Understanding The Ideology Of Digital Transformation
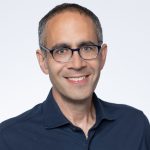
David Shaywitz
The phrase resounding in corporations these days is “digital transformation.”
What does that really mean?
According to proponents, digital transformation reflects the assertion that in order to remain competitive in the modern era, organizations need to radically rethink their approach to how they collect, manage, and analyze information.
Change is clearly afoot, but the ideology informing this hasn’t been entirely clear, beyond the vague sense that it seems to be driven by an energized alliance of technology and management consultants.
Recently, on the recommendation of a former colleague (DNAnexus CEO Dick Daly), I finally got my hands on what feels like the sourcebook for digital transformation, or at least a clear, contemporary expression of what digital transformation is and why consultants are pushing it.
The 2019 book – appropriately entitled Digital Transformation – is written by Tom Siebel. He’s a billionaire tech entrepreneur who has spent his career developing enterprise technology, and is currently the CEO of c3.ai, a firm that (besides sponsoring NPR), provides enterprise AI. That puts him in position to both support and benefit from companies undergoing digital transformation.
So of course it’s easy to dismiss Siebel’s book for being exactly what it is – an elaborate white paper that seeks to create a burning platform, motivating executives to urgently adopt the the sort of changes that would clearly benefit Siebel’s business. (Proceeds from the book itself apparently go to charity, according to the jacket cover.)
However, it would be a mistake to reflexively dismiss the book as a self-serving exercise. Much of Digital Transformation rings true, and resonates with so much I’ve seen and heard in multiple organizations. It feels like an extremely relevant and timely read, written by someone who understands both business and technology, and speaks to issues that every organization I know is trying to manage.
Having said that, there’s very little in the book specifically about biopharma and healthcare, and much of what’s there seems unlikely to resonate with many domain experts. I suspect this disconnect reflects the lack of progress to date in these industries, combined with Siebel’s limited first-hand experience here.
The Burning Platform
First, the burning platform. According to Siebel, the intersection of four significant “technology vectors” – cloud computing, big data, artificial intelligence (AI), and the internet of things (IOT) – is driving such profound change in the environment in which organizations live that businesses face as “mass extinction event.” Companies are fading from relevance at unprecedented rates, CEO tenures are growing ever shorter, and private equity firms are piling up increasing amounts of dry power, ready to pounce on corporations perceived as laggards. Companies, argues Siebel, “are facing a life-or-death situation.”
In case this is still too subtle, Siebel writes, in a chapter on AI in the defense industry:
“AI will fundamentally determine the fate of the planet. This is a category of technology unlike any that preceded it, uniquely able to harness vast amounts of data unfathomable to the human mind to drive precise, real-time decision-making for virtually any task.” He adds that as the US and China engage “in a war for AI leadership,” the “fate of the world hangs in the balance.”
Of course, motivating change requires not just a reason to change (unambiguously provided here), but also a direction forward – in this case drawing inspiration from a transformational event in the earth’s history:
“Recall how the Great Oxidation Event’s cyanobacteria and oxygen resulted in new processes of oxygenic respiration. Today, cloud computing, big data, IoT, and AI are coming together to form new processes, too. Every mass extinction is a new beginning. Changing a core competency means removing and revolutionizing key corporate body parts. That’s what digital transformation demands.”
Siebel reviews the distinction noted by organization theorist (and author of Crossing the Chasm) Geoffrey Moore. Moore draws the distinction between a company’s core – what creates differentiation, e.g. Tiger Woods’s golf skill – and a company’s context – everything else, such as marketing. Thus, Woods may make a lot of money from marketing, but his core, his competitive advantage, is how he plays golf. At a level of simplification, says Siebel, core is often viewed as intellectual property, while context is often outsourced. Siebel argues that many companies have digitized their context competencies, but not their core – but that is exactly what’s required, he argues.
Such change constitutes a difficult process that often requires a strenuous re-thinking of the underlying business, creating “something faster, strong, and more efficient that can do the same job in a totally different way – or do entirely new things.”
The key opportunity, Siebel argues, is for companies to “use data to reinvent their business models.” The change required is profound – and, argues Siebel, it must be driven by the CEO, rather than by the chief information officer or anyone else.
According to Siebel, “implementing a digital transformation agenda means your organization will build, deploy, and operate dozens, perhaps hundreds or even thousands, of AI and IoT applications across all aspects of your organization, from human resources and customer relationships to financial processes, product design, maintenance, and supply chain operations. No operation will be untouched.”
The Four Technology Vectors
The four technologies shaping our future, according to Siebel, are cloud computing, big data, AI, and IoT. In a nutshell:
- Cloud computing provides convenient access for all businesses to essentially unlimited compute and storage, with major providers (Amazon Web Services [AWS], Microsoft’s Azure, Google Cloud) routinely providing robust security and continuously improving resources, characterized by the “rapid innovation of microservices” such as Google’s TensorFlow designed to “accelerate machine learning.” Adds Siebel, “not a week goes by without another announcement of yet another useful microservice” from a leading cloud vendor.
- Big data refers not so much to the raw quantity of data collected, managed, and analyzed, but really to the mindset towards data – the idea of collecting everything, versus just a sample; in other words, “complete data.” As Siebel nicely puts it, the “significance of the big data phenomenon is less about the size of the data set we are addressing than the completeness of the data set and the absence of sampling error.“ (Whether this is achievable, or impossibly hindered by either technical or social/political barriers, is a topic we’ll return to shortly.)
- AI involves computers tackling problems that normally require human intelligence. Machine learning (ML) is a subset of AI that involves teaching computers to learn from experience, rather than pre-defined rules. ML might be used to train an algorithm to assess whether an image has a cat or not; this process tends to require a lot of “feature engineering,” where data scientists and domain experts determine what are the key parameters to feed into the algorithm to help it become more accurate. Deep learning is a subset of ML where “the important features are not predefined by data scientists but instead learned by the algorithm.”
- IoT is the idea of connecting “any device equipped with adequate processing and communication capability to the internet, so it can send and receive data” – essentially, the “convergence and control of physical infrastructure by computers.”
These four technologies, Siebel observes, present “powerful new capabilities and possibilities. But they also create significant new challenges and complexities for organizations, particularly in pulling them together into a cohesive technology platform.” Not surprisingly, “many organizations struggle to develop and deploy AI and IoT applications and scale and consequently never progress beyond experiments and prototypes.”
Digital Transformation: Implications For Healthcare
Digital transformation, Siebel asserts, will “improve human life.” How? Though “very early disease detection and diagnosis, genome-specific preventive care, extremely precise surgeries performed with the help of robots, on-demand and digital health care, AI-assisted diagnoses, and dramatically reduced costs of care.”
Skeptical about whether healthcare – characterized famously by Esther Dyson as “calcified hairball” system of care – can be disrupted? Siebel’s rejoinder (cited multiple times) is that in January 2018, when Amazon, Berkshire Hathaway and JP Morgan Chase announced their intention to enter the market, “$30B of market capitalization was erased from the 10 largest U.S. healthcare companies” in a single day of trading. While these stocks recovered almost immediately, the market reaction, according to Siebel, emphasizes the industry’s vulnerability.
Cloud
While Siebel doesn’t offer specific examples of healthcare and the cloud, he shares his view that executives who less than a decade ago proclaimed “our data will never reside in the public cloud” – something I personally heard from a number of healthcare leaders even five years ago – are now delivering a very different message that is “equally clear and exclamatory: ‘…we have a cloud-first strategy. All new applications are being deployed in the cloud. Existing applications will be migrating to the cloud. But understand, we have a multi-cloud strategy [to avoiding vendor lock-in].’” While healthcare was among the last to the cloud, it seems many health organizations have finally gotten the message.
Big Data
Siebel highlights the potential value to precision medicine of being able to access “the medical histories and genome sequences of the U.S. population.” His point, it seems, is that “big data” thinking enable us to contemplate considering the data of each person, rather than generalizing from a sampling of people. Actually acquiring anything approaching such a complete data collection, of course, is a non-trivial real-world challenge, as most in biopharma and healthcare recognize — and often lament. In biopharma, technical (as well as financial) limitations may stymie efforts to collect and subsequently analyze all possible information in human beings and other complex biological systems.
AI
Siebel is clearly taken by the potential of AI in healthcare, while acknowledging “the health care industry is just starting to unlock value from AI. Significant opportunities exist for health care companies to use machine learning to improve patient outcomes, predict chronic diseases, prevent addiction to opioids and other drugs, and improve disease coding accuracy.”
He suggests machine learning algorithms can be used “to predict the likelihood someone will have a heart attack, based on medical records and other data inputs – age, gender, occupation, geography, diet, exercise, ethnicity, family history, healthy history, and so on – for hundreds of thousands of patients who have suffered heart attacks and millions who have not.” (This again assumes it’s possible to get one’s hands on enough of the relevant data to train the algorithm. That’s profoundly difficult in today’s environment, beset by the problems of data interoperability, patient data hoarding by hospitals, proprietary EHRs that can’t/won’t talk substantively to each other, and an ecosystem of stakeholders who aren’t inclined to share data.)
Applications for deep learning in healthcare, according to Siebel, include “medical image diagnostics, automated drug discovery, disease prediction, bone-specific medical protocols, preventive medicine,” though additional detail isn’t provided.
Perhaps especially relevant to medical practitioners, Siebel suggests that “the ability to apply AI to all the data in a dataset” means that “there is no longer the need for an expert hypothesis of an event’s cause. Instead the AI algorithm is able to learn the behavior of complex systems directly from data generated by those systems….The implications are significant…An experienced physician [in Siebel’s future world, presumably] is no longer required to predict the onset of diabetes in a patient.” Instead, this information can be gleaned “from data by the computer – more quickly and with much greater accuracy.” I am aware of glimmers of progress in this area, which has been discussed for over a decade.
IOT
Siebel suggests that connected devices “give doctors the opportunity to track patient health remotely in order to improve health outcomes and reduce costs. By harnessing all these data, IoT supports doctors in predicting risk factors for their patients.” He notes that pacemakers “can be read remotely and can issue alarms to doctors and patients, warning if a heartbeat is irregular.” He reports that the “wearable industry has given people the ability to easily track all sorts of health-related metrics.” Combining wearable information with clinical data, he observes, “can create a holistic view of the patient, allowing doctors to deliver better care.”
So far so good, right? But Siebel isn’t through. “Soon,” he contends, “humans will have tens or hundreds of ultra-low-power computer wearables and implants continuously monitoring and regulating blood chemistry, blood pressure, pulse, temperature, and other metabolic signals. These devices will be able to connect via the internet to cloud-based services – such as medical diagnostic services – but will also have sufficient local computing and AI capabilities to collect and analyze data and make real-time decisions.”
I’m not sure even most quantified selfers would embrace such a future; if anything, this vision seems to evoke folk singer-songwriter Arlo Guthrie’s memorable description of his military physical examination during the Vietnam War era, where “they was inspecting, injecting every single part of me, and they was leaving no part untouched.”
Siebel points out that large sets of IoT-generated data can “uncover insights and make predictions,” such as using “AI predictive analytics to find potential barriers to medication treatment and identify potential contraindications. This gives doctors the tools to more effectively support patients, improve outcomes, reduce relapse, and enhance quality of life.”
He continues, “Imagine pill bottles that track adherence to prescribed medications, alerting doctors and users when patients fail or forget to take their medication. Also in development are smart pills that can transmit information on vital signs after being ingested.” (I’m sure Otsuka can envision this quite clearly….)
Finally, if you’re not creeped out yet by this degree of monitoring, Siebel, in pointing out that “data generated everywhere through an organization can have value,” reports that today, “Insurance companies…work with mining and hospitality companies to add sensors to their workforces in order to detect anomalous physical movements that could, in turn, help predict worker injuries and avoid claims.”
In this vision of digital transformation the future of both work and health apparently involves, and certainly aspires to, ever-more detailed monitoring and assessment of every facet of existence. It’s a vision that sounds like total, continuous surveillance.
Not only is this approach exceedingly, absurdly, invasive, but it may not even deliver the cost-savings Siebel repeatedly promises, as my Tech Tonics co-host Lisa Suennen points out:
“Tech can only reduce healthcare costs when financial interests are aligned,” Suennen reminds us. “Digital products for early diagnosis can just as easily lead to excessive testing and treatment when the impetus is to increase utilization (which increases cost). It is true that technology such as AI and robotics have the potential to lead to cost-reductions in healthcare, but there is far more to it than technology alone. As with all technology, it is a tool, not a solution. When the solution one is solving for is to increase revenue, the tool can work just as well in the hands of someone who benefits from increased cost.”
In short, Siebel’s perspective on the ideal future state of healthcare feels both dissonant (I’m not sure most people wanted to be constantly monitored for failure, like IoT-enabled equipment constantly surveyed by a technician) and elusive (based on the challenges of gathering even modest amounts of integrated health data in one place); moreover, as Suennen argues, it may not even deliver the beneficial economics Siebel anticipates.
Digital Transformation: Implications For Organizational Change
In contrast, Siebel’s observations on barriers for organizations contemplating digital transformations seem thoughtful and highly relevant, particularly regarding data, people, and prioritization.
Data
Siebel premise is that “successful digital transformation hinges critically on an organization’s ability to extract value from big data,” and a key initial challenge is how to organize all the data in the first place. But the good news, argues Siebel, is that large established companies are starting on their journey with one key advantage: they’re already sitting on a lot of data (though unlocking value from these data might be another story).
Argues Siebel, “incumbent organizations have a major advantage over startups and new entrants from other sectors. Incumbents have already amassed a large amount of historical data, and their sizable customer bases and scales of operations are ongoing sources of new data.”
He acknowledges, “Of course, there remain the considerable challenges of accessing, unifying, and extracting value from all these data. But incumbents begin with a significant head start.”
The challenge is what to do with all these legacy data. The temptation is to put it all in one place, a so-called data lake or data swamp. Not smart, Siebel argues.
“Storing large amounts of disparate data by putting it all in one infrastructure location does not reduce data complexity any more than letting data sit in siloed enterprise systems. For AI applications to extract value from disparate data sets typical requires significant manipulation such as normalizing and deduplicating data,” Siebel observes, adding “the key big data challenge “is to represent all existing data as a unified, federated image.”
People
To operate in this brave new world requires comfort with both the data and the emerging ways of thinking about data. Writes Siebel: “Generating value requires individuals in the enterprise who are able to understand all these data, comprehend the IT infrastructure used to support these data, and then relate the data sets to business cases and value drivers. The resulting complexity is substantial.”
Interestingly, and (based on my experiences over the years) perceptively, Siebel calls out what he describes as a common mistake: overconfident CIO’s who mistakenly (in his view) believe they can assemble the required data and analytics structures on their own, DIY-style. Siebel says he’s observed this sort of misplaced confidence from the time he was at Oracle, selling enterprise application software, and realized that their biggest barrier wasn’t competitors, but the CIO who wanted to solve the problem DIY, and, according to Siebel, generally failed. (Again: take with a grain of salt, given Siebel’s obvious interest in selling enterprise software.)
Siebel notes that companies obviously require more than just data experts – they also need “translators” who “can bridge the divide between AI practitioners and the business. [Such translators] understand enough about management to guide and harness AI talent effectively, and they understand enough about AI to ensure algorithms are properly integrated into business practices.”
But what it seems like companies need most of all, according to Siebel, is a ton of consultants – or as he politely refers to them, partners: “In a digitally transforming world,” he says, “partners play a bigger role than in the past.” He explicitly writes companies should involve management consultants for strategy, software partners for technology, professional services to build apps, and change management partners to get people to use to the new tech. Suddenly, you can begin to understand why “digital transformation” is so broadly embraced: it’s like an Oprah giveaway but for consultants (YOU get more consulting work, and YOU get more consulting work, and YOU get more consulting work…).
Priorities
While Siebel’s advice regarding consultants feels a bit self-serving, his advice about prioritization seems spot-on, and certainly aligns with what I’ve been suggesting, as well as with the advice that experts I admire, like Jim Manzi, seem to be offering.
Above all, says Siebel, focus on business needs, not abstract, highfalutin aims. “Work incrementally to get wins and capture business value,” he emphasizes. Much as Vizzini, in The Princess Bride, famously advises “never get involved in a land war in Asia,” Siebel’s counsels (perhaps for similar reasons) “Do not get enmeshed in endless and complicated approaches to unify data. Build use cases that generate measurable economic benefit first and solve the IT challenges later.” He also suggests adopting a “phased approach to projects,” seeking opportunities to “deliver demonstrable ROI one step at a time, in less than a year.”
He notes that “Many organizations get hopelessly mired in complex ‘data lake’ projects that drag on for years at great expense and yield little or no value.” There are many examples, he says, of companies wasting big money on such projects. He cites multiple examples of companies wasting years with “outside consultants to build a unified data model, only to see no results at all.”
While the use-case first approach “may sound like heresy to a CIO,” Siebel says that this approach “allows for focus on the value drivers.” The emphasis of a digital transformation strategy, he argues, should be “creating and capturing economic value.” Fulfilling this value mandate requires thoughtful roadmap and prioritization, “identifying and prioritizing functions or units that can benefit most from transformation.”
Finally, counsels Siebel, use common sense. “If a project does not seem to make sense, it’s because it doesn’t make sense. If it appears incomprehensible, it is likely impossible. If you do not personally understand it, don’t do it.”
Figuring out how to apply this admonition to use common sense to areas like healthcare and biopharma – where the benefits touted by technologists often don’t seem sensible (as both Derek Lowe and I observed this week), but in some cases, could be truly transformative — represents both the challenge and the opportunity of our moment.