Tech, Pharma, and the Uneven Distribution of the AI-Enabled Future
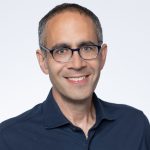
David Shaywitz
The worlds of technology and entrepreneurship are captivated by recent advances in generative AI and large language models (LLMs).
The arrival of ChatGPT, developed by OpenAI (a startup partnered with Microsoft), caused Google to declare a “Code Red,” akin to “pulling the fire alarm,” the New York Times explained. The latest class of startups at Y Combinator are reportedly flocking to AI. Students in the Harvard MS-MBA biotechnology program I advise tell me they are using ChatGPT constantly in section, and say the page is always open. I’ve also heard many medical trainees are routinely using it.
But if the future has arrived, as science fiction writer William Gibson famously observed, it’s not evenly distributed.
“People in tech are freaking out about LLMs, but not in science yet,” writes University of Rochester chemist and AI expert Andrew White.
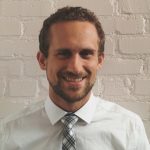
Andrew White
“AI hype may be too high,” White observes, “but we’re missing hype on smart people + AI…. this will be the biggest transformation in science since the internet. Universities must be completely rethinking education and research.”
White’s onto something. There is an absolute frenzy of activity in tech, and a rash of startups seeking to apply AI to some aspect of biopharma, often drug discovery.
But the view from within established biopharma companies tends to be more reserved.
Far, far more reserved.
Curiosity, wariness, weariness, and concern
As best I can determine, the view of incumbent biopharmas reflects a combination of curiosity, wariness, weariness, and concern.
There’s authentic curiosity around ChatGPT and AI more generally, wariness about the newest new thing, weariness about successive cycles of technology hype and disappointment, and concern about the many unknowns.
No one in the pharma C-suite wants to miss the AI train. Then again, no CEO wants to explain to the media how confidential company information was inadvertently leaked – as recently happened at Samsung. Biopharma also must be serious about patient confidentiality and strict adherence to regulatory process. Not surprisingly, the industry is loath to engage in anything that could put these vital priorities at risk.
Consequently, many biopharmas are taking a cautious approach, considering generative AI the way they routinely evaluate other emerging technologies. Rigorous guidelines for use are established, highly specified pilot projects are proposed, reviewed and subject to tiers of approvals. Once clear guardrails are established, the new technology is gently prodded and poked. The potential is gingerly explored.
It’s not just senior executives who are risk averse. Most of my colleagues in biopharma are skeptical.
After successive cycles of hope and disappointment, many seasoned drug developers just aren’t smitten by what seems like the latest shiny tech object. (I was probably in this camp as well before I started speaking with grounded AI experts like Zak Kohane and Peter Lee, who had early access to GPT-4, and began to appreciate over time that the technology represents something profoundly different.)
Many are still trying to understand what this technology can do. For instance, a colleague this week told me he tried GPT-4 for search and concluded “Meh. I’m still going to use Google.”
Playing around (or not)
What’s abundantly clear to me from Kohane, Lee, and others who have invested time in understanding GPT-4 is that:
- To really understand what GPT-4 can do, you need to spend a lot of time just playing with the technology, trying out all sorts of things, particularly in your area of expertise (like medicine).
- The more time you spend with GPT-4, the more it starts to feel like you’re developing a relationship with an extraordinary alien intelligence – powerful, imperfect, mystifying, intriguing.
Ideally – as one expert I spoke with this week suggested – the best way, in theory, for pharma colleagues to fully leverage GPT-4 would be to play around with it. This exploration would help determine the extent to which the technology could enable everyday tasks, and eventually contribute more profound insights.
Given the concerns about risk, I don’t see this happening in biopharma. While data scientists like Cloudera co-founder Jeff Hammerbacher famously aspire to “party on the data,” this unfettered, playful approach tends not to be the dominant mindset of large biopharmas, given their abiding concerns around potential downside consequences.
One way GPT-4 is likely to arrive in pharma is through consultant service companies like Tata Consulting Services, Accenture, and Cognizant, to use the three examples highlighted by Chamath Palihapitiya on the “All In” podcast. As Palihapitiya notes, these companies do coding-for-hire work at scale, and are thus likely to be the first to operationalize tools like GPT-4 (which can assist and enable coders) so these firms can accomplish more with fewer people.
GPT-4 will also come to pharma through applications, ideally from established, trusted vendors. Familiar Microsoft Office products supercharged by GPT-4 will be an early example. For many emerging tools, generative AI will operate under the hood, largely invisible. Users, understandably, are likely to focus on the capabilities an application is providing — the job to be done — rather than on the underlying technology making this possible.
Culture Contrast
The contrasting attitudes of established companies and startups towards emerging technology — including, but hardly limited to, GPT-4 — reflects radically different goals and relationships to perceived risk.
Large established companies are defined by their ability to execute reliably at scale. In biopharma R&D, this means being able to manage a portfolio of complex programs globally. Included: everything from the consistent manufacturing of a range of products to the safe, responsible, and efficient conduct of clinical trials to navigating different regulatory procedures across the world.
It’s a huge challenge to orchestrate these activities in parallel. The work requires a deliberate focus on establishing and optimizing repeatable processes, and making careful, deliberate choices – often involving multiple stakeholders – to ensure any risks to the established business are identified and hopefully mitigated.
Startups typically can’t, and don’t, work this way. Wall Street Journal technology columnist Chris Mims recently highlighted the prismatic example of Noam Bardin, a startup CEO who was absorbed into Google after his company, Waze, was acquired.
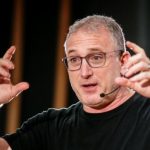
Noam Bardin
“What seems natural at a corporation,” Bardin said, “multiple approvers and meetings for each decision—is completely alien in the startup environment: make quick decisions, change them quickly if you are wrong.”
Bardin also described to Mims the differences in incentives he noticed before and after the acquisition. “Before the sale,” Mims reports, “everyone’s financial interest was aligned with the performance of the company’s products. Once Waze was a subsidiary, getting ahead was all about getting promoted.”
As I’ve discussed (see here, here) a nearly identical description is found in Safi Bahcall’s Loonshots. The author, a former biotech executive, explains how disruptive innovation can be stifled by corporate processes dominated by risk-aversion and enlightened careerism.
Suffocating processes designed to reduce risks are reportedly what caused Google to fall behind OpenAI and Microsoft in generative AI, according to the WSJ. “Now Google, the company that helped pioneer the modern era of artificial intelligence, finds its cautious approach to that very technology being tested by one of its oldest rivals [Microsoft],” the Journal reports (also covered nicely in this WSJ-associated podcast).
The risk aversion of large, established companies creates an opportunity for risk arbitrage. Startups can naturally shoulder more risk because they have less to lose and potentially more to gain. As I’ve discussed, this is reportedly how PayPal was able to defeat eBay’s attempt to develop a rival online payment product with Wells Fargo (called Billpoint). It is almost certainly why the most important generative AI applications for biopharma are likely to be developed by someone else.
Bottom Line
While many technology companies view generative AI as essential technology that must be adopted as an existential, urgent imperative, established biopharma companies are taking a more cautious approach, curious about the opportunities, but wary of the many uncertainties and risks.
Additional Astounding columns on generative AI: