Rebooting AI in Drug Discovery on the Slope of Enlightenment
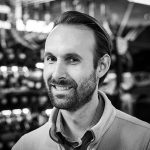
Jason Steiner, Architect & Advisor, AI-Drug Discovery
The past few years have seen a wave of AI investment in drug discovery in both large pharma companies and in venture-backed biotech startups. Expectations are running high. Management teams are betting that even marginal improvements on the ~90% failure rate of clinical trials will be worth the investment.
While there have been hints of improved R&D metrics in speed and cost, there has not yet been a clinical approval of a drug that may genuinely be considered “AI generated.” Benevolent AI and Atomwise, a couple of well-known AI-driven drug discovery startups, have made large strategic shifts in the aftermath of failures in clinical translation.
As AI tools mature, however, we are working our way through the Gartner hype cycle to the early stages of the Slope of Enlightenment. The future of AI in drug discovery is brighter than ever. The rise of “AI-first” biotechs, major strategic initiatives from pharma leaders like Genentech, GSK, and Sanofi, an ecosystem of industry providers developing products for the entire R&D pipeline, and a keen focus from regulatory agencies like the FDA are all pointing toward a more productive future.
The path to an approved clinical product is long and we haven’t seen an obvious AI drug discovery success, like a novel molecule invented in whole cloth by AI sailing all the way through the clinical trial process to FDA approval (though some companies may advertise this).
That’s probably a bit further out in the future, but when it happens, the whole world will know. In the near-term future, we might expect to see more substantial progress behind the scenes. There are many steps on the way where AI may be useful, and AI’s role in the fundamental mechanics of the drug discovery and development process are where I expect it to shine.
For those looking outside in, some of the major trends are detailed below:
Knowledge Management is King
This is not unique to pharma, however, its application across the massive data troves in both the scientific literature and proprietary databases is currently the most significant productivity lever. Efforts such as the JulesOS agent developed by GSK provide prompt-level access to the vast array of internal data to users without any need to know of such data a priori.
Similarly, an array of startups such as Elicit are providing products that can search and synthesize the scientific literature base en masse. Some of these capabilities are also being offered by frontier multimodal LLMs.
One such application was demoed by Google in its release of the Gemini model, showing the ability to scan hundreds of thousands of scientific papers, extract desired data, and update graphics and charts for review papers.
While it is still in early stages, the development of AI systems that can both synthesize, search, and reason across data is on the leading edge of scientific research in the form of “AI Scientists”. While de novo scientific hypothesis generation and testing is still in its nascency, the combination of generative models such as LLMs and search architectures such as those that powered the Alpha series of models from Deepmind, may enable a more automated form of science.
A key component of this will be building the physical and experimental systems that can translate AI-generated content into real physical testing and close the hypothesis/data loop.
Data is Different and Requires New Organization
One of the key shifts in life science research that began with genomics and is now expanding to many other types of biological information is the rise of “hypothesis-free” data generation.
In more traditional life science research, data has been viewed primarily as a means to answer a specific scientific hypothesis.
In the context of machine learning, however, data is viewed more from the perspective of the characteristics of its statistical distribution. This is a fundamentally different view on data generation.
As Aviv Regev, Genentech’s head of early R&D, has stated (paraphrasing) – they may make chemical compounds that will never become drugs, but that will be useful for training models that can generate many drugs.
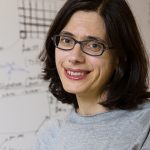
Aviv Regev
A key requirement of effectively implementing this strategy is the “lab in the loop” model that integrates wetlab and computational functions. This type of model is being pursued by an increasing number of AI-first biotech companies but remains relatively rare in traditional pharma where organizational structures often place computational groups as separate functional departments that more frequently default to service providers for the therapeutic and commercial units instead of being fully integrated.
A more comprehensive integration of wet and dry lab teams can yield greater efficiency for both, for example, by prioritizing better design of experiments for improved productivity. Such active learning has recently been demonstrated, for example, by improving the efficiency of experimental design to search the genetic perturbation space of CRISPR screens using prior knowledge and deep learning models. This closed-loop integration often requires large established companies to overhaul existing workflows and ways of thinking.
AI Applications Supersede Architecture and Scale
Much of the AI industry has consolidated around transformers as the architecture of choice for development because of its inherent scalability on existing computing accelerators. The term “LLM” has often become erroneously synonymous with AI in general. The primary focus of many of the frontier models has been toward massively increasing the size and scale of the data and compute they require to train.
However, for many applications (both in bio and beyond), this trend is not critical. Model architectures are becoming more computationally efficient and frequently getting better at learning from smaller and more curated data sets. Just in the past year, for example, new architectures for non-attention-based sequence models such as Hyena and Mamba were published that rival and may exceed performance metrics of attention-based models with significantly lower computational overhead.
Further, the architecture space of models addressing biological questions has been much more varied both in size and diversity than those in the LLM field. Smaller models with more task relevant architectures will continue to drive useful applications in drug discovery. Scale and attention are certainly not all you need to drive R&D productivity.
Realizing the Promise of AI in Drug Discovery
Platform technologies in the life sciences have ebbed and flowed in favor over the past few years. They hold the promise of dramatically improved long-term productivity often at the cost of high near-term investments. Major platforms like CRISPR, mRNA and AI hold tremendous promise for the future of medicine, and we are in the early days. The success of mRNA during COVID and the recent FDA approval of the first CRISPR cell therapy for sickle cell disease are key examples.
But the productivity power of a platform is demonstrated most powerfully not in the first success, but the second.
While both mRNA and CRISPR are specific modalities that have had decades of foundational research underpinning them, AI is a broad-spectrum enabling technology that applies to the industry writ large. It is being aimed to address the fundamental levers of cost, probability of success, and time to develop a product.
However, if we consider an average development time of 12 years and an average clinical success rate of 10%, the second success proof point is challenging especially if we have not yet seen the first. Even a doubling of the success rate and halving the development time — both tremendous achievements — would yield a net probability of 4% for seeing two clinical successes over more than half a decade, requiring a minimum of 25 pipeline candidates per platform.
The industry has gone through peak expectation cycles. Companies that have overpromised are in the trough of disillusionment. But the future of AI in drug discovery is just in the early days of the slope of enlightenment.
It’s an exciting future.
Jason Steiner is an architect and advisor specializing in AI for drug discovery. To read more about the intersection of technology and biology — Jason writes at Techbio<>Biotech
He is also a member of the Timmerman Traverse for Damon Runyon Cancer Research Foundation, a biotech industry effort to raise $1 million for young cancer researchers with bold and brave ideas.
As Jason puts it:
The pace of technological development in the life sciences is tremendous and is often being led by early career scientists pursuing innovative research efforts. Unfortunately, public funding for young scientists has been declining for decades. To ensure that we can keep the pipeline of future scientific developments strong, particularly to address major diseases like cancer, please consider making a donation.