Can Novartis Digitally Transform Clinical Development?
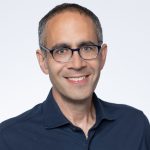
David Shaywitz
In 2018, Dr. Vas Narasimhan, newly-installed as CEO, told the Wall Street Journal he saw Novartis as “a focused medicines company that’s powered by data science and digital technologies.”
Since then, Novartis has tried to grow into this ambition, embracing the concept of digital transformation perhaps more conspicuously than any other large pharma.
It’s not easy, as Narasimhan himself acknowledged one year in, when he discussed with refreshing candor his early efforts, and shared some of the hurdles and disappointments he encountered.
Now, Narasimhan, together with colleague Luca Finelli (a 15-year veteran of the company, with experience in modeling and analytics), have published a paper in Clinical Pharmacology and Therapeutics outlining Novartis’s initial effort to apply their data science mindset to global clinical development, an area they saw as a particularly attractive opportunity. (Narasimhan isn’t alone; at a recent NewYorkBIO webinar, J&J’s newly-appointed Chief Data Science Officer, Najat Khan, similarly emphasized the value of focusing on clinical development.)
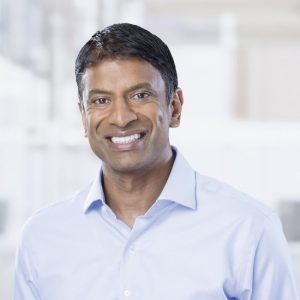
Vas Narasimhan, CEO, Novartis
The choice isn’t — or shouldn’t be — a particular surprise: clinical development represents a huge slug of pharma’s total R&D expenditure, the operational complexity seems ripe for digital refinement, and the effect of any improvement should be appreciated relatively quickly by the organization; these contrast with potential digital upgrades on the research side, which could take far longer to prove themselves.
Novartis also made a deliberate (and prudent) decision at the outset to focus on operational data, specifically, rather than patient-data; the authors point out that because such data are “fundamentally of non-human origin,” they aren’t subject to the various data protection and privacy regulations that can introduce additional layers of complexity to data analysis – especially when such data are sourced globally.
The basic idea was to turn decades of clinical trial operational experience into something that could be represented digitally and understood broadly, so that lessons could be extracted and applied going forward. Historically (and in fact, not so historically, as this is generally what happens today in most companies), “summarizing the status of just one study” requires “a strenuous review of many spreadsheets to obtain first the country, then the regional, and finally the global overview.” These data, the authors explain, come “from different systems through manual, one-time extractions, at different time points, and often following different assumption and rules.”
Novartis’s aspiration was to turn “tedious, time-consuming tasks that can often take days or even weeks to complete manually” into something more enlightened and efficient, using the rich operational data that already exist.
For example, the authors say, “historical trial management data indicate exactly how successful each medical center was at recruiting patients, for each investigational drug, past data shows how well clinical drug supply followed the initial plans, and finance data can tell the entire spend story behind a development program.”
In other words: armed with the requisite data, the company should be able to figure out how to select better clinical trial sites, improve the management of their drug supply, and estimate more accurately the true cost of developing a proposed medicine.
They aspired to build a data and analytics platform to achieve this goal (and were aided, apparently, by a McKinsey-owned analytics company called QuantumBlack). The initial challenge they faced was wrangling the relevant operational data. This was an especially difficult task since these data tended to be “locked into silos,” residing “in multiple internal dedicated system,” from which “extraction…is difficult, and requires time-consuming manual efforts that only IT personnel with special access rights can execute.” As they aptly summarize, the “operational data landscapes emerge as very fragmented.”
A key issue, they discovered, was that most operational data were “not generated with the intention of doing analytics. It typically just supports temporary tasks and transitions.” Thus, “one of the monumental efforts…has been ‘connecting’ and ‘curating’ that data so that it could be seamlessly utilized across the operational landscape. This involved re-processing decades of data from a variety of systems, each with other formats and identifiers – getting the data ready for analytics was at the foundation for our digital transformation.”
As they wryly observe – referencing a previous piece I wrote – “the amount of work required to bring all the data from different sources together to an analyzable format is largely underestimated in the public excitement about AI and machine learning.”
Novartis’s vision for this platform – called “Nerve Live” – encompassed multiple areas, from clinical trial operations to financial modeling, each envisioned as essentially an “app,” intended to deliver “intuitive user experiences, elegantly combining advanced analytics with well thought out application design and the right data context.”
The first app developed was an AI application designed to predict the “timing of ‘end of enrollment’ across > 300 studies simultaneous and through intuitive visualizations, allowed for root cause analysis down to single site activities…” According to the authors, a total of eight apps have now been developed, tackling topics from resource requirements to cost forecasts to patient enrollment predictions.
Naturally (like AstraZeneca, discussed here), Novartis also has a large “mission-control”-like room with a trial dashboard, the “SENSE Insights Center,” of which they seem especially proud.
I was especially interested in the discussion of “enablers” – essentially “key success factors” (assuming you accept the premise that this effort has been successful – we’ll come back to this). In addition to the inevitable citation of “senior leadership sponsorship,” they highlight the role of team composition and of the iterative approach they used, which I suspect were both critically important.
The key individual drivers of this process seemed to be the “product owners,” charged with leading all aspects of product development.
While this model emulates the approach used by both tech companies and pharma clinical development teams, the secret sauce seems to be the traits and experiences required of these product owners, a “mixed set of core competencies” that “are not easy to find in a single individual.”
These include expertise in data science, business, user needs, learning agility, project management, design thinking, and experience with what’s known as “Agile” product development.
Oh, and one more thing: “owners also need to know well how to navigate our IT process governance….” I suspect this last item — figuring out how to productively engage with incumbent IT — was particularly important.
Another critical aspect seems to have been the iterative approach, where the initial work seemed to focus on just one app, with the idea that palpable success with this could enhance subsequent buy-in.
Predictably, the most difficult challenges, according to the authors, involved not technology but rather “people, behaviors, and the change required for the transformation to succeed.”
Did it succeed? When they finally get to what seems like a critical question, the authors hedge – big time.
“Although we are just starting to assess systematically the benefit of the program,” they write, “initial projects indicate that productivity gains in the order of 10% are already achievable across the portfolio of activities.”
Translation: it’s not clear — yet — that the juice has been worth the squeeze.
My Takeaways:
I actually found this article to be enormously inspiring, representing what seems like a bold and ambitious vision, pursued intelligently.
I was pleased, though hardly surprised, to see the initial focus on operational data and palpable goals – two strategic elements I’ve long championed. I recognize the importance of identifying the right talent to lead these teams, though I can imagine, as Dr. Amy Abernethy, Principal Deputy Commissioner at the FDA, emphasized at a recent conference, that these qualities can and typically do reside in a group of people, and the real skill required might not be the multiple core competencies the authors aspirationally list, but rather the ability to elicit these from a well-chosen team.
That said, the critical importance of organizational savvy — alluded to discretely in the article — cannot be understated, as the work of Stanford’s Jeffrey Pfeffer has so clearly and painfully highlighted.
Finally, what of the results? If you’re a skeptic, you may look at all this, and probably say, “Surprise, surprise – another CEO shows up with his pet project, enabled by an army of consultants, lots of money is spent, lots of ink is spilt, and for what?” This is even how many critics view the efforts around precision medicine. The first rule of holes, they say, is to stop digging.
But I am encouraged. Emerging digital and data technologies offer pharma companies a critically important opportunity to do their critically important work better and, hopefully, cheaper. For incumbent companies, change is notoriously hard, and it’s always appealing to make cosmetic adjustments but to continue with business as usual as long as possible. And in fairness, it’s not always easy to know the right time to adopt a new technology and mindset; it’s possible Novartis is still too early, and the approaches available to companies in five or fifteen years will be so much better and so much cheaper that Novartis will feel foolish for choosing to be (in the context of the industry) an early adopter.
But I think Novartis is doing something very smart. By embracing this change, they are learning about the talent and competencies that future success will require, and their employees are starting to think in a way that seems crucial for the industry going forward, gaining valuable experience that is likely to be in high demand over the next decade.
I’m also not worried about the limited results apparently demonstrated to date; this is the nature of technology adoption – you need to figure out how to use it, and there’s what W. Brian Arthur has called a “mutual adaption” that’s required between technology and domain. I can envision the process Novartis is going through will ultimately lead to more efficient studies that are better for company and patients alike.
What we’re seeing is the difficult work of a much-needed industry makeover. Good on Novartis for ambitiously taking on this challenge.
(Disclosure: I do not have a personal or business relationship with Novartis.)