Why COVID-19 Can’t Be Directly Compared With the Flu
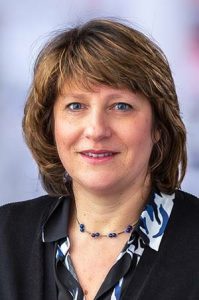
Ruth Etzioni, Full Member, Division of Public Health Sciences, Fred Hutch Cancer Center
A few years ago, I was preparing for a live radio interview about prostate cancer screening, my main area of research for the past 20 years.
As a statistician focused on getting the numbers right, I disagreed strongly with the new national recommendation from an influential task force that guides practice and reimbursement. Members of that task force argued against routine population-wide screening for this most common cancer in men, saying essentially that there wasn’t any discernable benefit.
In the last few seconds before we went on air, the host whispered to me, “just don’t talk about numbers please!”
The problem was not with the numbers, I told him. It was with a faulty comparison.
To support their recommendation, the panel had relied heavily on data from a national, randomized clinical trial that showed similar numbers of prostate cancer deaths among men screened and not screened.
This was the obvious comparison to make, the one mandated by established research principles, and the one for which the data was readily available.
But it was the wrong comparison.
It turned out that the national trial had started late, after prostate cancer screening was already commonplace. It was not a comparison of screening versus no screening; rather, the trial compared a group that had been screened against a group that had been screened almost as much.
As we face critical decisions about whether and how to resume our daily lives in the midst of the COVID-19 pandemic, we are being tempted into making the wrong comparisons again. This may be dangerous for individuals, but it could be catastrophic for national and state policies.
Comparison 1: Number of lives lost (so far) because of COVID-19 versus the seasonal flu. Even as the numbers change, the current projections of COVID-19 deaths (estimated at approximately 60,000 as of yesterday) are being compared with the reported deaths from flu in the current 2019-2020 season.
The CDC’s latest estimates are that 24,000 to 62,000 US deaths can be attributable to the flu in the season stretching from Oct. 1, 2019 to Apr. 4, 2020.
If all you did was look at the current COVID-19 projection, and compared it with the upper-end worst estimate of this year’s flu season, you could say they are in the same ballpark.
But this is a faulty comparison. The deaths from these two illnesses are occurring under completely different circumstances.
The COVID-19 deaths are in the presence of a nearly national shutdown, with school and university closures and widespread practicing of social distancing. The flu deaths from last season occurred largely in the absence of all of these interventions. What would the number of flu deaths be if every winter we locked down early in the season, in October or November, like we are doing now for COVID-19? That is the question to ask.
The takeaway here is that if we want to compare COVID-19 deaths with flu deaths, we need to do so under the same prevailing policies. We could compare reported COVID-19 deaths with the flu deaths expected under current work/school closures and social distancing policies. Alternatively, we could compare COVID-19 deaths expected in the absence of any of these interventions with the flu deaths actually reported. Either way, we would not have hard data from past experience to work with. We would have to resort to modeling. So let’s talk about models.
Comparison 2: Numbers of COVID-19 deaths reported or projected today versus those projected by earlier models.
Much has been made recently of updated projections of COVID-19 deaths that are considerably lower that what was predicted based on earlier model reports. A highly influential model from Imperial College London, early in the outbreak response, predicted upwards of 2.2 million deaths in the United States if policymakers did nothing to mitigate the spread of the virus. Subsequently, a US-developed model from the Institute for Health Metrics and Evaluation (IHME) had projected between 100,000 and 240,000 deaths, taking into account the ongoing mitigation policies. This model is the one most frequently cited by the Trump administration, but its predictions have changed considerably as the pandemic has evolved, and now stand at approximately 60,000 COVID-19 deaths in the US by the end of August.
Some people are using the changing numbers to argue that our efforts to mitigate the pandemic have been wildly successful. Some are arguing that the numbers are proof that we overreacted and intervened too dramatically. This comparison of current model projections with earlier ones is even fueling calls to rapidly unwind social distancing policies and reopen the economy.
This is the wrong comparison to be driving any decisions about relaxing the current restrictions.
We can’t compare current observations or predictions from the model with earlier predictions as if they were set in stone. This gives far too much credibility to earlier versions of a model that could not have been expected to provide accurate predictions in the first weeks of COVID-19 spread in the US.
Originally, the IHME model was constructed to replicate the increasing and subsequently declining pattern of deaths officially reported by China. To port this model to the US setting, the modelers had to compress or stretch the China curve to match the accumulating US data. In the early days of the pandemic in the US in early March, the IHME modelers had about 70 days of data from China to work with. But there was very little data to tailor the model to the US experience. The current version of the model is privy to a more complete, but still evolving, picture of COVID-19 in the US; it also incorporates data from Europe. No wonder the results keep changing.
The takeaway here is that comparing different versions of a model to learn about facts on the ground is a mistake. If we want to discern the likely impact of mitigating policies, we need to be using the same version of the model to do the comparison. This could help us to understand how well current polices might be working and what to expect if in their absence. We should not lose sight of how limited models are and how uncertain their predictions can be, but we should at least use and interpret them in a defensible way.
And what of prostate cancer screening?
Ultimately, the question of prostate screening benefit was put to rest by a trial conducted in Europe before screening became widespread there, along with a set of related modeling studies based on all of the accumulated data. My team published that work in 2017, confirming that screening saves lives.
The general lesson here is not about models, or cancer, or even COVID-19. It is about thinking clearly when using statistics to make life-or-death decisions. The numbers do matter, but first you have to get the comparison straight.