Rebuffed as Overlords, AI Experts Return in Peace, Seeking Partnership with Clinicians
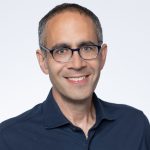
David Shaywitz
Why not healthcare?
That’s the core question at the heart of efforts to apply emerging digital and data technologies to healthcare and life science.
As Suchi Saria, an entrepreneur and a computer scientist at John Hopkins, where she directs the Machine Learning and Healthcare Lab, puts it, in the 2000s, these technologies transformed sectors, such as banking, in a fashion that was “kind of amazing.”
Artificial intelligence (AI), operating on these rich data, profoundly changed and improved the way business is done.
Take, for example, banking fraud detection. The industry “can’t imagine doing it without AI, and with AI they’ve increased sensitivity dramatically, timeliness dramatically” and have far improved specificity, Saria notes.
Contrast this with the AI experience in healthcare. In the last decade, healthcare has “basically spent a ton of our investments” to go “from no data to data, going from no digital infrastructure to additional infrastructure,” says Saria, but yet, “when we think of AI, we think of it as a thing that could be transformative, that has the potential, that is in the future.”
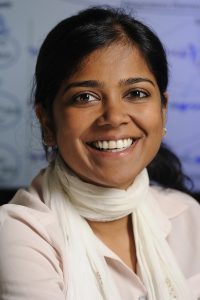
Suchi Saria, Founder, Bayesian Health; John C. Malone Associate Professor and Director of AI & Healthcare, Johns Hopkins University
What we’re missing, insists Saria, is that the future is now – “in reality, today. Now that the data exist, the use of AI in deriving value from the data that’s being collected is the single biggest opportunity in healthcare.”
It’s a hopeful perspective, though of course not universally shared. But it is hotly debated, perhaps nowhere more intelligently than at the recent, inaugural SAIL pre-symposium (virtual, of course) focused on AI and health, and featuring many of the field’s most thoughtful voices, including data scientists, clinicians, administrators, and even the Editor-in-Chief of the august New England Journal of Medicine (NEJM). All offered comments that were almost invariably germane, focused, and informative, representing the best of what conferences can be. You can watch the whole thing yourself here.
Six major themes emerged in my notes:
- The challenge of outcomes – what should we, and can we, seek to optimize?
- The centrality of bias – the need to ensure AI isn’t perpetuating and exacerbating inequities.
- The consensus for the “doctor and AI” mindset – rather than “doctor or”
- Promising use cases – the “green shoots.”
- Opportunities in evidence generation – and why leveraging electronic medical record data remains so hard.
- Stubborn hurdles and implementation challenges – including interoperability, data access, and conflating “interesting” and “important.”
The Challenge of Outcomes
Almost by definition, the goal of medicine is to improve outcomes. As NEJM editor Eric Rubin puts it, “we are interested in the impact on the patient,” adding “the closer we can get to something that we care about, the better off we are.”
Similarly, the lens through which UnitedHealth Group’s Chief Scientific Officer, Ken Ehlert, views potential AI solutions is “are we getting a better outcome?” Entrepreneur and academic ophthalmologist Michael Abramoff also stresses the importance of focusing on outcomes.
But such focus turns out to be easy to say but far more difficult to operationalize, as Harvard’s Zak Kohane points out.
“We’re not very good at looking at outcomes because the systematized capture [of outcomes], whether in trials or EHRs, is noisy, confounded.” He predicts that “many, if not all the AI programs that are going to be deployed in the next 10 years will be poor with respect to outcomes and rich with respect to either human labels or intermediate process measures.”
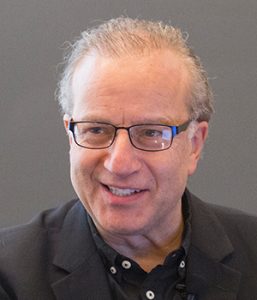
Zak Kohane, Chair of the Department of Biomedical Informatics, Harvard Medical School
These endpoints can seduce and mislead us, he suggests, leading us to optimize for something we regard as a proxy for meaningful outcomes, yet which may ultimately not be linked to the outcomes as closely as we’d like to imagine.
Kohane (a pediatric endocrinologist) cites the example of diabetologists seeking to improve microvascular disease by focusing on driving down the levels of glycosylated hemoglobin (HbA1c); this turns out to work, to a point, in terms of reducing kidney damage, but pushing “too” intensively for very low HbA1c levels was ultimately found to increase the risk of death. We were “misled by the process outcome in this case,” Kohane says. “For adults, minimizing glycohemoglobin was actually the wrong thing.”
“Medicine,” reflects Kohane, “is a beautiful art but it’s barely a science. As a result, many of our intuitions of what constitutes a solid correlate to outcomes, again and again, gets proven to us to be wrong.”
It’s also important to recognize, as University of Utah Health’s Chief Medical Information Officer, Maia Hightower, points out, that “the outcomes that we as clinicians may see as important may be different than what our communities see as important.”
The Ubiquity of Bias
Despite, or perhaps because, of a series of high-profile failures (like an AI-powered image classifying program that was able to recognize categories as fine as “Graduation,” yet mislabeled people of color as “Gorillas”), the AI community has tackled this challenge. The AI community has transformed itself from laggards to leaders, as Brian Christian captures in a captivating new book, The Alignment Problem, that I recently reviewed for the Wall Street Journal.
Duke University computer scientist Cynthia Rudin highlights a prismatic example of bias – an insurance company algorithm that aimed to predict which patients might need more care in the future, and thus might benefit from extra (anticipatory) services today.
The company used “cost as a proxy for care,” in their modeling, Rudin says. “The only problem is that black patients were receiving lower cost health care. They weren’t less ill. They were just receiving lower cost health care.” But these patients would have been systematically underserved by the algorithm’s recommendations.
Anant Madabhushi, who directs the Center for Computational Imaging and Personalized Diagnostics at Case Western University, offers another example from his own research on prostate cancer. Black men “tend to have more severe disease,” Madabhushi says, and “potentially higher incidence of prostate cancer,” yet “a lot of the existing risk models that we currently have for prostate cancer have been built largely with a plurality of non-black men represented in those datasets.”
Attuned to the possibility of racial differences in the disease – as IBM Watson’s Tiffani Bright points out, “you can’t measure what you don’t know about” – Madabhushi uncovered “actual differences in the area around the tumor” in pathology specimens taken from black men and white men. From this, they “created a dedicated model” that “resulted in a much higher accuracy in predicting risk of recurrence,” compared to a “population-agnostic model.”
At one point, there might have been a collective sense that the best way to avoid bias is to avoid collecting data that might predispose to bias, like race. But a key theme emerging from both this discussion and Christian’s book is that appropriately collecting and thoughtfully considering these data can be essential and invaluable.
“From an operations perspective,” explains Hightower, there’s now the “expectation within the healthcare system [that we’re] capturing all other types of data to tell the complete story of our patients.” She says they “do gender pretty well,” but are “not as good with race. And definitely when we talk about preferred language and LGBTQ+ status, it starts to deteriorate even more.”
As Kohane points out, such information can be critical. Consider hereditary breast and ovarian cancer (HBOC), associated with mutations in the BRCA1 and BRCA2 genes. Ashkenazi Jews are at significantly greater risk of carrying one of these mutations; according to a genetic counselor at Jackson Laboratory, “one in 40 Ashkenazi Jewish individuals versus one in 400 people in the general population carry a mutation in BRCA1 or BRCA2.” If a provider does not customize their calculation, “if they do not discriminate based on the ethnicity of the patient being a Jewish woman,” Kohane notes, “they are actually underserving that patient in a very unfortunate way. And I think that [customizing treatment based on factors that, where appropriate, include ethnicity, for example] is going to be increasingly true as we get more precise about our medicine.”
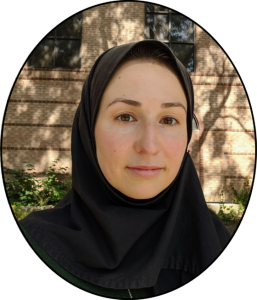
Marzyeh Ghassemi, Assistant Professor, University of Toronto, Computer Science and Medicine
Marzeyeh Ghassemi, a Canadian computer scientist focused on the application of machine learning to healthcare, and whose lab will be moving to MIT next summer, has thought deeply about fairness and bias. She is especially concerned about “unjust bias” – bias that “perpetuates systemic structural injustice that’s been visited upon a certain group for many reasons.”
One example she cites: the historical tendency for women’s pain to be “ignored when they go to the doctor.” Consequently, she says, if we feed that data into a model, “we make an algorithm that perpetuates that structure, systemic injustice. That’s a bias that’s bad, and we don’t want to do that.”
One specific suggestion Ghassemi offers: regulators requiring “performance guarantees across different subgroups.” She emphasizes considering such performance proactively, and demonstrating it, represents a better solution than perhaps the more convenient alternative of “narrowing the scope of your claims” – i.e. seeking approval only for a single group. Otherwise, she says, “we going to end up with a lot of devices and algorithms and bells and whistles and treatments that only work on wealthy white people.”
Kohane points out that the computer science community was two decades ahead of medicine in embracing open access publishing. “There is an interesting set of precedents where good societal behavior has actually been pioneered by the computational community,” he says, suggesting that perhaps computer scientists, as they seek to bring AI to medicine, could set another good example here as well.
Doctor and AI
While many journalists seem permanently stuck on the “will AI replace doctors?” storyline, the field itself moved on a long time ago, driven, it seems, less by political expediency – the idea that AI will be an easier sell if doctors are less threatened by it – than by authentic scientific humility.
Many leading AI practitioners have recognized the limitations as well as the power of their computational tools, and see in partnerships with people an opportunity to at once bring out the best from both computer and human while also guarding against some very real concerns.
Among the top worries: the unexpected fragility of AI algorithms. Approaches that seem to work brilliantly in a defined set of circumstances may fail catastrophically when the situation is changed – even imperceptibly.
For example, fascinating studies involving “adversarial attacks” have revealed that a seemingly sophisticated image recognition algorithm can be tricked by simply altering, in some cases, a single (well-chosen) pixel. Similarly, AI researchers working in healthcare have become increasingly worried about black box algorithms delivering misguided recommendations based on subtle flaws that may lurk undetected – as I discussed at the start of my recent WSJ review of Brian Christian’s The Alignment Problem.
As Duke’s Rudin (also one of the stars of Christian’s book) explains, right now, when thinking about the application of AI to many aspects of healthcare, “we don’t trust our models.” They might be “reasoning about things the wrong way.”
To leverage the power of AI while mitigating the risks, Rudin’s group is focused on using AI to develop clinical decision aids that are based on simple point schemes, to derive the sorts of scores that physicians are already accustomed to calculating – to ballpark a patient’s cardiovascular risk, for example.
The trick is using the sophisticated AI to figure out the most relevant variables to measure (a computationally difficult problem) and distill these parameters into simple integers, which a busy physician can still add up and evaluate in the context of the patient.
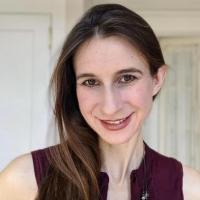
Cynthia Rudin, professor of computer science, Duke University
The scores produced by this approach, Rudin says, “are just as accurate as any model you can construct.” Plus, they offer the conspicuous advantage of being interpretable – the physician can “really understand how the variables work together jointly to form a final prediction,” she adds. Furthermore, “being able to have the human in the loop actually helps you with the uncertainty that you can’t quantify – the ‘unknown unknowns.’”
There’s also a hope that AI can help aggregate, organize, and prioritize the huge amount of information physicians and other health providers need to contend with, helping to distill for them the information they need – when they need it.
Both Microsoft’s head of research Peter Lee, and UnitedHealth’s Ehlert, for example, envision AI “augmenting what humans can do, absorbing and integrating knowledge for better decisions” as Lee puts it. The ability to process health information in real time, Saria believes, will enable medicine to (finally…) transition from a “reactionary paradigm to an anticipatory paradigm,” anticipating disease in time to prevent it or at least head it off at an early stage. For example, argues Saria, “AI is pretty much the only way to identify conditions like sepsis, and patients at risk of sepsis, early and precisely.”
Saria cites the management of stroke as another example, where AI can rapidly identify likely occlusive events, which a radiologist can immediately review and potentially validate, facilitating the timely triage of patients to a comprehensive stroke center for appropriate treatment.
Both Saria and Ehlert also flag the opportunity for AI to offer providers reference values for measurement that are personalized and contextualized for each patient, rather than based on average values for the population as a whole.
Columbia University biomedical informaticist Nick Tatonetti may have captured the shared sentiment the best, observing:
“Medicine is really about human interactions. Caring for somebody and curing someone of a disease is an extremely human activity. And humans should be centered in that process. A lot of technology that’s been introduced in health care has been rightly criticized for getting in the way of the patient doctor relationship, that human connection. There really is an opportunity for technology not to get in the way any longer, but start to disappear into the background and really put that interaction in the center.”
Uses Cases
Several speakers offered concrete examples of the application of AI in medicine. A particularly intriguing example, presented by Greg Hager, a computer scientist and director of the Malone Center for Engineering in Healthcare at Johns Hopkins, focused on surgical training.
Hager explains that the widespread adoption of the da Vinci surgical system, which enables surgeons to operate with robotic assistance, almost as if they’re playing a video game, offered a remarkable opportunity. The da Vinci’s recording of all aspects of a surgical procedure, from stereo videos to the force applied to the instruments, Hager realized, generates a fantastically useful dataset. By thoughtfully bringing AI to bear on these data, Hager and his colleagues break procedures down into steps, and “evaluate the quality performance of those steps.”
This analysis can determine “whether it’s an attending [senior physician] or a trainee who’s operating, just by the quality of the performance in that data.” Plus they can feed the data back into training. “Once we know where you lie in the skill scale,” Hager says, “we can understand where your potential deficits are, and we can turn that into a training regime so we can now say, look, here are the things that would be most useful for you to work on to improve your surgical technique.”
Perhaps not surprisingly, other use cases involved imaging.
Hager, for example, described the development of an algorithm intended not to replace radiologists, but rather, to enable them to use their skills most effectively. The approach he described would analyze mammograms, and sort them “extremely reliably” into two categories: clearly normal and everything else. This would “use the machine to replace the drudge work, the over and over again work,” and instead “allow radiologists to focus more at the tip of the pyramid, the place where there’s really high value and [the critical need for] human input.” As he summarizes, “We should be thinking about augmenting people,” and says the way to do this is “to allow them to focus on the place where people have the most value.”
Pathology offers another promising opportunity for the application of AI, Case Western’s Madabhushi points out. In an approach similar to Hager’s, pathology slides might be pre-filtered by a measure of complexity, with the most difficult cases presented to the pathologist when she is the most alert.
He also cited an Israeli company whose software provided “second opinion” reads, reviewing slides that pathologists had already identified as benign. This (theoretically) minimizes the downside risk, while enabling the identification of lesions that initially escaped human detection. (Of course, the concern would be the Peltzman Effect – the worry that pathologists might become less diligent in their initial reads if they thought a computer was likely to double-check their work.)
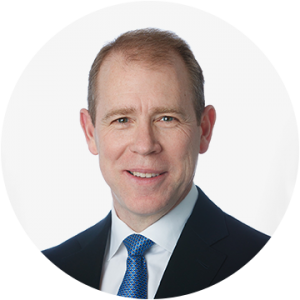
Ken Ehlert, chief scientific officer, UnitedHealth Group
More generally, both UnitedHealth’s Ehlert and Microsoft’s Lee express hope that AI could also improve our understanding of biology, and complex biological networks. Ehlert also suggests a useful function for AI could be producing for each patient a “patients like mine” function. The idea is that it would be enormously empowering for physicians if an algorithm could review data from millions of patients, presenting the doctor, in real time, with information about how similar patients fared, and what treatment approaches worked best. (This capability, as I’ve described, is painfully absent today.)
Lee, meanwhile, points to the opportunity for AI to provide doctors with “an intelligent assistant” function, “ambient clinical intelligence” that could listen to a physician interact with a patient and set up a clinical note for her accordingly.
From EHR to Evidence (?)
The digitization of the electronic health record would seem to present an enormous opportunity for learning and care improvement. Yet, as I’ve repeatedly and perhaps obsessively discussed (see here, here, and references therein), delivering on this promise has proved exceptionally difficult.
One issue seems to be a misunderstanding of what an EHR is, and isn’t. Essentially, we tend to think we are directly learning about patients, yet what we’re really learning, Kohane reminds us, “is the behavior of doctors.” He adds, “most events, most of the data items, are created by the doctor. So you’re actually learning from the doctor. You’re not learning from the biology.” We need to recognize the difference between the two, he cautions.
Kohane draws a contrast with the relative feasibility of using images as a substrate for AI. While acknowledging potential sources of variability in images (a biopsy of a heterogeneous tumor, for example, may happen to catch an unrepresentative sample), Kohane explains,
“I’m going to be much more confident about image-based metrics than I am about time series, EHR-based metrics, because I just know how much more variation there is [compared to] the slab of tissue that’s obtained in the OR or the retinal image. I assure you, it’s less than the practice of medicine in different cities, and how current, aggressive or venal different doctors are in different systems. That’s going to make our evaluation process for those algorithms that are very doctor-in-the-loop dependent, quite tricky to evaluate.”
Rubin of the NEJM also points to challenges of relying on EHR data. “As someone who contributes to the charts all the time, I’d say that a lot of them are driven by insurance claims rather than caring for the patient or getting the most complete collection of information on that patient.”
Adds Rubin, we need to think about “the purpose of the data that we’re going to collect, because if we can anticipate that purpose, we can do a better job of collecting data that fulfills that purpose.”
Yet even with their limitations, EHRs still capture data that would seem to be valuable, and provide the opportunity for insight, albeit not through the traditional, gold-standard mechanism of a typical randomized control trial (RCT), with its distinct, highly specified methods of data collection and analysis.
This presents a dilemma. On the one hand, as Saria asserts, “our reliance on RCTs alone for evidence generation is dramatically slowing down the rate at which we can learn from our data.”
Rubin’s response (effectively representing the broader medical establishment) is measured. He agrees both that RCTs are “the gold standard right now,” and that they “have tremendous limitations…right now, you can really only ask one question, and it can take 10 years and $100 million to get the answer to that question.” He notes that data from EHRs — “real world data” — is “fundamentally different, and it’s a work in progress to figure out how to make that rigorous,” adding “we have to figure out how to bring rigor, and how to understand the rigor within trials that are not traditionally designed.” Emerging disciplines, Rubin said, need to think about “what do they consider as rigorous,” and to develop “reasonable standards” that can be used for evaluation.
The challenge of leveraging EHR data for evidence generation was experienced directly by Microsoft’s Lee, in the context of his work with the Mayo Clinic on their effort to evaluate convalescent plasma for COVID-19 therapy. This effort enrolled over 100,000 patients, treated over 71,000, and resulted (famously or infamously, depending on your point of view) in the FDA granting Emergency Use Authorization for this treatment.
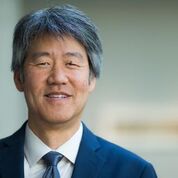
Peter Lee, Corporate Vice President,
Microsoft Research & Incubations
As Lee candidly describes it;
“For the vast majority of those 71,000 patients, there was tremendous access to clinical histories in electronic form. And so it was almost a perfect situation in modern era where we ought to be able to take all of that clinical experience in a compressed time frame and extract information about safety and efficacy of that experimental therapy…but the process was extremely difficult. And in fact, ultimately, the data was pretty impoverished. And so the amount of instrumentation that we need, the amount of forethought in clinical practice so that the digital exhaust of what we can learn from that clinical practice really feeds into advancing science and ultimately regulatory approvals. Altogether, this is still, in my mind, absolutely the future, but is just much more difficult and subtle than at least I had realized, even as short as one year ago.”
While the spirit to learn from EHR is willing, the flesh (or at least the requite infrastructure), it seems, remains weak.
Hurdles and Barriers
Perhaps predictably, two impediments to the productive application of AI to medicine emerged from the discussion: data sharing and implementation.
The challenge of data access, long lamented, remains a serious problem – perhaps the most significant problem, according to a clearly exasperated Rudin – in bringing AI to medicine.
Rudin notes:
“The thing that’s stopping a huge amount of scientific research in health care and AI is lack of data. It’s not an AI question, but if we could solve it, it would give a lot of AI answers…you can’t even reproduce a lot of the scientific papers from a few years ago. Sometimes you e-mail the authors, like the lead author of the paper, and they say, well, I never actually had access to that data in the first place. That was done somewhere else by somebody else. Then you email that person and they don’t have the data. It’s impossible to get it. So how are you going to estimate the effect of drugs? How are you going to, you know, reproduce any scientific study and do a better job of it using machine learning if you don’t have access to the data?”
While acknowledging the “tradeoff with privacy,” Rudin says that “if we cannot figure out ways to make data available for scientists to use, then AI is just going to continue to not be used in hospitals, that’s all I can say.”
Asked if the culture, perhaps, is starting to shift, Rudin is blunt: “No, it’s not.”
Even COVID-19, it seems, couldn’t motivate the necessary change.
Rudin expected that when the virus hit:
“We would be getting e-mails from everywhere saying, hey, we’ve got a bunch of data on COVID patients, here you go — [a dataset that has] a whole medical record for everybody with the COVID information and their survival and all this stuff. No. There’s a few databases that supposedly are available. But the truth is, they’re not. There’s a lot of barriers to even to get into those databases.”
The gap between the many high-profile, data sharing consortia that have sprung up with great fanfare in response to the pandemic, and the apparent difficultly experienced by a top academic computer scientist trying to use these data, seems disappointing (though I might add: hardly surprising).
Microsoft’s Lee also lamented the challenge of accessing the data needed during the COVID-19 crisis. Working with hospitals in Seattle and elsewhere, Lee says, “we saw this really completely, vividly.”
As Lee tells it, in the early days of COVID-19, it was “critically important” for hospitals and hospital systems “to understand what patients are being seen, what COVID-19 encounters were taking place, what capacity do we have to treat those patients properly? And that capacity is hospital beds, ICUs [intensive care units], PPE [personal protective equipment], testing and so on. And then how is that capacity being utilized?”
Yet, “despite the incredible digitization over the past decade and fifteen years in all manner of health care operations, what we found was we still had frustrating inability to sort of connect the digital dots here,” Lee says. “We had things like PPE tracked in digital ERP [enterprise resource planning] systems, we had encounters uncoded, but in free form text in EHR systems. And we had very little understanding of utilization.”
Even worse, explains Lee, “in terms of fundamental data interoperability standards, we couldn’t quite connect rapidly the identities of people across these various digital silos.”
While Lee insists he’s “optimistic about the future, because the world, and particularly the US, is moving rapidly to address these interoperability issues,” he emphasizes that the crisis forced many to “confront firsthand…some of the work that we still have to do.”
Beyond the (palpably traumatic) interoperability challenges several speakers highlighted, an additional difficulty apparent in the translation of AI into practice concerns.
In particular, both Saria and Ehlert emphasize that just because a problem is either “interesting” or “solvable,” and might represent an attractive academic research project, that “doesn’t mean it’s actually interesting to be solved in regular practice and regular life,” as Ehlert put it. He cited the example of a startup that “had put an enormous amount of energy” into developing a device that would use an automated system to instantly measure your height as you walked through the door. “I didn’t realize we had a height problem in health care,” he quips.
Observes Saria “in academia, we think a lot about publishing papers that show new models and evaluating performance of models. But when you start turning into practice,” you need to start “really thinking through all of the uses cases and thinking about harms versus benefit analysis.”
Related more broadly to implementation, Ehlert also highlighted what may be the most significant challenge of all: alignment. The issue, he points out, isn’t for-profit vs not-for-profit institutions. “The reality,” he astutely observes, “is everybody has a stakeholder.” He continues, “a hospital does well when there’s more admissions. A physician does well when they treat more patients. A pharmaceutical does well when they sell more pills. An insurance company does well when they manage the risk better.”
Noting the many different business models these different organizations have, it’s perhaps not surprising that, as Ehlert suggests, “One of the biggest issues I think that we all struggle with is how do we get alignment across those things.”
Perhaps, Ehlert says, we need to “look at our fellow humans” and agree “that our real goal” is for “people to have a better health outcome, and have the highest quality of life for the maximum number of years possible.”
If we’re all aiming for that shared goal, adds Ehlert hopefully, we should be able to “actually align people” to ensure that the “data is collected the way that it needs to be collected to actually make that happen.”
He’s right, of course. But it’s a big “if” – and a big “should.”
Note: some quotes have been very lightly edited for clarity.