Closing Medicine’s Feedback Gap: Can Tech Help Integrate Clinical Care and Clinical Research?
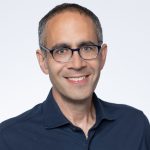
David Shaywitz
Medicine is plagued by a feedback gap, or perhaps more accurately, a feedback paradox.
On the one hand, clinicians are bombarded by feedback. Every day, there are a slew of process and billing metrics to review, providing an accounting of the volume of patients seen, and the intensity of each visit.
How thorough was the exam? What procedures may have been performed?
Even beyond the measures related to billing are an ever-growing number of measures related to guideline adherence. Doctors have an increasingly long set of questions to answer, boxes to check.
Did you ask about seatbelts use? Did you ask about home safety? Have you scheduled an eye exam for your patient with diabetes? These are often, individually, sensible and relevant considerations. In aggregate, however, they can be overwhelming and lead to guidance fatigue and ethical slippage, as Bill Gardner (here) and Drs. David Blumenthal and J. Michael McGinnis (here) have discussed, and as I recently reviewed in the context of COVID-19.
Yet for all these metrics and assessments, what’s often lacking is any sense of how the patients are actually faring – the thing that matters most.
It’s not at all clear whether all the carefully documented and billed work is leading to improved outcomes for patients. Are there approaches for some patients that are leading to better outcomes than other approaches, and which could be generalized? Are there other approaches that should be abandoned?
The heart of the problem is how most clinical research is done. On the one hand, to demonstrate, scientifically, that a particular approach (whether a novel drug or a new approach to therapy, like placing sick COVID-19 patients on their stomachs instead of their backs when they’re in the hospital) works, a clinical trial – ideally, a randomized, double-blind, placebo-controlled, to the extent possible – is performed. This is a highly choreographed exercise, where the exact characteristics of the subjects to be enrolled, the evaluations to be performed, and the criteria for success are all spelled out, clearly, in advance (or at least, ought to be). Enrolled subjects are tracked meticulously, and ultimately, you should be able to determine if the intervention was more effective than your control at achieving the pre-specified outcomes.
This is the way medical science advances – through rigorous clinical studies, and then the adoption of these results in clinical practice.
The conceit of this approach is that the results from a rigorous clinical study will be widely generalizable, meaning that the patient you’re treating will respond like the subjects in the study, and the treatment you’re providing will mirror that offered in the study.
Of course, we all know side effects can emerge over time that weren’t seen in clinical trials, and we recognize that certain groups – women and minorities in particular – have historically been underrepresented in clinical trial populations.
To the extent that you accept the basic premise that a well-run clinical trial yields results that can guide care, then it makes sense for health systems to impose processes that try to ensure patients with a particular condition receive the treatment indicated by clinical trials.
This approach has given rise to the idea of “disease pathways,” that provide a template for managing patients with a particular condition, and the embrace of process metrics, that try to ensure doctors are following the updated guidance – rather than, say, doing what perhaps they’ve always done because it’s what they know.
In practice, there are two problems with this system: one stemming from doctors who disregard these pathways, the other related to doctors who adhere to them.
Many physicians (perhaps especially in august academic institutions) worry most about their colleagues, especially those who practice in the community.
These physicians are often presumed by their university colleagues to be less attuned to the latest scientific literature, and hence neither of relevant advances in care nor practicing in a setting that encourages them to do so. Consequently, their patients may not be receiving the most optimal care, and have no way of knowing it.
But a second category of challenge involves the physicians who are attuned to guidelines, perhaps because they closely follow the literature, or perhaps because their health system nudges them to adhere. While arguably the patients in the care of these physicians are on balance better off than those in the first group, there are still important challenges – and embedded opportunities.
For starters, subjects in clinical studies are notoriously unrepresentative of the more general population – enrolled subjects tend to be healthier and to have fewer co-existing conditions, in effort to enable a clearer evaluation of the intervention, and provide a sort of “best case” read-out.
In addition, subjects in trials tend to be followed unusually closely – they may be reminded to take their medicine, for example, and encouraged along the way by study staff. While inclusion of a placebo group controls for the therapeutic impact of the extra attention (known as the “Hawthorne Effect”), it doesn’t account for the combined effect of experimental intervention plus attention – the increased likelihood that a subject in a study will reliably take a given medicine, say, compared to a patient in a doctor’s office, where the medication adherence rate can be astonishingly low. (It’s also why tech-enabled health service companies that feature a strong coaching and tracking component, like Omada and Virta, have gained considerable traction.)
As UCSF’s Dr. Fred Kleinsinger noted in a 2018 publication:
“Medication nonadherence for patients with chronic diseases is extremely common, affecting as many as 40% to 50% of patients who are prescribed medications for management of chronic conditions such as diabetes or hypertension. This nonadherence to prescribed treatment is thought to cause at least 100,000 preventable deaths and $100 billion in preventable medical costs per year.”
Finally, some patients in clinical practice may actually respond better than expected to a particular intervention – perhaps because of an intrinsic characteristic (involving their genetics or their microbiome, for example), or perhaps because of something subtle a particular physician or care provider did, a tweak that might goose the patient along a little bit. These are opportunities to learn from “happy accidents,” to institutionalize serendipity, so to speak, and benefit from the ingenuity of inquisitive physicians (medicine’s lead users, to use von Hippel’s term) –opportunities that are easily missed in the course of routine care.
The common feature of all these clinical care scenarios is the almost complete absence of tracking the one thing that matters most – actual patient outcomes over the long term.
- How is the psychiatrist doing with her patients with depression?
- How about the endocrinologist with his diabetics (in fairness, A1c is occasionally tracked)?
- Is the neurologist getting average, worse, or better, results than expected for patients with multiple sclerosis? For which patients?
A new medicine may get approved on the basis of robust clinical trials, but the approval of a drug, as I’ve argued, doesn’t necessarily equate with the improvement of outcomes of real world patients.
Why aren’t outcomes tracked in routine care the way they are in clinical trials? For one, it’s surprisingly hard to track patient journeys, especially as patients often get care from multiple medical systems – though even rigorously determining the outcome for a patient entirely within one medical system can be remarkably difficult, especially in the context of real life, when appointments are missed, and assessments can be spotty and inconsistent, and documentation unreliable.
But the second reason the information isn’t tracked is, essentially, no one (besides the patient!) really cares, in the sense of being personally invested in (and accountable for) the outcome. Certainly not in fee-for-service systems, where doctors (who, to be clear, obviously try their best for each patient) charge based on their activity rather than patient outcome. Hence, the extended push for approaches that seek to prioritize improved care, and specifically, improved outcomes. But even here it’s challenging – doctors will always argue that their specific patients are different – more complex or sicker, say, so of course they’ll do worse.
Often, the doctors may be right, and efforts to increase transparency around the success of certain surgical procedures has naturally led to notorious gaming of the system, where a mediocre heart surgeon who selects only the easiest cases scores higher than an exceptional surgeon who takes on the most difficult ones.
Attempts to adjust for complexity, inevitably, go only so far.
What emerges from this is a health system that, despite what may be the best intentions of providers, essentially, is flying blind, and lacks the basic ability to see what’s it doing, a system that lacks the fundamental ability to iteratively optimize and improve.
Yes, care improvement occurs – but over incredibly long periods of time, driven by the slow pace of robust clinical trials, rather than by the opportunities to systematically learn from patients and providers every single day.
For years, we’ve championed the concept of a “learning health system,” and today, it remains largely an unrealized aspiration.
What is to be done?
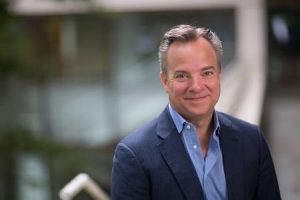
Mark McClellan, professor, Duke University
A remarkable recent two-day conference organized by Dr. Mark McClellan and colleagues at the Duke-Margolis Center (all materials available here) dug into exactly this challenge, drilling into the question of whether there can be some kind of convergence between the process of collecting and analyzing data for clinical trials and doing the same for clinical care; data collected outside of traditional clinical trial is often called “real world data,” or RWD.
While it would be a disservice to condense such a rich conference into tidy conclusions, there were nevertheless several powerful lessons that I took away.
For one, I was reminded yet again of the rigor and meticulousness of clinical trials. I knew this, of course, having designed, written and executed a number of studies, and having been immersed for years in research environments focused on this process. Even so, it was instructive to go through the challenges of defining who, exactly, has a particular condition – what is the definition of a “case?” What is the definition of an “outcome?” In the context of clinical trials, the criteria tend to be exceptional explicit, and evaluation of results can often require a deliberate, pre-defined process of adjudication.
The challenge, many speakers emphasized, is that doctors, busy taking care of patients – perhaps seeing patients every 15 minutes or 20 minutes (not uncommon, and I’ve heard of less) – barely have enough time to perform the minimum services (and documentation) required to get paid.
The detailed evaluation typically required in clinical trials feels like a luxury few busy physicians have as they fly through their day. Many speakers highlighted the importance of not contributing to provider burden, and several noted the increasing rate of doctor burnout, which some have attributed to the “death by a thousand clicks” nature of existing interactions with the electronic health record system.
Another huge challenge is that, while clinical trial data is typically captured in a dedicated database explicitly built with the desired analysis in mind, patient care data is often scattered across a healthcare system, or multiple healthcare systems, where it can be difficult to even know what other relevant data exist and might be germane.
For at least one speaker, UCSF breast surgeon and clinical trial innovator Dr. Laura Esserman, the right solution would involve the radical re-engineering of care delivery — “a sea-change in how clinicians practice,” so that clinical-trial quality data are routinely captured. By doing a much better job collecting data, and by improving how we gather and share data, she argues, we can ultimately both save time — by entering data once, and using it many times — and improve care.
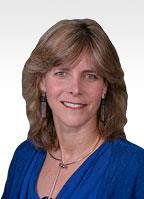
Laura Esserman, professor, surgery; UCSF
As Esserman points out (and I couldn’t agree with this point more), “Imagine a business where you have no idea what your outcomes are, no idea what your metrics are. We must be in the business of quality improvement.”
For many other speakers, the priority was searching for ways to improve the system while not requiring extensive changes in the way doctors practice. For all the discussion of stakeholder alignment, the intrinsic tension between providers and researchers was palpable, and acknowledged by a number of the presenters.
In one of the day’s best talks, Chhaya Shadra of Verana Health, a startup focused on real world data in several specialties including ophthalmology, neurology, and urology, argued “If you have to take time to improve documentation to help researchers, it’s not fair to clinicians whose primary responsibility is patient care.”
Similar sentiments were expressed by UCSF’s unfailingly insightful and articulate health IT policy researcher Julia Adler-Milstein, in a recent Annals of Internal Medicine commentary on a paper revealing that physicians spend around 16 minutes per patient engaging with the EHR system, about a third of the time on chart review (i.e. trying to find information), another quarter of the time on documentation (adding their own notes), and another 17% of the time on order entry – all told, a remarkable amount of hunting and pecking.
The dilemma we face, Adler-Milstein observes, is:
“How do we generate the foundation of clinical data needed to support the EHR’s many high value uses (including but not limited to clinical care) while doing so efficiently (for example, improving user interface design, using digital scribes, and simplifying documentations)? Even with the most efficient approach, physicians (and many other types of clinicians) will never obtain a direct return from future use of their documentation equal to their time cost of documentation. At a minimum, acknowledging this mismatch and making physicians feel valued for the time they spend in the EHR is needed. (emphasis added)
Many of the mitigation approaches Adler-Milstein described were also highlighted by speakers at the Duke conference: for instance, the founder of Google Glass company Augmedix described the company’s focus on serving as a digital scribe; other presenters emphasized the need for improved EHR user interface, and more generally, for more human factors research and design.
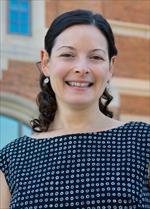
Julia Adler-Milstein, professor of medicine, UCSF
A number of presenters also emphasized the importance of understanding the questions you are trying to ask, and pointing out that for a number of applications – some population trends, for example – you may not need perfect data, and may be able to extract real value from what you have. But, even there, you still need to have a sense of both the quality of the underlying data and the nature of the existing imperfections, so that you don’t subsequently use the data inappropriately, and draw misguided conclusions.
Dr. Paul Friedman, chair of cardiology at the Mayo Clinic, offered examples that highlighted both the possibility and the limitations of using existing data. On the bright side, he highlighted a project demonstrating the use of artificial intelligence to deduce, with remarkable accuracy, a patient’s ejection fraction (a measure of heart output typically determined using cardiac echocardiography) from routinely collected electrocardiograms (ECGs) archived by the Mayo.
On the other hand, Friedman described the frustration of trying to predict COVID-19 infections (which may impact heart cells) using the sort of detection technology commonly available on smartphones and some wearables. In this case, he says, a key barrier turned out to be the lack of underlying standardization among these tools.
While all may report out something looking like a “standard” ECG, their approaches to deriving this are so different that you really struggle to develop an algorithm from these disparate data. Alignment around some set of standards involving sampling rate and dynamic range would help, he suggested.
Speakers evinced particular interest in the ability to stitch together multiple data types (say EHR data with claims data and specialty clinical data) using privacy-preserving technology like that offered by Datavant, a Bay Area startup cited by several presenters. This approach obviously won’t solve issues related to underlying quality of data that’s being linked, but it can help not only develop the rich data set representing a patient’s longitudinal journey, but by bringing together a range of sources, the technology may help surface — and in some cases, resolve — data entry errors and other ambiguities.
The opportunities – and the risks, especially around privacy – of collecting and including more data from wearables and other “consumer” products in a patient’s health record were also highlighted by several speakers. This 10-minute talk by Elektra’s Andy Corovos may be among the best overviews I’ve seen on this topic — a topic that for years has been especially near and dear to my heart.
As Veradigm’s Stephanie Reisinger noted in (another) compelling presentation, the consumerization of healthcare, including the increased use of devices and apps, represents a major healthcare trend, “empowering us to see and share health data,” and “driving informed consumers to demand a greater say over health journeys. Human bodies are becoming big data platforms.”
Of course, this possibility also relates directly to the sorts of security concerns Coravos and others highlighted.
What’s also clear is that pharma is looking at these large integrated datasets in different ways than they once did. Initially, pharma companies turned to real world evidence primarily in the context of health economics – typically “health technology assessments.” Primarily, pharmas were seeking evidence of real-world performance, to facilitate engagement (and negotiations) with payors (and, ex-US, regulators who require a discussion of relative value).
Today, Veradigm’s Reisinger says, the most common questions from pharma partners involve protocol optimization (understanding the impact of various inclusions and exclusion criteria and the number of patients you might be able to recruit, for example), patient finding (recruitment – an effort to connect eligible patients with suitable trials) and the development of synthetic control arms for some studies (for situations when a suitable control arm may not be feasible or ethically appropriate but a relevant basis of comparison is required, for example).
Finally, several speakers, including FDA’s Dr. Amy Abernethy, highlighted the potential value of real world data in understanding and developing effective therapeutics for COVID-19; this is the focus of the COVID-19 Evidence Accelerator, for example. The recent Surgisphere scandal involving COVID-19 data (I discuss this in detail here) was cited by several speakers including Abernethy, who highlights the need for “ruthless transparency.”
I left the conference feeling neither entirely elated nor thoroughly despondent – though perhaps with a healthy mixture of the two emotions. As Abernethy observed, “doing science is messy, and we’re doing science together as a community.”
There are real opportunities here, as well as intrinsically difficult hurdles – many presenters pointed out that the social/political/”psychological” (as one speaker said) hurdles are far greater than the technology hurdles. That said, it’s also quite possible that improved technology could catalyze advances that might highlight the potential value here and motivate further collaboration.
Another hope would be that an innovative health delivery system, perhaps reimagined along the lines that I’ve described here, and that Esserman has championed, emerges somewhere and proves so compelling to patients that it becomes the immediate gold standard, and is widely adopted.
A more immediate step might be the voluntary adoption of some of the technical standards for devices that Friedman describes.
In short, I came away impressed by the urgent need to improve how our healthcare system captures and shares data, and thus learns – or, presently, fails to learn – from patient experience. I also appreciate, along a range of dimensions, just why this seemingly urgent and obvious problem has remained such a stubbornly difficult nut to crack.
Above all, I’m struck, by the need – in the phrase of legendary NASA flight director Gene Kranz – to continue to work the problem.