Do We Need Models Anymore?
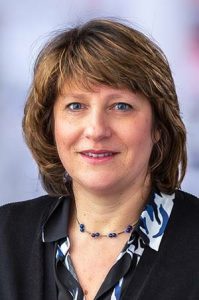
Ruth Etzioni, Full Member, Division of Public Health Sciences, Fred Hutch Cancer Center
Long ago, in the early days of the pandemic, models were everywhere in the news.
As our lives were upended and everything became uncertain, models were there to provide some predictability in the face of the unknown.
Never mind that predictions varied wildly – between models, and even within the same model at different time points. The models agreed that this was going to be bad (though at the time it was hard to believe).
They scared us, and we listened.
This is a story about models and how they help guide us as we navigate the coronavirus crisis. But it is also about guidance itself – and where to look for it.
The early models that predicted the course of the pandemic were almost uniformly epidemiologic or SEIR (Susceptible-Exposed-Infected-Recovered) compartmental models.
SEIR compartmental models envision disease as progressing through the SEIR phases and they keep track of how many people are in each phase at a given time. By using data about how long it takes to become infectious after exposure, and then how long it takes to show symptoms and making a few other assumptions, they estimate R0, the number of people a person with the virus infects, and then extrapolate into the future on the basis of this number. An effective R0 below one means the epidemic is shrinking rather than growing. A key goal of public health is to bring the R0 below this threshold number.
In Seattle, where I live, an SEIR compartmental model was developed by the Institute for Disease Modeling. The IDM is a research institute within the Bill & Melinda Gates Foundation, but is distinct from the Institute for Health Metrics and Evaluation at the University of Washington.
The Institute for Disease Modeling showed that the effective R0 had been reduced from around 3 in February — an alarming sign of a fast-spreading epidemic — to a much more manageable R0 of around 0.6 on April 15.
At that point in mid-April, with states under various stay-at-home orders, the talk was all about re-opening. We were banding together to flatten the curve, and we were making progress. People wanted to know: When would it be safe to emerge from lockdown, and go back to something more normal?
Here, models became useful too. With their compartmental model, the IDM modelers could project how the infection’s trajectory would change if activity and contact resumed at different levels and dates. One projection might assume reopening beginning on May 15, another might assume Memorial Day weekend, and so on. Different levels of activity could be captured by setting the effective R0 to the value estimated at different times in the past.
The IDM models from that decisive moment in mid-April made it clear that re-opening before May 15 would not be advisable. Re-opening at a later date might be OK so long as activities of daily life and the number of human contacts people have each day or week did not increase to pre-pandemic normal levels.
What increases in activity were sustainable and what could we do to keep a lid on R0? Could we re-open restaurants and bars? What size gatherings could be allowed? How about schools?
More complicated questions such as these call for a different type of model.
Agent-based models are more realistic models that simulate individuals within entire populations, interacting in home, work, and community settings. These models are driven by huge databases that describe population mobility and contact patterns, often gathered from GPS-enabled smartphone devices. With this information, modelers can tweak activity and contact in ways that are much more detailed than the SEIR models.
In principle, agent-based models can overcome a key deficiency of compartmental models – what we refer to as their mass action nature. Mass action means exactly that – compartmental models generally do not capture the heterogeneity in population contact and behavior – the superspreading events like choir practices, meat packing plants, long-term care facilities and large parties. They most often smooth everything out to one average rate of transmission. In principle, agent-based models can do better by zeroing in more clearly on the links between our behaviors and viral spread.
Agent-based models are rare because they are so data- and labor intensive to build. The IDM’s COVASIM is an agent-based model that has been deployed to explore questions about how much contact tracing might be needed to keep the lid on things in King County, home of Seattle and about 2.2 million people in Western Washington. COVASIM is now being harnessed to address school reopenings.
One thing is already clear: school reopenings must be considered in the context of the ambient level of infection in the community. If the virus is not firmly under control in the surrounding community — with R0 confidently driven down to a safe level — we can’t even begin to think about reopening schools.
But there are many things that agent-based models can’t do. They don’t have a detailed map of the land; they don’t capture all the meat-packing plants, long-term care facilities or fraternity houses that are potential hotspots. They don’t tell us whether we should open restaurants at 25% or 50% capacity or at all. They don’t tell us about behavior in bars change as the night goes on. They don’t provide some of this fine-grained detail down at the local level, complete with straight lines between cause and effect, that many officials would like to have at their fingertips when making policy decisions.
This is where I pause and ask myself: Do we really need models?
At an individual level, my take is – no, not like we did in the early days of the outbreak. We don’t need models to make the decisions that will help to keep us safe and keep infections in our community under control. There are basically two words here for those who can, supported by tons of virology data, epidemiologic data, and stories from around the world: NO GATHERING.
That means no getting together with others outside of your household for any length of time without facemasks and without distance. Every online search for “COVID outbreak at _,” – fill in the blank – church service, choir practice, extended family gathering, wedding, funeral, restaurant or bars, will turn up tons of stories. Try it.
We know what causes the virus to spread – being indoors in close contact with people over a sustained period of time. We need to avoid these opportunities to get sick or make others sick. And we need to find ways to protect those who can’t avoid them: our essential employees, our food processing workers, our supermarket clerks, our healthcare teams including their non-medical support staff, our undertakers, our dentists and dental hygienists, and our bus drivers. It’s a no-brainer. We don’t need models. The global experience is our guide at this point, more than four months after we became aware of the COVID-19 in the US.
But at a policy level, models are still important despite their limitations.
Without models, we end up with policies that move too fast and are guaranteed to increase the effective R0 way beyond sustainable levels. Reopening policies that permit gathering in restaurants, bars and religious services also send a signal that we are out of the woods, and can give people a false sense of security to organize their own large private events and get togethers that would only add to the danger.
With models, we can hope to develop evidence to support policies that may be unpopular, such as mandating face masks indoors, closing bars, or disallowing religious services. We can project how many lives a statewide mask mandate might save. We can quantify the size of gatherings that can be sustained, rather than get by on guesswork. We can zero in on the ambient effective R0 that will allow schools to reopen and work towards achieving it and sustaining it.
In the end, we do need models. But we also have enough data in hand to know what to do. We must stop looking for an authority and take responsibility. If we don’t, en masse, then we don’t need models to tell us it’s going to get a whole lot worse in the coming weeks and months.