, is as aware of these caveats as anyone. So credit him for not just writing about a solution but actually trying to build and inhabit it. Let’s see how it goes.
Jack Scannell: improve translational models
Next, let’s turn to Jack Scannell, whose thoughtful, scholarly musings on drug development I’ve admired for more than a decade (see here).
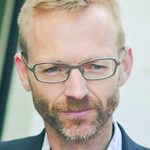
Jack Scannell
In his latest article, in Nature Reviews Drug Discovery, Scannell argues that the key success factor in drug development is “predictive validity” – how well the success of a given preclinical or translational model predicts ultimate success in clinical trials.
Everyone recognizes predictive validity is important, Scannell acknowledges, but argues we may underestimate just how important. A slight improvement in the predictive validity of a model – say increasing the correlation coefficient from 0.5 to 0.6, he says – can “often have a bigger impact on the probability of selecting a clinically useful drug candidate … than a 10x or sometimes a 100x change in the number of candidates tested.”
Phrased differently: if we had the choice, we’d be smarter to invest in a slightly better translational model than in a much larger drug screen.
Scannell points to the exploitation of translational models as one reason why pharma productivity famously declined between 1950 and 2010, despite profound advances in science and technology.
His argument is that it was precisely the ability to efficiently pursue existing translational models – he cites models of stomach acid secretion as an example – that led to an explosion of effective drugs for these conditions (e.g. H2 receptor antagonists, proton pump inhibitors), medicines that eventually went generic and raised the bar for future products .
The development of a good translational model can transform therapy, Scannell observes. He points to “the invention of the hepatitis C virus replicons” as an example, noting this approach “made it possible to produce reliable, high-titre, viral RNA replication in cell cultures, and to screen and optimize drugs.”
Pragmatically, what are drug developers to do? Scannell offers several suggestions, which, as he distilled in an email with me, include:
- Devote a lot more time and effort to evaluating models and to improving models.
- Focus on “model-able” diseases (as Vertex does, he says) and avoid those with bad models
- Think harder about calibrating models and assembling sets of complementary models.
- Fix financial incentives
The financial incentives comments reflect the observation, developed in the article, that “it is easier to capture financial value from novel drug structures than from novel decision tools,” essentially because “much of the value of the innovation can spill over to other firms at low cost.” While acknowledging “this is a difficult problem to fix,” Scannell suggests perhaps pre-competitive consortia, focused on developing decision tools (translational models) in areas of shared interest could make sense.
While immediately implementable solutions don’t leap from the pages of Scannell’s manuscript, he is almost certainly right about:
(1) the problem with inadequate predictive translational models;
(2) the undervaluing of incremental improvements in such models; and
(3) the need to pay meticulous attention to the performance of these models; otherwise, it will remain challenging to deploy them thoughtfully and to detect small but meaningful enhancements.
What about AI?
Predictably, neither Grainger nor Scannell believe AI is on the threshold of offering a solution to the fundamental problem in biopharma R&D: the need to increase the odds of success in late-stage clinical development.
For Grainger, the contribution of bias towards poor decision-making swamps any positive contribution AI might make. In his view, we don’t need AI – we have the information in hand today to make far better decisions, if only we could disentangle the many entrenched biases.
Scannell, for his part, also doesn’t believe AI is about to revolutionize drug R&D, and cites the work of Andreas Bender (whose work I’ve frequently discussed in this column – see here, here). Bender argues that AI researchers focus on computational models that don’t reflect that actual challenge that’s bedeviling R&D researchers: predicting safety and efficacy in advance of late-phase clinical development.
Academic researchers, Bender suggests, tend to focus on abstractions of drug development, artificial quantitative models that can be interrogated easily, and which produce publishable results. However, these mathematical models are largely removed from the actual questions and needs of real-world drug development, which tend to be closely enmeshed with the detailed context of the specific project. (For more on Bender’s arguments, see the slides here, and the references to which he links.)
If AI is to meaningfully impact drug development, Bender contends, we’ll need not just more data overall, but also, data that are directly relevant to the specific needs and nuances of particular drug development programs. Like Scannell, Bender proposes a role for large precompetitive consortia, though neither sound especially optimistic.
If AI isn’t likely to resolve the fundamental challenge of biopharma R&D, why is there so much excitement around (and investment in) the promise of emerging digital and data technologies?
Let me briefly suggest several possibilities:
- As I’ve previously described, the most significant impact of digital and data on biopharma, as in many other industries, appears to be in industrial efficiency, making repeatable processes, from manufacturing to shipping, flow better. Your drug may not have a higher chance of clinical success because of digital technologies (success or failure is largely baked into the molecule by the time it enters clinical studies), but you may find this out faster, and at less cost.
- Shared visibility of your data can serve as a powerful management tool, helping to align teams around a common set of information, enabling better execution. This appears to have been a critical factor (as I’ve discussed here) in Pfizer’s rapid development of their COVID vaccine.
- Technologies can help increase patient engagement (for example, in the context of decentralized clinical trials, as Lisa Suennen (now President of Digital and Data Solutions at Canary Medical) and I discussed with Craig Lipset on our Tech Tonics podcast in 2020). Deeper knowledge of patients, coupled with the savvy application of AI, can also enable earlier identification of patients who might benefit from a medication or a relevant clinical trial. One compelling example: a collaboration between the Mayo Clinic, an AI company (Anumana, a spinout of nference), and Janssen’s data science team, led by Najat Khan, revealed that patients with pulmonary artery hypertension could be flagged through a pattern identified by AI on routine electrocardiograms. This algorithm received a breakthrough device designation from the FDA in May.
- Improved data management can facilitate the collection and analysis of datasets that can generate future insights. Ideally, data around the predictive validity of translational models, as Scannell has proposed, might be evaluated rigorously, so that the models could be optimized iteratively, and applied thoughtfully.
Bottom Line
A critical challenge for drug developers is to improve their rate of clinical trial success. One cause for the low success rate: organizational biases that lead projects to continue far longer than they should. Another cause: inadequate (poorly predictive) translational models. In the short term at least, digital technologies are unlikely to meaningfully impact the success of clinical development, although programs are likely to be prosecuted more efficiently. Digital technologies are already contributing to drug development in other ways, including promoting alignment through dashboards; making trials more convenient for patients; and facilitating earlier disease diagnosis and patient identification. Eventually, improved data management and the application of appropriate analytics may lead to the generation of insights that truly make drug development smarter, so we can succeed more often, rather than fail more efficiently.