AI in Pharma: Can We Get Beyond “Assent Without Belief” By Channeling Ethan Mollick?
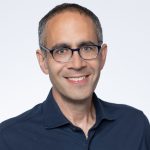
David Shaywitz
The phrase “assent without belief” has been used to describe the concept of going along with the outward manifestations of an ideology without true conviction. Most commonly, this is used to describe a familiar contemporary approach to religious observance.
It also seems to describe how the vast majority of biopharma colleagues view AI and other emerging digital and data technologies.
While few pharma employees are likely to stand up and object loudly when their CEOs boast in Davos about embracing AI, most also don’t consider AI as particularly relevant for their own work. Rather, if they think about AI at it, it’s typically viewed as merely the latest shiny object, breathlessly venerated by CEOs, and eagerly operationalized (at least in theory) by management consultants.
The upshot is that in practice, for most in pharma, AI specifically, and digital technologies more generally, tend to be seen as vaguely conceived top-down initiatives you need to navigate around in order to do you actual job.
Leading tech companies like OpenAI, Alphabet, Meta, and Microsoft, on the other hand, have profound conviction around AI. This is true not only for their executives but also for most of the engineers who are flocking to join. There is nothing performative about how these organizations are approaching AI.
Tech’s seriousness about AI is backed by investment. As a recent New York Times story notes, top tech executives are continuing to spend gobs of money on AI; their capital expenditures in the last quarter have increased 63% compared to a year ago, mostly AI-related. Even so, as the article’s headline notes, “a payoff still looks a long way away.”
The lack of an immediate return has troubled analysts at Goldman Sachs (as I recently discussed), and others, prompting concerns of a bubble.
Biopharma readers might take note of a striking recent report: a pharma CIO, after test driving an AI-enhanced version of Microsoft Office in his organization for six months, cancelled the upgrade, saying the additional cost (an extra $30/month per user) wasn’t worth it. Rather damningly, he described AI-produced PowerPoint slide decks as similar to “middle school presentations.”
Of particular interest – and something that I’m aware of occurring at other pharmas as well – the CIO noted that the ability to automatically take notes during Teams video meetings was regarded as such a liability by the Legal department (presumably because the document would be both legally “discoverable” and potentially incorrect or misleading) that this feature wasn’t activated by the company.
Ethan Mollick: AI Whisperer
These contrasting perspectives on AI would likely not surprise one of the most thoughtful voices on AI, Ethan Mollick. He is an Associate Professor of Management at Wharton, where he co-directs (with his wife, Lilach) the Generative AI Lab.
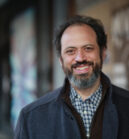
Ethan Mollick
Mollick, a former entrepreneur (and a scholar of entrepreneurship) who describes himself as drawn to technology but not a technologist himself, has become a celebrity of sorts for his ability to move effortless between the worlds of education, technology, and industry. He published the wildly popular Co-Intelligence in April, writes the “One Useful Thing” Substack, and advises policy makers including the Biden White House and companies such as J.P. Morgan, Google, and Meta.
Mollick is especially well-known for his compelling presentations and interviews; a recent podcast discussion with 20VC host Harry Stebbings provides a useful entry point into Mollick’s perspective and offers relevant guidance for those in biopharma trying to reconcile the excessive hype and ardent enthusiasm.
Mollick’s view is that while “everybody” has tried ChatGPT or similar models (like Google’s Gemini), only 5-10% of people in almost any typical company have even tried to use it more seriously, and only 2-3% of people have used it for at least the 10 hours Mollick believes is the minimum required to start to get a sense of what it can and can’t do.
In this sense, Mollick observes, “almost nobody uses these systems.”
One issue, Mollick argues, is that most large companies are fairly skittish about the use of AI. “The [local] regulatory environment is unclear,” he says, and argues that between absolute prohibitions, severe limits, and ambiguous restrictions (perhaps the most common challenge), many employees are understandably reluctant to use the technology. Because most don’t play around with the technology, Mollick argues, they’re unlikely to get familiar enough with it to start appreciating what it can and cannot do, and to figure out how to incorporate it into their daily work and life — a practice Mollick strongly encourages.
This is absolutely what I’ve both directly observed and also have heard about in biopharma specifically – lots of celebrating the idea of AI, but exceptional restrictions and anxiety around the actual use.
(There are exceptions: in my last role, I was able to partner with a spectacularly forward-thinking senior executive, Colleen Beauregard, and an imaginative data science colleague, Iksha Herr, to explore immersively the promise of genAI with Beauregard’s enthusiastic team.)
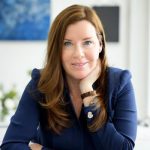
Colleen Beauregard
Consequently, Mollick explains, the use of generative AI in most organizations tends to occur in secret, as those who are adept at leveraging tend not to advertise this, fearing potential repercussions. This obviously tends to slow the adoption of the technology.
Mollick also emphasizes that generative AI is “jagged” – it’s “really good at some stuff, really bad at other things,” he says, adding “As a result, it can’t sub in for all of human work…The question is, can that jaggedness get overcome?”
While AI enthusiasts tend to focus on the technology’s “top line capabilities,” he argues, they often overlook the context — “the human systems” and “the organizational systems” — that these technologies “have to interact with.”
He adds, “We can’t be naïve about the work that needs to be done here to make this stuff operate. You can’t just drop these [technologies] in.”
Mollick contends that “we’re not even at the stage of integrating” generative AI into human systems yet. He adds that the way we interact with the underlying large language models is almost entirely via chatbot. He calls that an “insane process.” Mollick is excited about the downstream potential but anticipates “we have 10 years or so of integrating [generative AI] slowly into human systems” to get through first.
Wanted: “Skilled Artisans” or “Lead Users”
To effectively leverage AI, Mollick suggests, we should learn the lesson from the steam engine. The advances in productivity didn’t arise directly from Watt’s invention, he explains, but rather from “having skilled artisans in your factory, who said, I’ve got to the thing that can make power go back and forth. How do I create the gearing to connect that to [the tasks I want to do]?”
He continues, “It was the skilled artisans that made all of this work and made the manufacturers capture all the money. So you want to be a skilled artisan right now, you want to figure out how to take the back-and-forth power of an LLM and convert that into usable work inside your organizations.”
This perspective – I have typically favored Eric von Hippel’s term, “Lead Users,” but “Skilled Artisans” also works – aligns perfectly with perhaps the central message this column has repeatedly conveyed for years (see here in particular, also here, here, and here), informed by the work of James Bessen, Carlota Perez, and others.
In short:
- Transformative emerging digital and data technologies hold exceptional promise for the discovery, development, and delivery of impactful new medicines for patients.
- This potential isn’t likely to be captured by merely substituting the new technology for an earlier technology.
- Rather, leveraging new technology requires re-imaging and re-inventing the work, in ways that were not readily achievable with previous technologies.
- Beyond the reimagination, successive incremental improvements (as James Bessen and Robert Gordon have described, as discussed here and here) are also required to unlock meaningful productivity gains from new technologies.
- Productively integrating transformative technologies takes time, as Paul David, James Bessen and Carlota Perez have all argued. Productively integrating AI (contra rosy consultant predictions) will also take time, as Mollick eloquently explains and as this column has consistently emphasized.
- The key driving force for leveraging new technologies are the lead users/skilled artisans who are impassioned about solving critical problems and open to adopting new technology if it can help get the job done better.
- The fascinating question for biopharma where the effective adoption of new technologies like AI will come from. The contenders are:
- Large pharmas: well-resourced but exceedingly restrained, fairly impatient with their capital (given the demands of investors), and often defined by a famously challenging bureaucratic culture that can suppress innovation, as Safi Bahcall has chronicled (see here, here).
- Smaller biotechs and innovative techbio startups: more willing to take risks, but generally less well-resourced, and often have neither the time nor the financing to absorb the inevitable costly failures so common in drug development – see here.
- Ardent tech AI champions: have the appetite for risk and perhaps the financial resources and necessary patience but may lack the domain expertise (as emphasized by Mollick in the context of education) to know what they don’t know.
- My bet: adoption will be driven by agile biotechs and techbios who successfully re-imagine a critical aspect of the drug discovery and development process and – this is key — are lucky enough to be able to persuasively demonstrate this value before their funding runs out.
- Bonus recommendation: For more on the central role of contingency in success, see both this recent piece on parallels between success in film and pharma, and Cass Sunstein’s thoughtful, if somewhat dispiriting new book, How To Become Famous.