Hot Topics in Biopharma: Initial Impact of Digital, Data Dilemmas in Clinical Studies, and the Search for ‘New Normal’
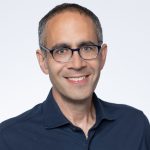
David ShaywitzFor today: topics relevant to many drug developers (and others):
For today: topics relevant to many drug developers (and others):
- The initial impact of digital
- The dilemma of data collection in early clinical studies
- The elusive search for “new normal” ways of working
Initial impact of digital in biopharma
The sexy promise of digital/data/AI in biopharma was that emerging digital technologies were going to solve our most important and vexing problems. At the most breathless moments, some asked whether IBM’s Watson would cure cancer.
AI, generally speaking, has continued to struggle in areas where the underlying data are a mess and/or where it’s difficult to aggregate adequate amounts of reasonably reliable data. One example where such insight is needed but not currently available: translational models.
As I discussed in a recent column, drug developers desperately need improved translational models, improved abilities to predict which candidate molecules are safe and effective. This would increase the success rate of large and costly Phase 2 and Phase 3 trials. While AI has proved remarkably helpful in domains such as predicting protein folding, it’s currently difficult to imagine that in the near future, AI will be able to anticipate reliably what will happen — good and bad — when a molecule is introduced into the human body.
On the other hand – as I described in 2021 — emerging digital technologies are having an immediate impact in the “digital transformation” of how pharma functions, similar to the way other businesses have been transformed. The new direction tends to emphasize large platforms, repeatable processes, and real-time dashboards that enable teams, as well as the broader organization, to align around shared data. In short: business operations, including R&D operations.
(I’ve previously emphasized the value of dashboards as a valuable management tool for Pfizer in the development of their COVID-19 vaccine; separately, I have also discussed the downside of excessive reliance on dashboards and metrics.)
The emphasis on operations in pharma closely parallels areas of near-term progress in healthcare, where much of the initial effort, and low-hanging fruit, seem to be in what physician and venture capitalist Vineeta Agarwala calls “the workflow and administration category,” and highlights the intensive activity she’s seen in the “revenue cycle management and billing world.” (The comments are from a recent podcast, featuring Agarwala, fellow VC Vijay Pande, and physician and digital champion Eric Topol; the episode, here, is highly recommended.)
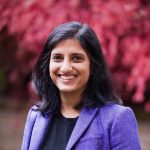
Vineeta Agarwala, general partner, Andreesen Horowitz
Why operations? For one, these data tend to be plentiful, easily obtainable, and readily analyzable. For another, these data are consequential in a fashion business leaders intuitively understand, particularly when focused on parameters like efficiency.
A more interesting question is whether these efforts will ultimately enable higher-level inquiry, or will just enable drugs to fail slightly faster and cheaper. The optimistic view – which I favor — is that eventually the process of digitization will enable the thoughtful secondary analysis of large volumes of laboratory and clinical data. That sort of analysis could catalyze the development of novel and effective medicines. In the short term, though, most of the impact is likely to be on more prosaic measures of process efficiency.
One final point: a key aspect of the digital vision for biopharma is to maximize the opportunity for the organization to learn from every byte of data collected. This mirrors the much-discussed and often elusive (see here) vision for a learning healthcare system, where the goal is for each patient to benefit from the knowledge gleaned from each previous patient and aggregated for all previous institutional experience. (Dr. Agarwala nicely discusses this aspiration in a recent McKinsey interview, here.)
Historically, pharma companies have struggled to comprehensively capture and leverage the totality of their data and experience, as both data and expertise tend to reside in hard-to-locate organizational silos. The digitization of the enterprise offers the hope that more wisdom will be shared, allowing more insights to emerge.
Dilemma of data collection in early clinical studies
As much as drug developers try to understand a new molecule in pre-clinical studies and models, the introduction of a compound into a human being for the first time represents a profoundly important event, and contributes to a step-change increase in our understanding of the molecule.
The initial (Phase 1) clinical studies are focused on ensuring the new molecule is safe and well-tolerated; the product is given to healthy subjects (Phase 1a) and volunteer patients (Phase 1b) in carefully escalating doses in a controlled and monitored research environment.
Phase 2 studies, generally somewhat larger, are focused on starting to figure out whether the new medicine is likely to be acting the way you hoped in the body – does it seem like it might be working on treating the disease?
Broadly speaking, there are two schools of thought regarding how to approach early phase studies. Many researchers see these trials as an opportunity (some would say obligation) to learn as much about the medicine and the human body as possible. New molecules, they point out, are ultimately “perturbogens,” designed to poke certain parts of certain cells in certain ways. By analyzing in as comprehensive a way as possible how the body responds, there is the opportunity for extensive knowledge capture and insight generation. Thus, some scientists want to include in early phase studies a number of additional assays and evaluations.
The other school of thought – generally adopted by the folks responsible for the conduct and operation of clinical studies – emphasizes the value of parsimony.
They argue clinical studies should be (and must be) as simple as possible, with the minimal number of tests required to achieve the trial’s primary objective. Reducing complexity, they point out, increases both study recruitment (a simpler study with fewer evaluations is generally more convenient and less burdensome for subjects) and study conduct, since there are fewer ways for things to go wrong. The much-lauded RECOVERY study in COVID is frequently cited as highlighting the value of parsimony in trial design.
The truth is that it’s a very difficult balance to strike, and if (like me) you’ve been around long enough, you’ve lived through the ongoing struggles as well as the consequences. I’ve seen clinical teams lamenting the omission of data elements that they subsequently wished they had, but I’ve also seen clinical study protocols (often drafted by recent academic emigrees) that are incredibly clever and refined and complex – and then prove to be impossible to recruit and execute.
Clearly, an elaborately designed scientific study with zero subjects isn’t particularly useful – the phrase “succès d’estime” springs to mind. At the same time, an extremely simple study that fails to capture valuable data may represent a critical missed opportunity to advance the science and accelerate therapeutic development for the benefit of patients.
My increasing concern here is that just as we’re gaining the ability to leverage and contextualize increasing amounts of multimodal data, especially critical for translational science, drug development organizations find themselves under enormous pressure to make studies as lean as possible. Short-term performance metrics (the vital signs intensively scrutinized by most companies) are relentlessly focused on efficiency – on how fast and cheaply a study is conducted.
The consequences of this emphasis – the missed opportunity to capture meaningful insight – is far more difficult to measure, and is appreciated (if at all) only far later in development.
To summarize, the short-term corporate incentives to drive efficiency tend to be more palpable than the incentives to resist them in favor of more extensive data collection. Presumably, if or when the value of richer data collection – and the pragmatic utility of and necessity for translational research and data – becomes more readily apparent, the balance might change, and measures viewed as optional today might become essential in the future.
Elusive search for “new normal” ways of working
Finally, a few thoughts on the “new normal.” Despite the efforts of most corporate leaders across most sectors of the economy to return employees to the office, many are still choosing hybrid work, and are coming to the office only sporadically.
The surprise, perhaps, is just how effective groups comprised of many hybrid workers seem to be. Teams are functioning, the work is getting done, and the employees are on balance happy with the arrangement — even if management continues to grumble.
I was talking about this recently with a technology colleague, a long-time pharma industry veteran who only recently joined our company, and had actually started working remotely even before the pandemic.
My colleague emphasized – correctly, I think – how much everyone has learned about remote work during the pandemic. The combination of improved tools and extensive experience has enabled many employees to become phenomenally effective. Particularly when combined with occasional, high-value in-person team experiences, hybrid work seems like a promising alternative for many employees that seems here to stay.
Full disclosure — I remain a bit old-school here. As much as I dislike the commute and dread the Cambridge traffic each day, I still come to the office 4-5 days a week. I like running into people unexpectedly and serendipitously, and I appreciate the sense of place – however empty the place may often be.
When not driving my kids to and from school — moments I cherish — I use my time in the car to listen to podcasts and audiobooks, catch up with friends and family, and follow-up with colleagues at work. But could I use the hours I spend in bumper-to-bumper traffic more productively outside the confines of my automobile? I suspect the answer is yes.