Get In-depth Biotech Coverage with Timmerman Report.
23
Jul
2020
Pfizer Secures $2B for COVID-19 Vaccine, BioNTech Raises $500M, & Our Test
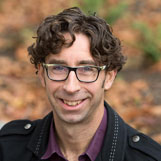
Luke Timmerman, founder & editor, Timmerman Report
When Donald Trump first considered a run for president in 2000, he never rose above 1 percent in the polls.
America changed in the early years of the 21st century.
Trump was always good at harnessing tabloid media weaknesses to his advantage. When our information commons shifted to a 24/7 advertising-based attention-grab economy on the Internet and TV, he was uniquely positioned to seize the moment.
Through feeding the beast with an endless stream of outrage and conflict and provocation and distracting infotainment, he put himself in a position to drive cultural and political conversation in America. By dominating the attention economy, he ended up winning the Presidency in November 2016.
Here’s what we know has happened as of July 23, 2020.
- More than 4 million people in the US have been infected with COVID-19.
- More than 144,000 Americans have died from the infection
- More than 1,000 people in the US are dying each day
- Other countries with serious leaders are performing better. On July 23, the US recorded 1,067 deaths; Germany had 5; Italy, 10; France, 10; the UK, 53. (Source: Worldometers)
- About 32 million Americans are collecting unemployment checks, and new claims are rising
- The US still has no national testing, tracing, and isolation strategy
- The US has no national plan to safely re-open schools this fall
- About 29 million rental households are at risk of eviction
This is a test.
We have a chance to correct this mistake by voting in overwhelming numbers this fall.
If we fail this test, we can expect long-term erosion of US leadership in biopharma. Driving away legal immigrants, 25 percent proposed budget cuts to the NIH, and crude drug price controls are just a few of the anti-biotech policies that this Administration and its enablers supported in office.
Entrepreneurs around the world see an opportunity to poach US biotech talent. See the increasingly bold appeals by ex-US companies, like the one below.
I appreciate our friends in Canada and around the world. But this is up to us in America to chart a different course.
This Week in Drug Pricing
When our information commons is algorithmically transmogrified into a psychological warfare battle zone – not a marketplace for ideas – then it stands to follow that you can’t have a reasoned debate over serious topics like what the price ought to be for a COVID-19 vaccine.
The infodemic, and our nation’s resulting dalliance with a cynical entertainer-in-chief, is the main reason why the US has failed – and continues to fail — to respond properly to the COVID-19 challenge.
Science
- Research in the Context of the Pandemic. NEJM. July 17. ( Clifford Lane and Anthony Fauci)
- The Infectious Nature of Patient-Generated SARS-CoV-2 Aerosol. MedRxiv. July 21. (Joshua Santarpia et al)
- Safety and Immunogenicity of the ChAdOx1 vaccine candidate. A Preliminary Phase I/II Report. The Lancet. July 20. (The Oxford Vaccine Group) (See press release summary)
- Rapid Decay of Anti-SARS-CoV-2 Antibodies in Persons with Mild COVID-19. NEJM. July 21. (Javier Ibarrando et al)
- Anti-SARS-CoV-2 IgG From Severely Ill COVID-19 Patients Promotes Macrophage Hyper-Inflammatory Responses. BioRxiv. July 13. (Willianne Hoepel et al)
- Impact of Delays on Effectiveness of Contact Tracing for COVID-19. A Modelling Study. The Lancet. July 16. (Mirjam Kretzchmar et al)
Vaccines
- Why Those Most at Risk of COVID-19 Are Least Likely to Respond to a Vaccine. NatGeo. July 17. (Roxanne Khamsi)
- Pfizer Gets $1.95 Billion US Contract to Produce 100 million COVID-19 Vaccine Doses by Year End. NYT. July 22. (Noah Weiland et al) (Press release).
Treatments
- Dexamethasone in Hospitalized Patients with COVID-19. A Preliminary Report. NEJM. July 17. (The RECOVERY Collaborative Group)
- Inhaled Interferon Beta Shows Promise for COVID-19, But Scientists Urge Caution. NYT. July 20. (Benjamin Mueller)
- Just-Evotec Secures $18M US Defense Contract to Make Monoclonal Antibodies Against SARS-CoV-2. (Company statement)
Timmerman Report Articles
- When Raising Drug Prices Helps Patients. July 23. (Chris Morrison & Peter Kolchinsky)
- Adaptive Trials: Pioneered in the USA, Implemented in the UK. July 22. (Uciane Scarlett)
- Fidelity Invests $55M in Seer for Scalable Proteomic Technology. July 22. (KJ Mulam)
- Abe Ceesay on The Long Run podcast. July 21. (Luke Timmerman)
- Barring Foreign Talent is an Assault on Biotech. July 20. (John Maraganore & Jeremy Levin)
- Clinical Trials: A High-Value Attack Surface for Entrepreneurs. July 20. (David Shaywitz)
- Karuna Therapeutics Innovates With Schizophrenia Treatment. July 20. (Annie Iserson)
Financings
Boston-based Longwood Fund closed its fifth fund with $170 million to put to work into biotech companies, largely around company creation.
Burlingame, Calif.-based ALX Oncology raised $185.7 million in an IPO at $19 a share. The company is working on drugs that block the CD47 checkpoint pathway.
Emeryville, Calif.-based Berkeley Lights, a digital cell biology company, raised $204.9 million in an IPO at $22 a share.
Watertown, Mass.-based Pandion Therapeutics, a developer of treatments for autoimmune diseases, raised $135 million in an IPO at $18 a share. (See TR coverage of the company’s Series A financing, January 2018).
London and Raleigh, NC-based Verona Pharma, the developer of treatments for respiratory diseases, raised $200 million in a private placement.
Columbus, Ohio-based Forge Biologics raised $40 million in a Series A financing led by Perceptive Xontogeny Venture Fund. The company is seeking to manufacture viral vector gene therapies for other companies on contract, and to develop its own novel therapies.
New York-based Elevation Oncology raised a $32.5 million Series A financing, led by Aisling Capital. The company is going after genomically defined cancers.
Boston-based Cerevance, a company working on brain diseases, tacked on an additional $20 million to close its Series B financing at $65 million.
San Francisco-based Olema Oncology raised $54 million in a Series B financing to advance its work against breast cancer.
Germany-based BioNTech, the mRNA vaccine and therapeutics company working on a COVID-19 vaccine in partnership with Pfizer, raised $511.5 million in a stock offering at $93 a share.
South San Francisco-based Cytokinetics, the developer of treatments for muscle diseases, raised $201 million in a public offering.
Kiniksa Pharmaceuticals raised $155 million in a stock offering. It says it’s based in Bermuda, which we all know is a place where companies go so they can pay their fair share of taxes to support the education and research that makes for a thriving biotech hub.
Personnel File
- Biogen hired Mike McDonnell as chief financial officer.
- Amy Miles, the former CEO of Regal Entertainment, joined the board of directors at Amgen.
- Boston-based Gamida Cell hired Michele Korfin as chief operating and chief commercial officer.
- Woburn, Mass.-based Replimune, the developer of oncolytic virus therapies for cancer, hired Andrea Pirzkall as chief medical officer.
- South San Francisco-based Twist Bioscience, the DNA synthesis company, hired Erin Smith as senior vice president of government affairs and public policy.
- Toronto-based Notch Therapeutics hired David Main as CEO. It’s working on universally compatible T-cell therapies.
- San Francisco-based Parker Institute for Cancer Immunotherapy hired John Connolly as chief scientific officer.
- Cambridge, Mass.-based Generation Bio, the developer of non-viral vector gene therapies, hired Matthew Norkunas as chief financial officer.
Racial Equity
- Investing in Racial Diversity. A Call to Action in the Biotech VC Community. Nature Biotechnology. July 22. (Jackie Grant)
- Leave a Ladder. LifeSciVC. July 20. (Sam Truex)
Data That Mattered
Wilmington, DE-based Incyte said its JAK inhibitor ruxolitinib (Jakafi) met the primary endpoint and key secondary endpoints in a Phase III trial of patients with Graft-vs.-Host disease. The results support findings from two previous studies.
New Haven, Conn.-based BioXcel Therapeutics passed a pair of Phase III clinical trials with its sublingual film formulation of dexmedetomidine. The first study was in schizophrenia patients, and the second was for bipolar disorder. The drug appeared to work fast (within two hours), it worked at both a low and high dose, and had a clean safety profile.
San Diego-based Amplyx Pharmaceuticals said in a topline press release that it passed a Phase II clinical trial of a first-line treatment for invasive fungal infections with Candida.
Deals
GSK stepped up its game in the mRNA vaccine and therapies arena by partnering with Germany-based CureVac. The big drugmaker paid 120 million Euros upfront in cash, and another 150 million Euros in equity investment to work on as many as five mRNA vaccine and therapeutic candidates against infectious diseases.
Cambridge, Mass.-based EQRx in-licensed a CDK4/6 inhibitor from G1 Therapeutics, to develop a low-cost challenger in that therapeutic category of cancer treatment.
South San Francisco-based Assembly Biosciences struck a deal with BeiGene, which obtained China rights to Assembly’s three drug candidates for hepatitis B virus. Assembly is getting $40 million cash upfront.
Foster City, Calif.-based Gilead Sciences paid $300 million for a 49.9 percent ownership stake, and the exclusive right to acquire South San Francisco-based Tizona Therapeutics, the developer of a novel checkpoint inhibitor against cancer.
France-based Genfit said it will stop investing further in its lead drug candidate for NASH, and will release a new corporate strategy by the end of September.