How The Unmet Needs of Patients Made Me A (Grounded) BioTechno-Optimist
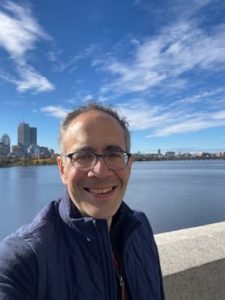
David Shaywitz on the Longfellow Bridge
My Ground Truth
Every other week, I stroll across the Longfellow Bridge from Cambridge to Boston. It can be a magnificent walk in the fall and spring, when the weather is temperate and the skies clear. You can see the deep blue of the Charles River, the Esplanade on your left, with the Boston skyline behind it, and the Citgo sign in the distance.
On the other side, you see the biotechnology cluster of Kendall Square and a large swath of MIT, expanding out from the river’s northern shore. Boston University’s campus lies a bit further upstream, on the south, while Harvard’s campus is a few bridges further down, to the north.
For me, the regular walk to Boston provides me with critical grounding – partly because I’ve spent most of my adult life within the vista before me, but mostly because of the hour-long teaching conference at Massachusetts General Hospital (MGH) I attend, learning about the complicated challenges faced by a patient receiving care on internal medicine service. The discussion is often inspirational and is invariably humbling.
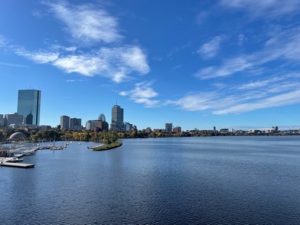
The Charles River, as seen from the Longfellow Bridge
The conference, and the activities that support and enable it, were originally conceived by two MGH medicine residents, Dr. Lauren Zeitels and Dr. Victor Fedorov. In 2016, they founded the Pathways program, “dedicated time during residency for house staff to connect with scientists and delve into the fascinating biological questions that arise at the bedside.” Particularly in a busy hospital like MGH, with many acutely ill patients requiring focused attention, such time to reflect can be fleeting, yet remains vital, as Dr. Denny Ausiello and I discussed here.
Tragically, Drs. Zeitels and Fedorov died in 2017, in an avalanche while snowshoeing in Canada. The pathway program endures as a legacy to the power and urgency of their vision.
Pathways conferences are led by talented medicine residents who spend two weeks focused intently on the biological questions underlying a patient’s illness, with the hope of identifying key scientific questions that, if answered, could point the way to improved diagnosis and treatment. Like most clinical cases discussions, the conference presentation is oriented around the patient, starting with a review of how the patient came to be in the hospital, and generally ending with a discussion of the patient’s hospital course and anticipated trajectory. In between these essential bookends, there’s invariably an intriguing discussion around the often-mysterious biology underlying a patient’s illness.
By design, the team focuses on complex or confusing cases – at times, it feels like it might be called the “idiopathic conference” since many of the diagnoses featured tend to fall into this category (“idiopathic” is the fancy medical term for “we don’t understand what’s going on”).
Unfailingly, these deep dives into biology expose a central truth that has long impressed me about medicine: how little we still understand about how the body works, about what causes many diseases, and how limited are our arsenal of tools to diagnose and effectively, precisely treat many ailments. I felt this profoundly when I was a medical student seeing patients on the wards at MGH, and again when I was an internal medicine resident and later endocrinology fellow at MGH. I continue to experience these exact emotions today.
When you look at the examples of progress in medicine and biology, the list is impressive. The modalities available to diagnose and treat disease continues to expand, while the terrible diseases that we’ve learned to prevent (like polio) or more effectively manage (like cystic fibrosis) speak uncontestably to the promise of science.
Yet when you take your measure by your ability (or lack of ability) to diagnosis and treat the patient in front of you – much less prevent the disease from occurring in the first place – you are quickly overwhelmed by profound humility.
But you also feel something else. Not only do you leave these conferences with a deep appreciation for the limitations of our existing knowledge, you invariably emerge with a palpable sense of urgency, a determination that we must do better, and drive harder to ensure that nascent technologies, both biological and digital, are brought to bear, relentlessly as well as thoughtfully, in service of patients.
Level-setting expectations for emerging tech
If the MGH Pathway conferences regularly remind me of how urgently improved diagnostics and therapeutics are required, recent events have reminded us how difficult the journey will be.
Several recent examples stand out:
Precision Medicine
Consider this question from Andreessen-Horowitz general partner and Bio+Health lead Vijay Pande, during his podcast interview of Olga Troyanskaya, a computer scientist and geneticist at Princeton.
With remarkable candor, Pande asks,
“There was so much excitement about precision medicine with genomics, right? I think the idea was that, okay, we could sequence a patient and maybe sequence a tumor and from that we would be able to do so much. We’d be able to figure out which cancer drug to give or which drug in general to give. And that hasn’t quite come to fruition yet, it feels like — or maybe it has happened so gradually that we’ve lost track. What’s your take on precision medicine?”
The idea that precision medicine hasn’t quite lived up to the admittedly extravagant expectations is a familiar perspective on the wards and in lab, but to hear it from within the reliably exultant investment community feels like real progress.
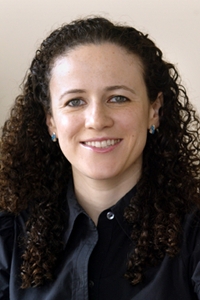
Olga Troyanskya, professor, Princeton University
Troyanskaya’s response offers similar candor. While pointing out that “we have seen a lot of successes,” she also recognizes that “a lot of it was hype,” adding “None of us, I think, quite appreciated quite how complex it is. We sort of thought we’ll find these few genes, they’re the drivers, we’ll develop targeted therapies, we’re done.”
To be sure, she points out, there are “true revolutionary successes of precision medicine.” She cites our increasingly sophisticated approach to breast cancer and some subtypes of lung cancer as two examples.
But, Troyanskaya adds, “What I think we underestimated is that it’s not enough to actually just know the genome, that it’s not, you know, it’s not just a few causal genes.” We also need to understand the non-coding 98% of the genome, she says, and to capture other types of data, including proteomics and metabolomics.
AI for Drug Discovery
Coming up with impactful new medicines is remarkably difficult, and the idea that AI can improve the odds offers obvious appeal.
But at least some of the bloom is off the rose, as it’s now clear that many of the so-called “AI discovered” drugs have not made it through development.
“The first AI-designed drugs have ended with disappointment,” writes Andrew Dunn in Endpoints.
He continues,
“Over the last year-plus, the first handful of molecules created by artificial intelligence have failed trials or been deprioritized. The AI companies behind these drugs brought them into the clinic full of fanfare about a new age of drug discovery — and have quietly shelved them after learning old lessons about how hard pharmaceutical R&D can be.”
He also points out that while the last few years have been notoriously challenging for small biotechnology companies (the XBI biotechnology stock index has dropped by 50% over the last two years), it’s been even worse for companies focused on AI drug discovery, dropping by 75-90% over the same time.
[Chart courtesy of A. Dunn, Endpoints News]
(It’s also been a challenging time for digital health, see this report from Rock Health, and for healthtech – see this report from Bessemer Venture Partners. Interestingly, investor focus in these areas seems to be shifting from the aspiration of radically disrupting healthcare and biopharma to the appreciably more modest goal of making existing processes, especially non-clinical or back-office, somewhat more efficient.)
As many commentators have pointed out, the obsession with “AI drugs” is a little silly, in that AI is a tool that may inform a complicated, multi-step process. Moreover, a range of computational techniques are often involved in the development of medicines. Thus, the idea that one product is an “AI drug” and another isn’t feels more than a little arbitrary.
There’s also the issue of expectations – even if AI is able to contribute to the efficiency of early activities in drug discovery, at best this might only improve the overall process slightly given the multiple downstream hurdles that remain (especially since it’s not clear, as we’ve discussed, that AI can offer particularly useful predictions around late phase clinical studies, where most of the time and dollars are spent).
VC Patrick Malone (cited by Dunn) may be exactly right when he says,
“If you take the hype and PR at face value over the last 10 years, you would think [the probability of creating a successful drug] goes from 5% to 90%. But if you know how these models work, it goes from 5% to maybe 6% or 7%.”
In a separate post on LinkedIn, Malone (citing Leonard Wossnig, Chief Technology Officer at LabGenius) riffs on a key underappreciated challenge in drug discovery, particularly drug discovery that involves algorithms: figuring out what to optimize for – the so-called “objective function.”
For instance, you might try to identify a compound that binds incredibly tightly to a specific receptor, say. Yet it turns out that in many cases, such an approach isn’t quite right – empirically, you may discover you need a molecule that binds with moderate affinity to multiple receptors. (I’ve discussed this nuance in the context of phenotypic screening and the discovery of olanzapine, a medicine for schizophrenia, for example; see also this captivating recent commentary about phenotypic drug discovery, penned by researchers at Pfizer).
Generative AI in the Enterprise
Generative AI captured our collective attention with the November 2022 release of ChatGPT, as this column has frequently discussed.
Despite the insistence of consultants that if your company is not already leveraging generative AI, it’s behind, I’ve not seen many examples of generative AI in actual use at large companies, particularly biopharmas.
It turns out, this perception is well-founded. According to Andreessen-Horowitz co-founder Ben Horowitz – a great champion of AI – “We haven’t seen anybody [involved in generative AI] with any traction in the enterprise.” (“Enterprise” in this context refers to the information technology systems and processes used by large companies, like pharmas.)
According to the technologist Horowitz was interviewing — Ali Ghodsi, co-founder and CEO of the big data analytics company, Databricks – there are at least four reasons generative AI hasn’t yet entered the enterprise:
- Big companies invariably move slowly and cautiously.
- Corporations are terrified that they will lose control of high-value proprietary data.
- Companies typically need output that is exactly right, not sort of right.
- There is often a “food fight” (as Ghodsi puts it) at companies about which division controls generative AI.
From what I’ve seen across our industry to date, I can only say that Ghodsi seems to deeply understand his enterprise customer base.
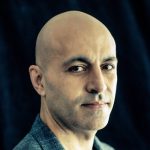
Ali Ghodsi, co-founder and CEO, DataBricks
I was also struck by how Ghodsi – whose business embraces and enables generative AI – readily acknowledged the limitations of this technology. “It’s stupid and it makes mistakes,” Ghodsi says, adding “it quickly becomes clear that you need a human in the loop. You need to augment it with human. Look, there’s no way you can just let this thing loose right now.” (The need for a human in the loop was also a key assertion Harvard’s Zak Kohane made in his recently-published book about ChatGPT and medicine – see here.)
Ghodsi was also skeptical about performance benchmarks cited for generative AI – including around their supposedly high scores on medical examinations. He suggested that the models might have picked up the test questions during the course of their training (training that’s shrouded in mystery, as Harvard’s Kohane has pointed out), and thus, their high scores may be deceptive — like an exam where you got the answers the night before.
To be sure, Ghodsi remains excited about the potential of generative AI; he’s just realistic about its present limitations.
III – BioTechno-Optimism?
The talk of tech these days is the latest manifesto penned by Andreessen-Horowitz co-founder, Marc Andreessen, this time on “Techno-Optimism.” In case you missed it, his simplified thesis is that technology=good, and anyone who would constrain it=bad.
The piece has triggered an energetic and largely critical response from writers like Ezra Klein in the New York Times (“buzzy, bizarre”), Steven Levy in Wired (“an over-the-top declaration of humanity’s destiny as a tech-empowered super species—Ayn Rand resurrected as a Substack author”), science fiction author Ted Chiang (“it’s mostly nonsense”), and Jemima Kelly of the Financial Times (“is the billionaire bitcoin-backing venture capitalist OK?”).
Levy’s analysis was perhaps the most resonant:
“[Andreessen] posits that technology is the key driver of human wealth and happiness. I have no problem with that. In fact, I too am a techno-optimist—or at least I was before I read this essay, which attaches toxic baggage to the term. It’s pretty darn obvious that things like air-conditioning, the internet, rocket ships, and electric light are safely in the ‘win’ column. As we enter the age of AI, I’m on the side that thinks that the benefits are well worth pursuing, even if it requires vigilance to ensure that the consequences won’t be disastrous.”
But if I’m being honest, I thought there was something visceral in Andreessen’s screed – or perhaps more accurately, in the impulse behind it — that really touched a chord for me, emphasizing both the value emerging technology can bring and the incredible challenge of nurturing new technologies through their growing pains (what Carlota Perez might describe as the Installation phase), to the point where the demonstrated value is unassailable (the Deployment phase).
Not only is it intrinsically difficult to figure out how to implement new technology effectively, necessitating all sorts of incremental innovations (see here), but there’s exceptional resistance all along the way (at least outside of public relations, where new technology is championed relentlessly).
Incumbents – particularly in healthcare — resist technology for years before eventually (perhaps) absorbing it. In part this reflects inertia – we’re most comfortable with what we already know.
Another factor, as I’ve written, and as Andreessen observes (with his usual bluster), involves the precautionary principle. Stakeholders (in my view) are appropriately concerned about doing harm with novel technologies, but routinely overlook the harm that’s done by inhibiting the adoption of promising new approaches.
Moreover, new technologies often bring significant uncertainties, as Dr. Paul Offit has nicely described in You Bet Your Life (my WSJ review here). This is true not only for new medical treatments, as Offit nicely discusses, but also in how high-stakes research is conducted.
Consider a typical example from the world of clinical trials, the lifeblood of biopharma and academic clinical researchers. Imagine that you hear about a new tech-enabled approach for a key aspect of this process — patient recruitment, say, or decentralized data collection, an approach which is claimed to work better than traditional methods.
If you are leading a clinical development team in a pharma company, where everyone in the business is already breathing down your neck with advice and suggestions and telling you that you’re already behind and reminding you how important it is to be successful, the last thing on earth you want is to take on more risk.
If you use established procedures, at least you know what you’re getting into. But if you try a new approach (even an approach perhaps piloted under far more forgiving conditions), you could get yourself in deep trouble. This actually happens. Not surprisingly, faced with these sorts of decisions, many rational actors within pharma prefer to be “fast followers” rather than adventurous pioneers, a mindset Safi Bahcall has described in Loonshots (see my WSJ review here, and this additional, biotech-focused discussion in Forbes, here).
Concern about assuming new risk (beyond intrinsic risk of new molecules) is also why Gregg Meyers, the Chief Digital and Technology Officer at Bristol-Myers Squibb, says “this is an industry that will not adopt something unless it is really 10x better than the way things are historically done.” See also this generally accurate perspective on the challenges of selling innovative tech into pharma, written from perspective of a startup that’s developing the technology.
(Bio)Technology: Critical Enabler of Medical Progress
Ultimately, for me, it comes back to the patients we discuss every two weeks at MGH. We need far better diagnostics, far better therapeutics, and we need to understand health and disease at a far more sophisticated level.
Historically, improved tools – the microscope, ultrasound, MRI, DNA sequencing – have radically redefined the way we understand and approach disease. Today, emerging modalities and tools — like antibody-drug conjugates (which are enjoying a banner year), cell and gene therapy (enduring real growing pains but with demonstrated successes and tantalizing possibilities), spatial biology techniques (quite exciting), and of course artificial intelligence (which is getting more capable by the second) offer exceptional promise, even if in many cases we’re just beginning to contemplate how to leverage these new technologies effectively. Safety and ethics – appropriately — remain paramount consideration as well; see my recent discussion of Ziad Obermeyer’s important research revealing hidden bias in medical algorithms, and my WSJ review of Brian Christian’s essential The Alignment Problem, here.
While I disagree with Andreessen’s denunciation of skeptics and naysayers, I feel his frustration, and share his belief that technology (guided, I would add, by inquisitive researchers, practitioners, and other “lead users” who are passionate, thoughtful, and deeply empathetic) offers the most promising path forward in our drive to improve the human condition in the area of health and medicine.
Sometimes, it seems, we get so caught up in our clever criticism and overwrought angst that we get in our own way, stall our own momentum, and impede the progress upon which the health of our patients, and of our future patients, will so critically depend.