And Just Like That: What the Viral Adoption of a Clinical AI App Means for Pharma R&D
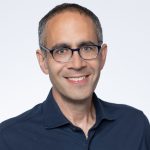
David Shaywitz
In 2011, we were experiencing the ascension of technologies like the cloud and the smartphone. Apps had become a thing: social network apps like Instagram (the iPhone “App of the Year” in 2011) and Twitter, utility apps like Evernote and Dropbox, navigation apps like Google Maps and Waze, and game apps like Angry Birds.
Yet in medicine, as I wrote that year in Forbes, the “killer app” was…a comparatively old-school e-textbook known as Up-To-Date. The company that created it was founded in 1992.
Written and reviewed by medical experts, Up-To-Date was where everyone in medicine, from earnest med students to overworked residents to seasoned clinicians, turned in the 2010s to find current, reputable information about medical conditions they required to most effectively care for their patients.
Ten years later, in 2021, Up-To-Date was still the go-to app; same for 2022 and 2023.
Yet today, this may be changing. When a colleague recently mentioned that young doctors now seemed to be using an AI-based resource called “Open Evidence,” I was surprised and somewhat skeptical.
But when I asked clinical colleagues who work with young doctors every day, I learned that the rumors seemed to be true.
As UCSF Chief of Medicine Robert Wachter wrote on X, “I think [Open Evidence] is becoming go-to resource for residents. It handles complex case-based prompts, addresses clinical cases holistically, & really good references.”
Harvard clinical colleagues shared similar experiences; one told me the uptake has been “viral,” adding, “I’ve NEVER seen anything like this.”
I know that my academic colleagues will be examining closely both the use and the impact of Open Evidence, with particular emphasis on the effect on patient care.
Lessons About Technology Adoption
For the biopharma-focused readers of TR, the Open Evidence example serves (or should serve) as a vivid reminder that things don’t change — until suddenly they do. A year ago, everyone was using Up-To-Date; today, many young doctors are embracing Open Evidence.
For emerging technologies, change tends to be driven by “lead users” (to use MIT professor Eric von Hippel’s term) – front-line workers who are focused on solving a pressing problem, and are glad to utilize whatever approach seems most effective.
When you are a medical resident, your pressing problem is the overwhelming number of things you are dealing with, coming at you from everywhere, all at once. You desperately want to provide the best care to your patients, and you are motivated to turn whatever resource seems most useful.
That Open Evidence seems to have met this threshold (at least for a number of early-career physicians) is strong testimony to its perceived value. Harried residents, presumably, are not using Open Evidence merely because they are curious about AI, or because there is a department initiative to utilize AI; they’re using it because they see the Open Evidence as the best solution for their problem. It’s a tool that’s been adopted because of the palpable value it provides.
To these busy young doctors, AI through Open Evidence isn’t a proverbial “solution in search of a problem.” It’s a customized tool addressing their immediate, pressing needs.
There’s an analogy from the field of genetics. For years, I remember hearing endless criticism of physicians for their reluctance to leverage genetics in clinical practice; the urgent need to better educate clinicians in genetics was a familiar, oft-repeated plea.
Yet, when a genetic diagnostic test (non-invasive prenatal testing, or NIPT) became available that could evaluate reliably specific fetal chromosomal abnormalities from a sample of peripheral blood, and in many cases obviate the need for an amniocentesis, the adoption was both rapid and widespread. Patients, doctors, and payors all seemed to embrace it – because the benefits were palpable.
Implications for AI in Pharma
Which brings us, predictably, back to AI in pharma.
In my last three pieces, I argued that:
- Picking winners consistently in R&D is a vexing challenge.
- Human causal biology, if leveraged rigorously and thoughtfully (eg Vertex, Regeneron) may be able to help.
- While many readers clearly remain skeptical, human causal biology, as leveraged by Vertex and Regeneron, is driving distinct value, and there will be further advantage to be found by thoughtfully leveraging AI.
Readers turned out to be even more skeptical about the application of AI to R&D than they were about the application of human genetics – and impassioned geneticists were often the most critical.
As one reader (not from the Boston area, incidentally) and genetics enthusiast wrote,
I also think you are far too bullish on AI – I really dislike statements like: “Emerging technologies like AI will help improve scientific understanding and enable better decisions”. We have no idea yet exactly how transformative AI will (or will not) be, and professing with certainty that it will provide value fans the flames of hype that drive so many scam companies to slap a branded faceplate on GPT4 or raise money from VCs with not real vision beyond “AI+$$$$$=awesomeness”.
The AI Chasm in Pharma R&D
I appreciated the candor and perspective, which were certainly familiar, and speak to the sizeable chasm that exists in pharma R&D between AI optimists and skeptics.
On the pro-AI side, there seem to be two largely distinct cohorts: a small group of scientifically sophisticated enthusiasts who are really excited to explore the promise of AI across R&D, and a larger group of “digital transformers.”
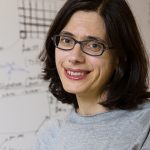
Aviv Regev
The AI-curious scientists, from what I’ve seen, tend to have very little status and organizational clout in most large pharmas, although there are exceptions (Aviv Regev at Genentech/Roche comes to mind). More often, at best, they seem to be viewed as adorable (a word I’ve actually heard used by digital transformers).
The mission of the digital transformers is to execute broad corporate initiatives that are launched from the C-suite, driven by management consultants and focused on operational efficiency, typically assessed by near-term process metrics. These organizational ambitions, invariably emphasizing the infusion of AI across the enterprise, are trumpeted by CEOs at Davos and by big pharma execs at industry conferences like HLTH.
But turning a means into an end can be problematic. Goodhart’s Law (see here)observes that “When a measure becomes a target, it ceases to be a good measure.” Similarly, when the mere use of AI becomes the goal, rather than a tool, the result can be a perfusion of performative AI and a dearth of thoughtful application to address the most critical problems a pharma faces: discovering and developing the next original, impactful medicine.
Consequently, it’s understandable why the vast majority of pharma R&D veterans remain generally skeptical about AI in R&D, since it seems to bear all the stigmata of The Next Great Corporate Initiative that needs to be endured in the process of actually doing great science and coming up with impactful new medicines.
The wild hype around AI doesn’t inspire confidence either. While most startups aspire to lofty goals and tend to launch with brash promises, the extravagant expectations offered by AI startups may be in a league of their own.
As industry chemist and distinguished “In the Pipeline” blogger Derek Lowe recently reminded readers, in 2014, Recursion Pharma “stated back then that they were going to develop 100 drugs in ten years” – an outlandish proposition that made it difficult for many experienced drug developers to take them seriously.
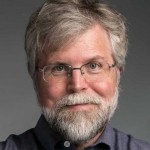
Derek Lowe
My concern is that understandable skepticism can easily bleed into reflexive cynicism (I’ve discussed the “cynicism trap” here), that might lead R&D teams to overlook early but authentically promising opportunities that could be truly transformative.
It’s especially disappointing to me to sense some of this cynicism emanating from geneticists in particular, since at the time that many these geneticists were leaning into the tools and technologies of large-scale genetics, they were on the receiving end of critics who doubted the promise of the approach.
A representative article, from Stephen S. Hall in Scientific American in 2010, was titled “Revolution Postponed: Why the Human Genome Project Has Been Disappointing.”
The subheadline to Hall’s piece reads: “The Human Genome Project has failed so far to produce the medical miracles that scientists promised. Biologists are now divided over what, if anything, went wrong—and what needs to happen next.”
Yet over time, and with a huge amount of effort (and financial resources), the value of the Human Genome Project and related endeavors (like the UK Biobank) started (arguably) to prove themselves. (See, for example, this 2020 article by Richard Gibbs.)
True, genetics has perhaps not lived up to some of the most hopeful early expectations (see the thoughtful comments of Princeton geneticist and computer scientist Olga Troyanskaya here), but by any reasonable estimation, the efforts have proved extraordinarily enabling for science, medicine, and biopharma R&D.
Bottom Line
I expect AI will ultimately prove similarly transformative, and, when developed wisely and utilized thoughtfully, will be viewed as an essential tool for managing the burgeoning complexity of biopharma R&D. Less certain is when such palpably useful AI tools for advancing R&D science will start to arrive: this year? This decade?
Like the young doctors now relying on Open Evidence, pharma R&D scientists may soon discover – perhaps sooner than you think – that the use of AI has become second nature for us, part of the fabric of our work, and we may wonder how we managed to survive so long without it.