Learning From COVID-19: The Lessons For Real World Data
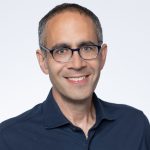
David Shaywitz
The COVID-19 crisis created an urgent need for healthcare data.
For starters, it was necessary to characterize the spread of the pandemic. Quickly, reports were needed on the capacity of healthcare facilities responsible for care of the severely afflicted. Then there was the urgent need to assess the trajectory, and outcomes, of patients admitted to hospitals.
The profound difficulty our healthcare system has had responding to each of these needs – despite the often heroic efforts of so many dedicated individuals – has revealed critical gaps in the way healthcare data are gathered, shared, and analyzed.
The challenges of defining the spread of COVID-19 relates in part to existing deficiencies – “We don’t really have a public health infrastructure,” Walmart Health’s Senior Vice President Marcus Osborne explains.
The Centers for Disease Control and Prevention also made some high-profile blunders early on – perhaps most prominently, the distribution of a flawed initial test for the virus, which forced the country into catch-up mode from the start, as the New York Times and others have discussed. Also a key factor: the Trump’s Administration’s apparent distrust of, and disdain for, the established experts in the public health community; a representative headline, from Axios: “Trump’s war on public health experts.”
The failure experienced by hospitals in assessing capacity has been persuasively documented by Wall Street Journal reporters Melanie Evans and Alexandra Berzon.
The challenge of understanding collectively what happens to patients once they’ve been admitted to a hospital may be less visible, but remains equally problematic. We are far better at, or at least more diligent at, determining what a patient should be billed for than determining at the most basic level how they actually fared, both individually and for most categories of patients.
This represents the “feedback gap” I recently described, in the context of a July conference on “Establishing a High-Quality Real-World Data Ecosystem” organized by the Duke-Margolis Center.
Recently, the Margolis Center convened another meeting, focused specifically on “Applying Lessons Learned from RWE [real world evidence] in the Time of COVID-19 to the Future.” While the individual presenters were uniformly hopeful and optimistic, I emerged from the proceedings with the strong sense that the pandemic has thrown into sharp relief a number of persistent and long-standing challenges.
Those who are interested can watch the conference video on YouTube.
Several topics caught my attention.
First, related directly to the origin of the feedback gap I previously described, is the idea emphasized by UCSF’s Dr. Laura Esserman. In typical care, “we don’t get outcomes on everybody – that’s a problem with medicine.” She added, “We should track outcomes on everyone, and isn’t that just real-world data?”
She continued,
“Our current electronic health records are not organized for quality improvement and you shouldn’t have to go to the IRB to get permission to collect the data that allows you to do your job.“
In other words: how can we improve the care we routinely provide if we’re not routinely, and systematically, determining and analyzing how the patients we’re currently taking care of are doing?
Dr. Robert Califf – legendary cardiology clinical trialist, former Commissioner of the FDA, and now Head of Clinical Policy and Strategy at Verily – highlighted a consequence of this failure: the vast amount of clinical practice is not informed by high-quality evidence. He cited a recent study that reported how few of the cardiology guidelines (just 8.5% of the recommendations in the American College of Cardiology/American Heart Association guidelines) are based on the highest level of evidence (supported by multiple randomized controlled trials – RCTs). Unfortunately, this pattern that doesn’t seem to have changed in the last decade.
While we have a robust clinical trial enterprise, Califf explained, it isn’t meeting a number of critical needs. In particular, he says, “We are not generating the evidence we need to support the healthcare decisions that patients and their doctors have to make every day.”
Fixing this, he says, will require us to “deal with the fragmentation and misaligned incentives in our system.”
As I’ve argued, a key “reason the information isn’t tracked is, essentially, no one (besides the patient!) really cares, in the sense of being personally invested in (and accountable for) the outcome.”
The consequence of our failure to collect – and the lack of adequate motivation to routinely collect – the information we need to improve care, even at the level of most individual hospitals, much less the regional and national level, has been felt especially acutely by FDA Deputy Commissioner Dr. Amy Abernathy. An expert in real-world evidence from her Duke and Flatiron Health days, Abernathy has been seeking to organize the incoming COVID-19 data and analyze it through collaborative efforts such as the COVID-19 Evidence Accelerator (in which I’m a participant).
Reflecting on what she’s learned, Abernethy highlighted what struck me as the observational research version of Mike Tyson’s memorable epigram, “Everyone has a plan until they get punched in the mouth.”
In the case of learning from COVID-19 RWE, there was important methodological lesson to be learned from the challenges of even the seemingly most basic elements, such as defining “time zero,” determining what constitutes a hospital admission, and discerning whether a patient was receiving intensive or critical care.
Many of these issues were surfaced, Abernethy noted, at the Evidence Accelerator, when participants were encouraged to show their work, and get into the critical “nitty-gritty.” Some of these challenges were also highlighted by conference participants, including Harvard’s Griffin Weber.
Abernethy also pointed out that we’ve become relatively proficient at understanding at a glance what to look for in a high quality RCT, assessing attributes like adequate statistical power and how the blinding was managed. Now, she said, we need to develop this intuitive understanding for observational studies as well.
Abernethy also emphasized the need to refine our conception of RWE. We often tend to view RWE-driven studies as a “replacement product” for RCTs – but this framing may be misleading and distracting. Everyone would like to have robust RCTs to answer every question, she said, but that’s not possible, and we need RWE “to fill in the gaps.”
RWE, she emphasized, can be used for a range of purposes, such as understanding patterns of care, or deciding which RCTs should be conducted.
This is a critically important idea: the value of RWE is not as a substitute for RCTs, but rather to more effectively capture the totality of data in the healthcare system, and to provide information about healthcare as it’s actually practiced, within the acknowledged messiness of routine care, as I’ve discussed.
I was also struck by Abernethy’s focus on the importance of high-quality datasets, which would seem to be the cornerstone of meaningful analytics. Abernethy highlighted the problem of data gaps, and the need to link data sets and fill in missing data using different data sources, in effort to approach a level of “completeness” that would enable meaningful study. She noted that technology might be helpful here, in the form of “synthetic controls” (statistically generated comparators based existing data; a nice explainer from Jen Goldsack here) and the use of tokenization (an approach to de-identification of data permitting it to be shared; a useful white paper from Datavant, a leading startup in this space, here).
Abernethy also offered what I thought was spot-on advice regarding the development and application of technology, and some important advice about how healthcare could more effectively engage with technologists and tech companies.
There’s a pervasive problem, she said, with “vendor-think” – the idea that the healthcare stakeholder (hospital, payor, biopharmaceutical company, health services researcher) specifies what a vendor needs to provide, and then the vendor “builds against that list.”
She described with perfect clarity not just how many large healthcare organizations typically approach large projects, but also the mindset within healthcare organizations that I’ve witnessed and described, where data experts and statisticians are often treated as second-class citizens.
What’s needed, she persuasively argued, is for authentic collaboration, where you have at the same table not just the manager or executive, say, who’s sponsoring the project, but also healthcare domain experts, who understand the subtleties and context of how the data were generated, as well as the technologists – the data scientists and engineers who can build and refine the solution.
Such ongoing collaboration not only ensures a better mutual understanding of needs, but also enables the work to proceed iteratively, and to evolve as the participants refine their understanding of both the problem to be solved and the solutions that can be envisioned.
Achieving this balance is notoriously difficult, and vanishingly rare to see in practice. This barrier – a hurdle in organizational dynamics as much as technological expertise – also represents an exceptional opportunity for an integrative and empathetic leader who can not only bring the right people to the table, but (and this is the hard part) ensure their talents are fully elicited and authentically embraced.