Lessons For Biopharma from a Healthcare AI Pioneer
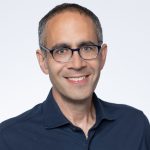
David Shaywitz
As drug developers consider how to leverage AI and other emerging digital and data technologies, they look to related businesses, such as healthcare systems, for lessons and learning.
We would be hard-pressed to find a better guide to AI in healthcare than Ziad Obermeyer, an emergency room physician and health science researcher at the University of California-Berkeley. His research focuses on decision-making in medicine and on the equitable use of AI in healthcare.
Obermeyer was recently interviewed on the NEJM-AI podcast by co-hosts Andrew Beam and Raj Manrai (both faculty in the Department of Biomedical Information at Harvard). The entire conversation — reflective, nuanced, and chock full of insights — should be required listening for anyone interested in potential applications of AI in biopharma. The most relevant highlights for biotech are summarized below.
AI in Medicine: The Opportunity
Obermeyer didn’t set out to become a doctor. He initially studied history and philosophy, then did a stint as a management consultant before eventually applying to med school. He hit his stride once he began his residency in emergency medicine.
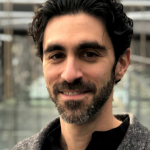
Ziad Obermayer, associate professor and Blue Cross of California Distinguished Professor at the Berkeley School of Public Health
“I really, really liked being a doctor,” he says, “and I think there’s something about that exposure to the real world and the problems of patients that I think it’s shaped the problems that I work on in my research as well.”
Obermeyer began to see medicine as “a series of prediction problems,” and saw artificial intelligence (specifically, machine learning) as a tool that could help make doctors better by assisting them with challenges like establishing a diagnosis, assessing risk, or providing an accurate prognosis.
If you walk into a doctor’s office today, he readily acknowledges, you’re not overwhelmed by a sense of futuristic technology as you fill out paperwork and listen to the fax machine hum.
However, he notes, AI is already widely used in medicine – it’s just operating at the back end, behind the scenes. “On the population health management side, on a lot of other operational sides, like clinic bookings, things that have a direct impact on health, these tools are already in very, very wide use.”
Medicine today is still quite artisanal, guided by rules of thumb and local traditions, Obermayer says. Much of the problem, he suggests, is that it’s hard for doctors to wrap their heads around the volume and variety of healthcare data, which are “high-dimensional” and “super complicated.” To make the best possible predictions given the number of variables, he argues, requires assistance through approaches such as machine learning.
The question isn’t how we think about AI plus medicine; rather, he says, “that is medicine. That is the thing that medicine will be as a science.” This perspective is shared by others in the field including Harvard’s Zak Kohane, who often asserts “medicine is at its core an information- and knowledge-processing discipline,” and progress requires “tools and methods in data science.”
Casting his eye towards the future of AI in medicine, Obermeyer can envision both bear and bull scenarios.
His fear is that AI tools, in addition to harboring biases (see below), will be used for “local optimization of a system that sucks and that isn’t proactive, that’s very oriented towards billing and coding.” He can envision “a very unappealing path where we just get a hyper-optimized version of our current [suboptimal] system.”
”There is a certain lack of ambition in how people are applying AI today,” he said.
More hopefully, he can imagine a future where AI helps solves some of healthcare’s most vexing problems. One opportunity area he sees are addressing conspicuous “misallocation of resources” – essentially, improving our ability to provide the right treatment for the right patient at the right time.
For example, Obermeyer points out that many patients die of sudden cardiac arrest, while at the same time, the majority of defibrillators implanted to prevent sudden cardiac deaths are never triggered. It would be far better medicine, he observes, if more defibrillators were implanted in the patients who would ultimately need them.
He also envisions how AI might enable new discoveries around the pathophysiology of disease by linking biological understanding, biomarkers, and outcomes. A squiggle on an ECG isn’t just an image that an AI can recognize, like a cat on the internet. “We actually know a lot about how the heart produces the ECG,” he explains. “We know what part of the heart leads to what part of the wave form. We have simulation models of the heart that we can get to produce waveforms.”
Consequently, he views the idea of “tying together that pipeline of biological understanding of the heart and how the heart generates data,” and connecting data about patient outcomes is “super-promising,” and suggests it may eventually lead to new drug discoveries. “There are a lot of things you can do once you get the data talking to the biology,” he says.
AI in Medicine: Tactical Considerations
The exceptional promise of applying AI in medicine seemed to be matched only by the challenge of implementing it.
Obermeyer described hurdles in four key areas: data, talent, bias, execution.
Data. AI depends on data as its foundation. This can be a particular problem in healthcare Obermeyer says, noting that “getting the data that you need to do your research is a huge, huge preoccupation of any researcher in this area.” The problem, he continues, “is that the data are essentially locked up inside of the health systems that produced the data. And it can be really perverse… it’s Byzantine and it’s very frustrating, and I think it’s really holding back this space.”
Obermayer established an open-science platform (Nightingale) to make it easier for researchers to get access to datasets from healthcare systems. One example: the team digitized breast cancer biopsy slides that “were literally collecting dust on a shelf in the basement” of a hospital and linked these data to EHR information and cancer registry data.
Getting started wasn’t easy. He approached 200 healthcare systems, he said, and only five agreed to participate: several large non-academic health systems and a few small county hospital systems.
Obermeyer has also set up a for-profit company, Dandelion Health, that aspires to serve as a trusted data broker to make it easier for healthcare AI tool developers to think about their creative applications, rather than spending too much time wrestling to get access to the data in the first place. “There are so many insights and products that could directly benefit patients that are not getting developed today because it’s so hard to access those data,” he says.
Talent. Obermeyer sees healthcare systems as operating at a disadvantage in the digital and data world. “Hospitals can’t hire all the computer scientists that they would need” to do the necessary data science,” he says, “and they’re not going to win the war for talent against Google or Facebook or even just computer science departments of different universities.”
Obermeyer also doesn’t feel that it’s feasible to pair a healthcare expert and an AI expert; he believes it far better to have a “single brain,” even though he acknowledges this “seems ridiculously inefficient” because of the time and effort required to gain this kind of medical and data science expertise. (See here for a contrasting perspective from Dr. Amy Abernethy, championing the collaboration approach.)
The good news, though, is that Obermeyer shares the optimism of venture capitalist Bill Gurley that there’s a huge amount of useful, free information available online, and motivated individuals can find a lot of the training they need; this seems to be how Obermeyer himself became proficient in artificial intelligence.
Obermeyer suggests two conceptual paths for healthcare experts interested in mastering AI. One, he says, starts with statistics, since he (somewhat controversially) regards AI as “an applied version of statistics with real datasets.” In his view, there’s “no substitute for learning the basic statistical stuff. And I think as a starting point, that is an amazing place to start to get a handle on thinking about how AI works, where to apply it, where it can go wrong”
The second route into AI, Obermeyer says, and the one he took, begins with the microeconomics “toolkit,” which he argues was designed “for dealing with data that’s produced by humans and is messy and error prone and driven by incentives. That seems a lot like medicine to me.”
Obermeyer sees the ultimate goal of data science training as learning how to formulate problems effectively – “how to take an abstract question and then think about what is the data frame that would answer this question.”
Obermeyer also points to how helpful AI itself can be to trainees. ChatGPT is particularly helpful in writing code, he says, and approvingly cites AI expert Andrej Karpathy’s quip, “The hottest new programming language is English.”
Obermeyer sees the ultimate goal of data science training as learning how to formulate problems effectively – “how to take an abstract question and then think about what is the data frame that would answer this question.”
Bias. Obermeyer’s research is focused on bias and AI; he seeks to root out hidden bias, as well as to use AI to reduce bias.
Obermeyer is especially well known for a 2019 Science paper that identified an unexpected bias in a population health algorithm. The tool he studied looked at health data from a population and tried to predict which patients were most likely to get sick in the upcoming year, so they could receive extra attention, pre-emptively, and thus stay healthier.
When Obermeyer’s team looked at how this worked in practice, the team found that Black patients predicted to be at same health risk as White patients were far more likely to get sick.
As the authors explain, “The bias arises because the algorithm predicts health care costs rather than illness, but unequal access to care means that we spend less money caring for Black patients than for White patients.” In other words, by using health care costs as a proxy for health care needs – a common assumption of convenience — the algorithm developers inadvertently overlooked, and ultimately propagated, an underlying bias.
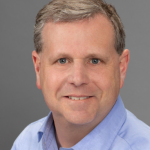
David Cutler, Otto Eckstein Professor of Applied Economics, Harvard University
Obermeyer has also explored how AI might be used to reduce healthcare disparities. He explains that the project was inspired by a talk from a friend and colleague, Harvard healthcare economist David Cutler, on the consistent observation that “black patients have more pain than white patients…even when you control for severity of disease.”
For example, if you consider patients with equally severe knee arthritis, based on standard X-ray scoring, Black patients on average will report more pain than White patients. Cutler attributed this gap, Obermeyer says, to “stuff that’s going on outside knee” – psychosocial stressors, for instance. But Obermeyer thought the issue was something in the knee, and together they decided to study the problem.
Obermeyer’s team trained a deep learning algorithm to predict patient’s pain level – rather than a radiologist’s arthritis score – from the X-rays. “This approach,” the authors report, “dramatically reduces unexplained racial disparities in pain.”
According to Obermeyer, “the algorithm is doing a much, much better job of explaining pain overall, but it’s doing a particularly good job of explaining the particular pain that radiologists miss and that black patients report, but that can be traced back to some pixels in the knee.”
Execution. Motivated by both his sense of purpose and innate curiosity, Obermeyer was clearly frustrated by the slow pace of some of the research in academia, in contrast to an urgency he noticed from colleagues from industry.
“One of the things that I’ve really come to appreciate about the private sector and basically my new non-academic friends and acquaintances,” he said, “is, boy, do they get [stuff] done.”
“They don’t have projects like I have that have gone on for eight years. If it goes on for eight days, it’s like, what’s going on? What’s taking so long? So there’s an impatience and a raw competence that I’ve been trying to learn from that world.”
Bottom Line and Implications for Biopharma
Obermeyer’s experience can’t help but resonate with drug developers. Ours, too, is a business focused on “a series of prediction problems.” Our work tends to leverage digital and data technology far less than many other industries, yet (as I’ve discussed) there are pockets (such as in manufacturing and supply chain management) where there is a remarkable level of sophistication. There would seem to be a profound opportunity for drug developers to make better use of multi-dimensional data. There is already a strong focus on applying emerging technology to improve near-term efficiencies, and the earnest hope these technologies also can be used to identify and elucidate profound scientific opportunities to improve human health. Access to high quality data remains a crippling problem for industry data scientists focused on R&D. Great talent is always in demand, and upskilling employees is an industry priority, while figuring out how to integrate most effectively talented drug developers with skilled data scientists remains a work in progress. Bias is, of course, an area of exceptional concern and focus, and the notion of using technology to promote equity is particularly appealing. Finally, the ability of industry to execute when inspired reminds us of what we can achieve, while the relatively limited impact of AI and data science in R&D across the industry to date, particularly when contrasted with the outsized potential, reminds us of how far we still have to go.
Recent Astounding HealthTech columns on Gen AI
- Generative AI: No Humbug
- Welcome to the AI Irruption
- New Book Contemplates How Generative AI Will Serve Man
- Tech, Pharma, and the Uneven Distribution of the AI-Enabled Future
- Big, If True: Opportunities and Obstacles Facing AI
- Pharma R&D Execs Offer Extravagant Expectations for AI But Few Proof Points
- Learning From History How To Think About the Technology of the Moment