Grand Défi Ou Goulot D’étranglement Ultime: A French Pharma Tackles Data Science
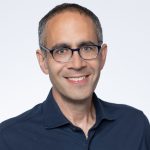
David Shaywitz
Most biopharma companies have started down the path of digital transformation – a fundamental overhaul of everything they do for the digital age.
It’s not clear yet that anyone has arrived at the desired destination.
Even so, there have been some early wins, generally related to operations, as the CEOs of both Novartis and Lilly have described. Arguably, the most significant R&D success has been the organizational alignment and focus afforded by accurate, up-to-date digital dashboards, reflecting, for example, the status of the COVID clinical trials that Pfizer was running, as discussed here.
Behind the scenes, many biopharma R&D organizations have been exceptionally busy trying to apply emerging digital and data technologies to improve every aspect of how impactful new medicines are discovered, developed, and delivered. This strategic focus – and my current job description – is an industry preoccupation.
R&D represents such a vast opportunity space for emerging digital and data technologies that it can be difficult to keep track of all the activity across this expansive frontier. But a recent podcast delivers. A January 2023 episode of BIOS hosted by Chas Pulido and Chris Ghadban of Alix Ventures, and Brian Fiske of Mythic Therapeutics features Sanofi’s CSO and head of research, Frank Nestle. He provides a comprehensive, fairly representative introduction into the many ways biopharmas are approaching digital and data. He also shares insights into key underlying challenges (spoiler alert: data wrangling).
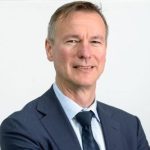
Frank Nestle, chief scientific officer, Sanofi
Nestle is a physician-scientist and immunologist by training; he has been with Sanofi since 2016, and in his current role for about two years.
Below, I discuss the key points Nestle makes about digital and data across R&D, and then offer additional perspective on these opportunities and challenges.
Vision: The Great Convergence
Nestle envisions that we’re heading towards a “great convergence between life sciences, engineering, and data science.” He adds that the “classical scientific foundations of physics, chemistry, and biology” have each “had their heyday.” Now, he says, “it’s data sciences and A.I.”
AI/ML Impact on Research: Optimizing the Assembly Line
Early drug development, Nestle argues, can be understood as an assembly line, where a new molecule is designed and then serially optimized. At each stage, “we are optimizing drug-like properties, like absorption, biodistribution in the body,” he explains. Historically, decisions along the way were made by people – often with extensive experience — sitting around a table and reviewing the data. Now, Nestle is trying to collect the rich data associated with each step in a more systematic way, so that A.I. can contribute.
At the moment, Nestle says, the focus is on using data science to optimize each individual step, but allows that eventually, a “grand model” might be possible.
Nestle notes that both early focus and early successes involve small molecules. For example, the number of potential molecules that must be synthesized and evaluated in the course of making a potential small molecule drug has been reduced, he said, from 5,000 to “several hundred.” He asserts Sanofi remains interested in applying A.I. to the optimization of biologics. The challenge is “complexity.” Nevertheless, he suggests that using A.I.-based approaches to optimize biologics holds “at least as much promise, if not more promise, than in the small molecule space.”
To accelerate their efforts in the early drug discovery and design space, Nestle says, Sanofi has partnered with a number of tech bio companies including Exscientia, Atomwise, and Insilico Medicine.
Translational Research: Organizing Multimodal Data
The process of understanding the molecular basis of disease, Nestle says, begins with “molecule disease maps.” The best description of these maps that I found was actually from ChatGPT (a technology I recently discussed), which reports that the term:
“refers to a visual representation or diagram of the molecular interactions and processes that are associated with a particular disease. This map can include information about the genes, proteins, and signaling pathways involved in the disease, as well as how these elements interact with each other. The goal of creating a molecular disease map is to gain a better understanding of the underlying causes of a disease and to identify potential targets for therapeutic intervention. By mapping the molecular interactions involved in a disease, researchers can gain insights into the complex biological mechanisms that drive disease progression and develop more effective treatments.”
According to Nestle, “these molecular disease maps are becoming more complex and more rich in data sets by the day.” He continues, “It started with mainly genetic datasets, but now we have expression data sets at every single level from RNA to proteins to metabolites. And we look at these molecular disease maps actually at a single-cell level.”
The upshot, he says, is that these “provide us with an incredible space of data to interrogate.”
The algorithms used to analyze these data are often simple cluster analyses, Nestle says, but the goal is always to “reduce dimensionality” and find a “signal in these sometimes very noisy datasets.”
These molecular disease maps not only inform biomarker identification, but also assist with the identification of patient populations who might be particularly well (or poorly) suited for specific medications.
Also contributing to the translational work, according to Nestle: a partnership with the French-American company Owkin. He cites their expertise in both federated machine learning (focused on clinical data associated with various medical centers) and digital pathology.
Emerging Trial Technologies and Patient-Centricity
Nestle describes digital biomarkers as “absolutely ready for prime time.” He cites the use of actigraphy (see this helpful explainer from Koneksa) in the assessment of Parkinson’s Disease as a promising example in an area – neurology – where such biomarkers are critically needed because “trials just take too long.” He also mentions an approach under development, pioneered by MIT professor Dina Katabi, that repurposes a typical wireless router to monitor activities such as itching and scratching in some skin conditions.
Sanofi, like all biopharmas, is interested in decentralized trials; Nestle highlights a partnership (initiated pre-pandemic) with the company Science37. He also sees a “clear future not only for (the use of technology) in patient recruitment but also remote patient monitoring.” The adoption of technology to enhance trial recruitment and patient monitoring, he says, has been accelerated, dramatically and irreversibly, by the pandemic.
Nestle also emphasizes the role and importance of real world data (RWD) as a tool to better understand patients and their journeys. Insights from RWD can be used to improve “study feasibility or sample size optimization or endpoint modeling,” he says, and points to a “journey mapper” Sanofi has used to integrate and interpret RWD. This approach helped identify additional indications for Sanofi and Regeneron’s IL-4/IL-13 inhibitor dupilumab (Dupixent). That work has translated into benefits for a broad range of patients — and more revenue for the company.
Finally, Nestle highlights internal work on “integrated platform data solutions.” That sounds like efforts focused on supporting a drug after it’s launched through the provision of enabling technologies connecting patients, physicians, and data.
Limitations: Analyse Sexy, Données Difficiles
Perhaps Nestle’s most important comments concern the limitations of advancing A.I. and other emerging technologies. And the most difficult challenge – “the ultimate bottleneck,” he asserts –is “the data.”
He continues:
“Right now, data often exists in silos, they’re fraught with missing values – those zeros, as we call them — they’re not labeled correctly, they’re difficult to find. And that’s probably one of the biggest hurdles … that whole effort of generating, aggregating, normalizing, processing the data sometimes outweighs the actual analysis effort.”
He points out that “building foundations” – required for thoughtful data management – “is not necessarily a KPI [key performance indicator]” for large pharmas (who tend to be more focused on near-term measurements of performance). Hence you can only accomplish this, he says, with strong strategic support from “very senior leaders.” (This support may prove both more elusive and more essential in the context of Sanofi’s disappointing 2023 outlook – see here.)
A second problem Nestle points out are the well-intentioned country-specific regulations governing data protection. These policies tend to be quite fragmented across nations and healthcare systems. That impedes the flow of data, and complicates opportunities to learn from the aggregated experiences of patients. The federated approach used by Owkin represents one approach to managing some of these challenges.
Additional Reflections
Nestle offers a comprehensive and generally upbeat assessment of the opportunities before us in the application of emerging digital and data technology to R&D.
Additional opportunities I’m particularly excited about include imagining what may be possible (more accurately, anticipating what seems likely to be possible) through current and future large language models like GPT-3 and (soon?) GPT-4. One example: effortlessly matching clinical trial protocols to the patient populations already present at certain medical centers.
There are additional significant challenges that are easy to lose sight of, particularly in the context of such a compelling vision. For instance, I’m impressed by the challenge of timely digital biomarker development and validation. In a regulatory environment where teams struggle to validate electronic administration of well-established paper scales, the challenge of validating wearable parameters is often substantial, and doing this at the same time you’re developing the molecule you hope to use the digital tool to assess is exceptionally, often prohibitively ambitious. The many levels of complexity, and relatively constricted timelines, can be overwhelming.
On the clinical trial front, the universal embrace of decentralized trials – an obviously patient-centric concept that is endorsed and pursued by most everyone – belies the many challenges in pulling these off at all, to say nothing of doing them efficiently and reliably, and using available technology platforms (PR promises aside). The complexity and expense of actually executing meaningfully decentralized trials (versus, for example, conducting a single check-in remotely and calling it a win) is the elephant in the room (one of them) that isn’t discussed in polite company, but which preoccupies many of us who believe deeply in the concept of decentralized clinical trials and are eager to see the promise fulfilled.
Also not to be underestimated: the challenge of organizing multimodal data on a platform where the data can be accessed and analyzed. I appreciate the value of giving names (like “digital molecular map” or “journey map”) to important problems and significant data missions. Ultimately, though, success depends on the quality of the underlying data and the utility of the platform on which these data are housed and analyzed. This is arguably yet another area where vendor slideware seems far ahead of actual user experience.
Some of the most significant challenges digital and data efforts face within R&D are (remain) organizational. Traditional drug developers – those in the trenches, doing the work – are still trying to figure out what to make of data science and data scientists in what is still a largely traditional drug development world. This challenge is compounded by a massive gap between the hype of technology and contemporary reality.
On the other hand, when there is compelling, readily implementable technology, it’s enthusiastically adopted. Every structural biology group, for instance, routinely uses AlphaFold, the program that predicts protein folding (structure) based on underlying amino acid sequence data.
An area of real opportunity (and acknowledging my own bias) is translational medicine, where practitioners struggle to parse actionable insights from a motley collection of multi-modal data. (The need is particularly great given that the industry’s most costly problem — see here — is the absence of good translational models.) The concept of integrating diverse data to generate biological and clinical insights is universally celebrated, of course, but the data wrangling challenges are exceptional. Perhaps because of this, translational medicine, arguably, still hasn’t quite lived up to its potential. As a discipline, it offers particular promise to thoughtful data scientists, with profound opportunity for outsized impact.
Bottom Line
R&D organizations recognize that emerging digital and data technologies represent important, perhaps essential, enabling tools to advance their mission. As Sanofi’s Frank Nestle explains, digital and data technologies are increasingly deployed across a range of R&D activities. So far, our reach far exceeds our grasp, but there’s been real progress, along with a high probability of more success. Our greatest challenges are navigating not the sexy analytics and data visualizations that everyone covets, but rather, the far less glamorous work of establishing the underlying data flows upon which everything depends. This is a lesson familiar to accomplished data scientists like Recursion’s Imran Haque and Verily’s Amy Abernethy, among others – see here.
The data challenges are amplified in large biopharmas because these companies:
- operate internationally, necessitating adherence to a wide array of data restrictions;
- are the result of decades of mergers and acquisitions, further complicating data management;
- inevitably involve complex organizational politics that must be navigated, as Stanford’s Jeffrey Pfeffer has compellingly described.
Data science continues to hold extraordinary promise for biopharma; translational medicine represents a particularly compelling opportunity. Our collective challenge is figuring out how to work through the considerable data wrangling challenges and deliver palpable progress.