How to Navigate the Blizzard of COVID-19 Antibody Studies
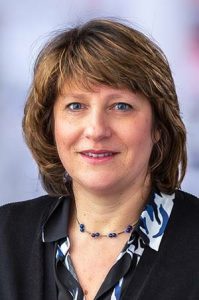
Ruth Etzioni, Full Member, Division of Public Health Sciences, Fred Hutch Cancer Center
What is the single thing you wish you knew about COVID-19?
Here is what I wonder about most.
How many of us have had it already without knowing it – either because we did not have symptoms, or because we thought it was something else, or because we thought it might be COVID-19 but we could not get tested?
My interest in this matter is both personal and societal.
Personal, because three days after I came back to Seattle from London Jan. 27 on a packed Delta flight, I came down with a major chest cold.
During the day, I was OK. Things went downhill as evening came on, and I suffered with coughing fits and fevers through each night. This went on for 12 miserable days.
Was it COVID-19? If, like me, you have searched for “did I have the coronavirus,” it’s clear that plenty of people are wondering the same thing.
Having recovered from that nasty cold, and having gotten a negative test earlier this month, my interest is now more societal in nature. Societal because we need to know how many people have had COVID-19 to understand the real reach of the virus, its true contagiousness. We must be able to identify who is immune in order to carefully plan our exit from lockdown.
So I have been drawn to three new studies that report the results of serology tests conducted on persons without a prior COVID-19 diagnosis – in the City of Chelsea, Massachusetts; in Gangelt, a municipality in the Heinsberg district of northwestern Germany; and in California’s Santa Clara County.
All three of these places are known virus hotspots. Chelsea has the highest rate of infections in Massachusetts, Gangelt had an outbreak following a superspreading carnival event in mid-February, and Santa Clara County (the home of Silicon Valley) became an epicenter in early March.
Their results are strikingly different. In Chelsea, 33% of individuals tested were positive, in Gangelt 15%, and in Santa Clara 1.5%.
Like all diagnostic tests, antibody tests can be wrong in two ways. First, they may produce a false negative – you have had the virus but the test doesn’t detect it. Or they may produce a false positive – you haven’t had the virus, but the test says you have. The false negative and false positive error rates define the test accuracy. There are concerns that the majority of the tests currently being marketed (more than 95 to date) do not make the grade.
Because antibody tests are not perfect, we can’t simply use the reported fraction testing positive to estimate how many people have really had the virus. If the false negative rate is high, that means we have likely undercounted; if the false positive rate is high, we have overcounted. We can use these error rates to fix the fraction found to have antibodies so that we get the right result. Let’s see how this worked in Chelsea, Gangelt and Santa Clara.
The Chelsea study used a rapid test to look for antibodies in the blood from BioMedomics. The company says its false positive and false negative error rates are around 10%. When the researchers accounted for these error rates, they found that although 33% of cases tested positive, the actual number with antibodies was lower – around 30%. That’s still a startlingly high prevalence, much higher than the 2 percent officially diagnosed via the standard RT-PCR diagnostic test. It tells us that in Chelsea, there are 15 cases that we don’t know about for each case already reported.
The Gangelt study did not specify which antibody test they used. Researchers there reported a low false positive error rate (less than 1%) but did not provide a false negative rate. More clarity on the product being used, and its error rate, is needed to evaluate the results. But as things stand, the number of people estimated to have been infected in Gangelt is between 15-17 percent. (That assumes false negative rates in the range of 5-15%.) In the Heinsberg region, in which Gangelt is located, almost 4% of the population was officially diagnosed via the RT-PCR-based diagnostic test. This implies that in Gangelt, there are roughly 4 cases we don’t know about for each diagnosed case.
And in Santa Clara? This study used a test from Minnesota’s Premier Biotech. Based on the reported performance of their test (and some independent validation at Stanford University), the authors did the math. They concluded that 2.5% had previously been infected. Based on an even tinier fraction of the population with a confirmed diagnosis, the researchers concluded that there are at least 50 cases that we don’t know about per diagnosed case in Santa Clara County.
So. 4 to 1, 15 to 1, and 50 to 1.
That’s a lot of variation in the balance between cases we know about and cases we don’t. What gives?
One answer is that the estimates may be incorrect; for example, a chorus of criticism has surfaced about the Santa Clara study. Critics argue that the method of sampling (via Facebook ads) preferentially enrolled subjects who had had symptoms, and the study likely understated the false positive rate, both of which could have inflated the estimated prevalence of antibody-positive individuals.
But a study done in Los Angeles just reported very similar results, suggesting as many as 55 undiagnosed cases per known case of COVID-19 there. This study was better designed because it used a more random sampling method, but it used the same serology test as the Santa Clara study. Therefore, it doesn’t address the false positive rate issue. This is important, because if you don’t get the error rates right, you can’t properly fix the reported fraction testing positive to figure out the real prevalence of antibody positivity.
Another answer, one that bears serious consideration, is that we’re oversimplifying. The notion that there is a single number – of silent, undiagnosed COVID-19 cases for each reported diagnosis – is just too basic. Clearly, it depends on where you live.
Going deeper, we can look to three key factors that drive the balance between cases that we know about and those that we don’t: testing, timing, and demographics.
Germany is famous for its massive testing response to COVID-19, particularly in the region of Heinsberg, the location of one of the country’s largest outbreaks. The opposite was true in Santa Clara, where testing got off to a slow start. By Apr. 1, half the number of cases per capita had been diagnosed in Santa Clara County compared with Washington State, despite both being among the locations with the earliest recorded US cases of the virus, in late January.
More testing leads to more people being diagnosed. This means more cases that we know about, and fewer that we don’t, and ultimately, less of an imbalance between undiagnosed and diagnosed cases. With much less testing, the number of known cases drops, and this could explain the Santa Clara findings.
Timing is critical too: when infections took off, when testing ramped up, when social distancing and other restrictions began, when the antibody test was actually done.
Timing impacts not only the balance between diagnosed and undiagnosed cases, but the overall size of the infected population. It’s a little hard to know how much this played a role in the dramatic difference between Chelsea (30%) and Santa Clara (2.5%) but, for the record, the Bay Area’s shelter-in-place order came on Mar. 16 when there had been just two reported deaths in Santa Clara County. Massachusetts came later, ordering the shutdown of non-essential businesses on Mar. 24 after 9 COVID-19 deaths in the state.
The size of the infected population and the balance of known infections don’t just depend on testing and timing. Demographics also matter.
According to an article in the Boston Globe, about 65% of the residents of Chelsea are Latino, and only 21% are white. Many live in crowded conditions, where it is hard to isolate. And many work in service industries and health-related fields, so they can’t shelter at home.
In contrast, only about 25% of the residents of Santa Clara County are Latino and 51% are white. Many work in the tech industry and are able to work from home. Population density in Santa Clara County is an order of magnitude lower: around 1,500 versus 18,000 per square mile in Chelsea. So, contact rates that drive infectious transmission are likely far higher in Chelsea than in Santa Clara County.
These factors are some you might want to think about when interpreting the impending blizzard of antibody studies, which I am guessing will continue to show highly variable results.
The take-home message though is this. The balance of undiagnosed to diagnosed COVID-19 cases is not just about biology. It depends on many other things. Ultimately, while 50 to 1 seems high to me, these studies make me think that the size of the undiagnosed population in the US is a lot bigger and the virus a lot more contagious than we imagined. State and national policy makers can and must use this knowledge to shape our strategy for carefully exiting from COVID-19 lockdown.